August 21, 2018 feature
A new map management process for visual localization in outdoor environments
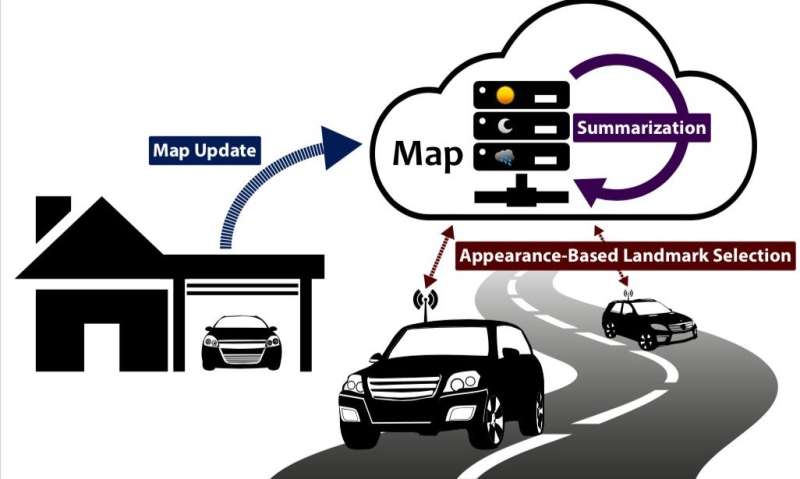
Researchers at ETH Zürich's Autonomous Systems Lab have recently developed a map management process for visual localization systems, specifically designed for operations in outdoor environments involving several vehicles. Their study, presented at this year's Intelligent Vehicles Symposium (IV) and available on arXiv, addresses the key challenge of incorporating large amounts of visual localization data into a lifelong visual map, in order to consistently provide effective localization under all appearance conditions.
"Self-localization is pivotal for any kind of mobile robot, including autonomous vehicles," Mathias Bürki, one of the researchers who carried out the study, told Tech Xplore. "While most autonomous research vehicles are equipped with 3D LiDAR sensors, these are still expensive, and their suitability for future mass production is thus questionable. On the other hand, camera sensors are very cheap, and have already made their way into current automotive fleets (e.g. for parking assistant systems). Therefore, we have been investigating the potential of using cameras as a main sensor for precise localization of autonomous vehicles."
One of the primary challenges encountered when developing visual localization systems for outdoor environments is ensuring that these systems cope well with appearance changes. These include both changes occurring in the short-term (e.g. illumination, shadows, etc.) and long term (e.g. seasonal changes, foliage, etc.).
Past research found that maps created for visual localization could theoretically be adapted to work under varying outdoor appearance conditions. Nonetheless, adapting these maps can be very expensive, requiring substantial resources spent on the servers maintaining the maps and on the autonomous vehicles themselves. While there are a number of solutions that could help to reduce costs and address the complexity of this problem, so far, these have only been investigated in isolation.
"The goal of our recent research was to combine different components and approaches that improve scalability, such as offline map summarization, and online appearance-based landmark selection, in order to build a completely scalable and resource efficient localization and mapping system," Bürki explains. "We also wanted to investigate in detail how well this system works in real-world, long-term conditions, how long it takes for the visual maps to converge to a stable state, how well the different components dealing with scalability work together, and whether one interferes with the other in an undesired way."
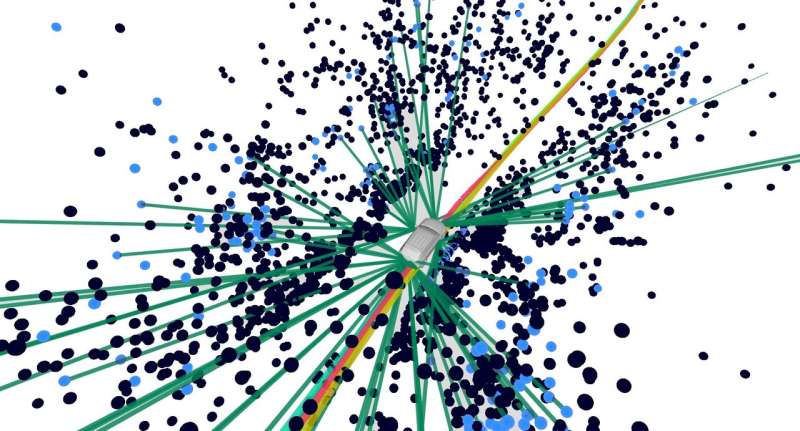
The map management process developed by Bürki and his colleagues works by adding new datasets to the map over time, continuously updating it to better cope with new appearance conditions. Every time a new dataset is added to the map, a subsequent map summarization step ensures that its size does not exceed a certain limit.
"If the new dataset has been recorded under appearance conditions that are already well-covered by the map, the dataset is not added to the map, but statistics about the landmark observations are improved, which in return makes appearance-based landmark selection in future sorties more efficient," Bürki explains.
The researchers tested the new map management process in the real world under challenging outdoor conditions. The results of their evaluations were highly promising, suggesting that their lightweight map management mechanism could help to develop visual localization systems for autonomous vehicles that work well under different appearance conditions, while also performing better at landmark selection.
"Our most meaningful finding was that it is indeed possible and practically feasible to build such a visual localization and mapping system that a) is, and remains efficient, b) is, and remains scalable, and c) delivers accurate localization in outdoor environments in long term," Bürki said. "Another finding was that online appearance-based landmark selection and offline map summarization work well together and complement each other."
In future, most highly-performing autonomous vehicles will most likely be equipped with 3D LiDAR sensors, as these currently appear essential to guarantee safety and ensure that the vehicle effectively perceives obstacles in its surroundings, including pedestrians. Recently, the cost of these sensors has decreased substantially, which could also facilitate their widespread adoption in years to come.
"We will now focus our research more towards the question of how LiDAR sensors can be used to support visual localization," Bürki said. "Especially in poor lighting conditions, cameras unavoidably reach their limits, while LiDARs are well suited also for dark conditions."
More information: Map Management for Efficient Long-Term Visual Localization in Outdoor Environments. arXiv:1808.02658v1 [cs.RO]. arxiv.org/abs/1808.02658
Abstract
We present a complete map management process for a visual localization system designed for multi-vehicle long- term operations in resource constrained outdoor environments. Outdoor visual localization generates large amounts of data that need to be incorporated into a lifelong visual map in order to allow localization at all times and under all appearance conditions. Processing these large quantities of data is non- trivial, as it is subject to limited computational and storage capabilities both on the vehicle and on the mapping backend. We address this problem with a two-fold map update paradigm capable of, either, adding new visual cues to the map, or updating co-observation statistics. The former, in combination with offline map summarization techniques, allows enhancing the appearance coverage of the lifelong map while keeping the map size limited. On the other hand, the latter is able to significantly boost the appearance-based landmark selection for efficient online localization without incurring any additional computational or storage burden. Our evaluation in challenging outdoor conditions shows that our proposed map management process allows building and maintaining maps for precise visual localization over long time spans in a tractable and scalable fashion.
© 2018 Tech Xplore