Chip design with AI inside—designed by AI
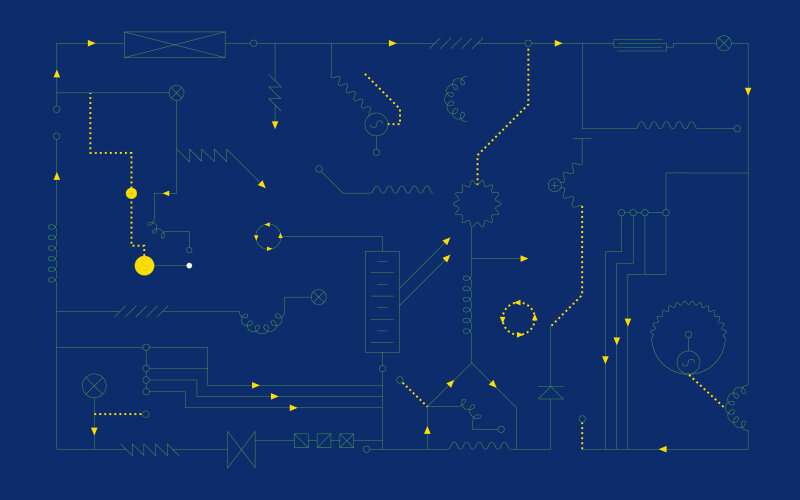
In less than a decade, artificial intelligence (AI) has gone from an obsession of a few ivory tower academics to runaway commercial success, potentially adding around US$13 trillion to the global economy by 2030 according to a McKinsey projection. One reason that AI is taking off now rather than when it was first conceptualized in the late 1950s is the availability of affordable computational power, in turn, made possible by steady advances in chip design.
But for all the technological advances ever smaller and more powerful integrated circuits (IC) have ushered in, designing the chips themselves remains a time-consuming and labor-intensive task. Although electronic design automation (EDA) software automating the placement of transistors on a chip has been available since the 1980s, the input of experienced human engineers is still required in what is largely a trial-and-error process, together with EDA tools to find the optimized sweet spot.
"More specifically, a large number of simulations and verifications are manually performed during the conventional design process. If the specification in any design cycle is not met, the designers have to redesign and verify the performance through simulation again," explained Salahuddin Raju, a Scientist at the Institute of Microelectronics (IME), A*STAR.
"Many EDA companies have joined AI bandwagon, offering specific AI capabilities across different design tools. However, their approach is not flexible enough to include design styles of various chip design houses and does not provide learning together in a cohesive manner with the designers. Moreover, AI-assisted EDA tools are sold at a premium, forcing customers to be locked into contracts with specific EDA vendors and increasing the cost of the chip design," explained Rahul Dutta, a Principal Research Engineer at IME, A*STAR.
But what if AI could be used to design chips instead, irrespective of the underlying EDA tools and design? In a virtuous circle, a team of A*STAR researchers from IME and the Institute for Infocomm Research (I²R) has now developed a machine learning framework which works in tandem with the EDA tools to capture the experience of seasoned chip designers, using it to reduce the cost of designing new chips while simultaneously exploring new design spaces.
The incredible shrinking chip
For the last fifty years, chips have become simultaneously smaller and more powerful in keeping with Moore's law. Moving from the 180nm process to 90nm in the mid-2000s, for example, effectively allowed chip makers to squeeze double the number of transistors on the same chip. Smaller chips mean shorter distances traveled within the chip, resulting in greater speed while shrinking transistor sizes mean less energy consumption. Both these factors combined to make chips cheaper as they got smaller.
But this size-cost relationship has begun to break down. These days, making chips even smaller has become so expensive and complicated that it may no longer make financial sense to keep developing smaller processes. Manufacturing costs aside, it is the design of new chips that takes up a sizeable portion of the total cost, with paying for EDA software estimated to contribute nearly half of the total development cost. Semiconductor consulting firm IBS predicts that shifting from 16nm to 10nm processes increased the cost of chip design by US$174.4 million, while moving even further to 7nm processes would cost nearly US$300 million.
"Furthermore, with the increased circuit complexity in advanced technology nodes, circuit design criteria become more stringent and designers have to go through more iterations to achieve multiple design goals," Raju said. "As a result, productivity suffers, firms incur more cost and it takes more time to bring the product in the market."
Despite the costs involved, IC foundries can ill-afford to compromise on their hardware design. Unlike software that can be shipped in a less-than-perfect state and subsequently patched, defective chips cannot be fixed once produced, potentially costing companies eye-watering sums. A hardware bug in Intel's flagship Pentium chips discovered in 1994 reduced the company's profits by 37 percent, going down in history as one of the costliest mistakes in hardware design.
Less data, more learning
To reduce the cost and time taken to design new chips, the team led by IME's Kevin Chai, Senior Scientist and Head of IC Design, turned to AI, specifically, a subset of machine learning known as semi-supervised learning. In supervised learning, the algorithm is trained using a set of inputs paired with the desired outputs, requiring a large amount of pre-labeled data. In the case of chip design, input features are the design variables of the circuit, such as transistor length, width, bias and temperature, etc., while the outputs are design goals such as power consumption, bandwidth, other performance criteria and chip area.
"When a design specification or desired output is set, the learning model proposes the input parameters for the design. The design is then verified by computation- and time-intensive EDA simulations," Chai said. "To reduce the number of simulations required, we used a semi-supervised learning model that can be trained with a small amount of labeled data and a large pool of unlabeled data."
The resulting AI algorithm and EDA automation, created under the Smart IC Design with Learning Enablement (SMILE) program, reduced the amount of labeled data required by 90 percent compared to supervised learning. As previously mentioned, EDA software has been around for a long time, and great strides have recently been made in the field of machine learning; it was integrating both advances that was the key challenge, Chai said.
"In the whole design iteration process, there is no human designer in the loop. The circuit designer just has to select the circuit topology and design specifications at the initial stage and the rest of the design is performed by the tight integration of EDA tool with the AI framework," Rahul added.
Data-driven chip design
The resulting AI was able to complete a complex design in just one day, whereas a human designer would ordinarily have required one week. Furthermore, when the AI-designed chip was fabricated by a foundry and tested at A*STAR laboratories, its performance was found to be twice that of the best human optimized design. This performance was achieved through balancing chip design trade-offs in speed, area and power.
With these impressive results, the SMILE platform has already attracted interest from players in the semiconductor industry, such as fabless IC design companies, Chai said. However, he notes that the technology is still in the development phase, and will require further validation and generalization to make it compatible with a wide range of circuit topologies before it can be deployed commercially.
Nonetheless, AI is undoubtedly the future of chip design, Chai continued. "SMILE will definitely change the way circuit designers look at design," he said. "Gone are the days where much is dependent on experience and heuristics. Designers of new chips will be greatly aided by 'data-oriented' design strategies, thus greatly reducing the number of simulation iterations, the time taken to reach design targets and the costs of design optimization."