Machine learning model predicts mortality in COVID-19 patients
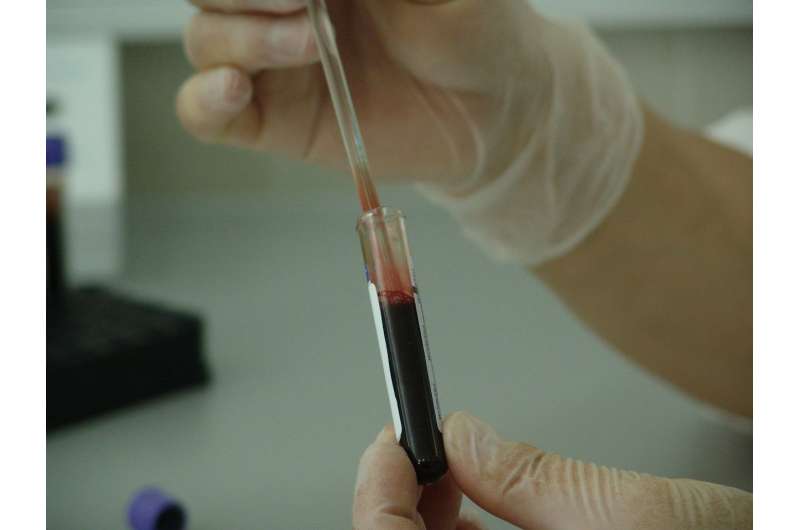
Machine learning tools selected three biomarkers—lactic dehydrogenase, lymphocyte and high-sensitivity C-reactive protein levels—that can predict the mortality of COVID-19 patients from blood samples from 485 infected individuals in Wuhan, China, according to paper published in Nature Machine Intelligence. These tools predicted the mortality of individual patients more than ten days in advance of their outcomes with more than 90% accuracy.
Fast, accurate and early clinical assessment of patients' COVID-19 severity is vital. However, there is no currently available predictive biomarker to distinguish patients that require immediate medical attention and to estimate their associated mortality rate.
Ye Yuan, Li Yan colleagues analysed blood samples of 485 patients from Wuhan, China, to identify robust and meaningful markers of mortality risk. Samples collected between 10 January and 18 February 2020 from patients in Tongji Hospital were used for model development. Of the 375 cases included in the analysis, 201 recovered from COVID-19 and were discharged from the hospital, while the remaining 174 patients died.
The authors designed a mathematical modelling approach based on machine learning algorithms devised to identify the biomarkers most predictive of patient mortality. The problem was formulated as a classification task, where the inputs included basic information, symptoms, blood samples and the results of laboratory tests, including liver function, kidney function, coagulation function, electrolytes and inflammatory factors, taken from general, severe and critical patients. The model selected lactic dehydrogenase (LDH), lymphocyte and high-sensitivity C-reactive protein levels as the most crucial biomarkers distinguishing patients at imminent risk. This finding is consistent with current medical knowledge that high LDH levels alone are associated with tissue breakdown occurring in various diseases, including pulmonary disorders such as pneumonia. Most patients had multiple blood samples taken throughout their stay in the hospital. However, this model only used data from the patients' final sample. Nevertheless, the model can be applied to all other blood samples and the predictive potential of the biomarkers can be estimated.
The authors conclude that their model provides simple, interpretable and intuitive clinical test to precisely and quickly quantify the risk of death. They also suggest that lymphocytes, a type of white blood cell, may serve as a potential therapeutic target, which is supported by clinical studies. They note that, as more data become available, this procedure will need to be repeated for better accuracy.
More information: Li Yan et al. An interpretable mortality prediction model for COVID-19 patients, Nature Machine Intelligence (2020). DOI: 10.1038/s42256-020-0180-7