A novel AI algorithm for digital pathology analysis
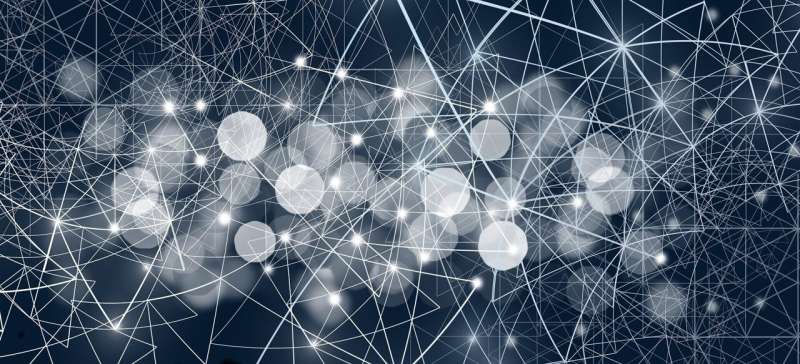
Digital pathology is an emerging field which deals mainly with microscopy images that are derived from patient biopsies. Because of the high resolution, most of these whole slide images (WSI) have a large size, typically exceeding a gigabyte (Gb). Therefore, typical image analysis methods cannot efficiently handle them.
Seeing a need, researchers from Boston University School of Medicine (BUSM) have developed a novel artificial intelligence (AI) algorithm based on a framework called representation learning to classify lung cancer subtype based on lung tissue images from resected tumors.
"We are developing novel AI-based methods that can bring efficiency to assessing digital pathology data. Pathology practice is in the midst of a digital revolution. Computer-based methods are being developed to assist the expert pathologist. Also, in places where there is no expert, such methods and technologies can directly assist diagnosis," explains corresponding author Vijaya B. Kolachalama, Ph.D., FAHA, assistant professor of medicine and computer science at BUSM.
The researchers developed a graph-based vision transformer for digital pathology called Graph Transformer (GTP) that leverages a graph representation of pathology images and the computational efficiency of transformer architectures to perform analysis on the whole slide image.
"Translating the latest advances in computer science to digital pathology is not straightforward and there is a need to build AI methods that can exclusively tackle the problems in digital pathology," explains co-corresponding author Jennifer Beane, Ph.D., associate professor of medicine at BUSM.
Using whole slide images and clinical data from three publicly available national cohorts, they then developed a model that could distinguish between lung adenocarcinoma, lung squamous cell carcinoma, and adjacent non-cancerous tissue. Over a series of studies and sensitivity analyses, they showed that their GTP framework outperforms current state-of-the-art methods used for whole slide image classification.
They believe their machine learning framework has implications beyond digital pathology. "Researchers who are interested in the development of computer vision approaches for other real-world applications can also find our approach to be useful," they added.
These findings appear online in the journal IEEE Transactions on Medical Imaging.
More information: Yi Zheng et al, A graph-transformer for whole slide image classification, IEEE Transactions on Medical Imaging (2022). DOI: 10.1109/TMI.2022.3176598