Designing next generation analog chipsets for AI applications
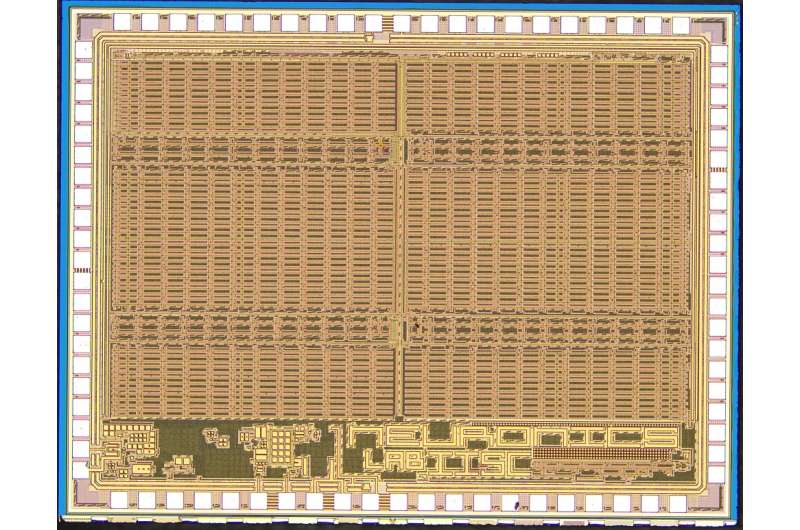
Researchers at the Indian Institute of Science (IISc) have developed a design framework to build next-generation analog computing chipsets that could be faster and require less power than the digital chips found in most electronic devices.
Using their novel design framework, the team has built a prototype of an analog chipset called ARYABHAT-1 (Analog Reconfigurable technologY And Bias-scalable Hardware for AI Tasks). This type of chipset can be especially helpful for Artificial Intelligence (AI)-based applications like object or speech recognition—think Alexa or Siri—or those that require massive parallel computing operations at high speeds.
Most electronic devices, particularly those that involve computing, use digital chips because the design process is simple and scalable. "But the advantage of analog is huge. You will get orders of magnitude improvement in power and size," explains Chetan Singh Thakur, assistant professor at the Department of Electronic Systems Engineering (DESE), IISc, whose lab is leading the efforts to develop the analog chipset. In applications that don't require precise calculations, analog computing has the potential to outperform digital computing as the former is more energy-efficient.
However, there are several technology hurdles to overcome while designing analog chips. Unlike digital chips, testing and co-design of analog processors is difficult. Large-scale digital processors can be easily synthesized by compiling a high-level code, and the same design can be ported across different generations of technology development—say, from a 7 nm chipset to a 3 nm chipset—with minimal modifications.
Because analog chips don't scale easily—they need to be individually customized when transitioning to the next generation technology or to a new application—their design is expensive. Another challenge is that trading off precision and speed with power and area is not easy when it comes to analog design. In digital design, simply adding more components like logic units to the same chip can increase precision, and the power at which they operate can be adjusted without affecting the device performance.
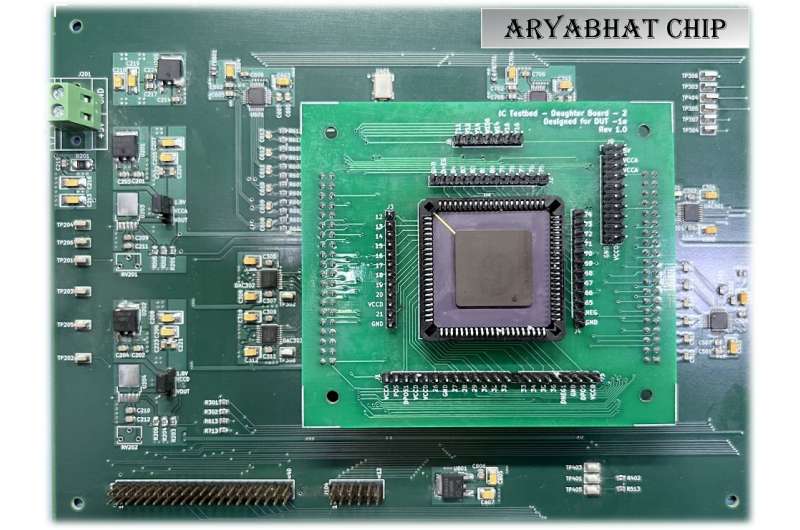
To overcome these challenges, the team has designed a novel framework that allows the development of analog processors which scale just like digital processors. Their chipset can be reconfigured and programmed so that the same analog modules can be ported across different generations of process design and across different applications. "You can synthesize the same kind of chip at either 180 nm or at 7 nm, just like digital design," adds Thakur.
Different machine learning architectures can be programmed on ARYABHAT, and like digital processors, can operate robustly across a wide range of temperatures, the researchers say. They add that the architecture is also "bias-scalable"—its performance remains the same when the operating conditions like voltage or current are modified. This means that the same chipset can be configured for either ultra-energy-efficient Internet of Things (IoT) applications or for high-speed tasks like object detection.
The design framework was developed as part of IISc student Pratik Kumar's Ph.D. work, and in collaboration with Shantanu Chakrabartty, Professor at the McKelvey School of Engineering, Washington University in St Louis (WashU), U.S., who also serves as WashU's McDonnell Academy ambassador to IISc. "It's good to see the theory of analog bias-scalable computing being manifested in reality and for practical applications," says Chakrabartty, who had earlier proposed bias-scalable analog circuits.
The researchers have outlined their findings in two pre-print studies that are currently under peer review. They have also filed patents and are planning to work with industry partners to commercialize the technology.
More information: Kumar P, Nandi A, Chakrabartty S, Thakur CS, Process, Bias and Temperature Scalable CMOS Analog Computing Circuits for Machine Learning, arXiv preprint arXiv:2205.05664 (2022) arxiv.org/abs/2202.05022
Kumar P, Nandi A, Chakrabartty S, Thakur CS, CMOS Circuits for Shape-Based Analog Machine Learning, arXiv:2202.05022 (2022) arxiv.org/abs/2202.05022
Thakur CS, Chakrabartty S, Kumar P, A RECONFIGURABLE AND SCALABLE MULTI-CORE ANALOG COMPUTING CHIP, Provisional IP, Challan: 2511210015847