This article has been reviewed according to Science X's editorial process and policies. Editors have highlighted the following attributes while ensuring the content's credibility:
fact-checked
peer-reviewed publication
trusted source
proofread
Creation of training data to estimate the states of care robot users
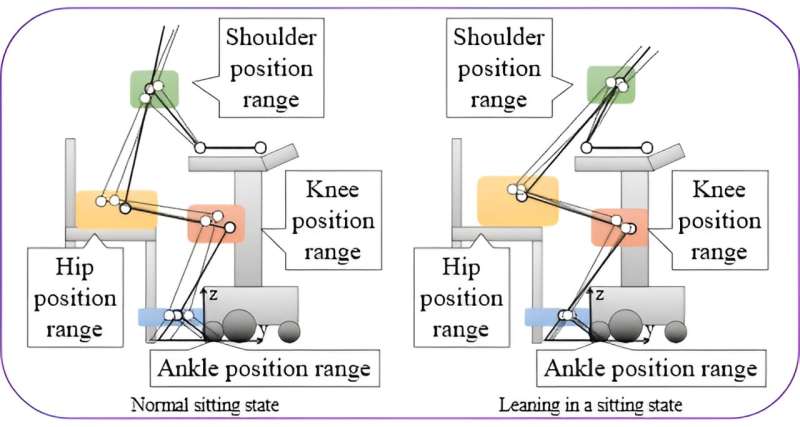
A research team led by Assistant Professor Mizuki Takeda from the Department of Mechanical Engineering, Toyohashi University of Technology, has developed a technique to generate training data for robots that operate based on estimations of the user's state using machine learning. The research is published in the journal IEEE Access.
To date, some methods based on machine learning have been proposed to estimate the state of a robot users using candidate points for the position of the center of gravity. However, for such learning, training data corresponding to when the robot is used to support movements are required. The research team developed a method of creating training data by using a human body link model without the need to analyze the movements.
The development of robots supporting frequently performed tasks such as standing up and walking is progressing as elderly people with weakened muscles often require assistance in their daily lives. Robots often need to automatically conduct assistive actions to reduce the burden on human caregivers. This feature of robots requires estimating the state in terms of the posture of the elderly who use the robot and providing suitable support for their state.
The research team has proposed a method for calculating the candidate positions of the center of gravity of the robot user and estimating the state using machine learning. However, in this method, it is necessary to acquire the user's movements as training data while using the robot. Particularly, acquiring the data in abnormal states (such as postures in which the user is likely to fall while walking) could be strenuous for the elderly being in these abnormal states.
Therefore, the research team developed a method for creating training data for candidate positions of the center of gravity by using a human body link model without the need to measure movements. The human body link model is a simple model representing the human body with rigid links and rotating joints. This model can be used to simulate human positions in each state such as sitting, standing, and abnormal states and thus to create training data.
Experiments have confirmed that the care robots can learn based on the training data created by this method, estimate the user's state, and support standing up, walking, and sitting down actions.
The team believes that the developed training data creation method based on the human body link model can be applied to care robots of various shapes and serving various purposes. Moreover, it can be applied to industrial and communication robots, where operations based on state estimations of persons are required.
In the future, the research team plans to create a system that is safer and easier to use by developing an interference-based robot capable of presenting the necessary information to the user for effective communication between humans and robots.
More information: Mizuki Takeda et al, Training Data Generation Using Human Link Model for State Estimation of Care Robot User, IEEE Access (2023). DOI: 10.1109/ACCESS.2023.3292344