Using machine learning to understand materials
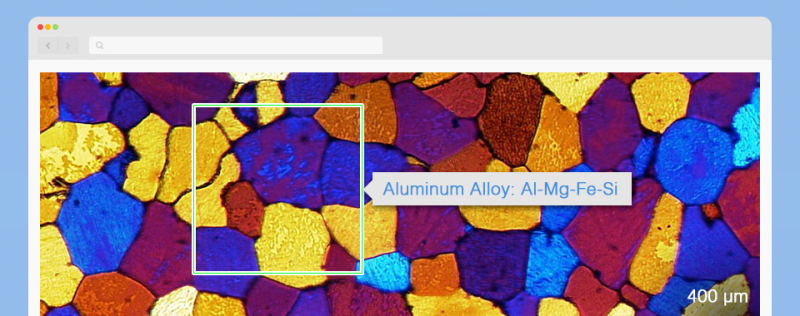
Whether you realize it or not, machine learning is making your online experience more efficient. The technology, designed by computer scientists, is used to better understand, analyze, and categorize data. When you tag your friend on Facebook, clear your spam filter, or click on a suggested YouTube video, you're benefitting from machine learning algorithms.
Machine learning algorithms are designed to improve as they encounter more data, making them a versatile technology for understanding large sets of photos such as those accessible from Google Images. Elizabeth Holm, professor of materials science and engineering at Carnegie Mellon University, is leveraging this technology to better understand the enormous number of research images accumulated in the field of materials science. This unique application is an interdisciplinary approach to machine learning that hasn't been explored before.
"Just like you might search for cute cat pictures on the internet, or Facebook recognizes the faces of your friends, we are creating a system that allows a computer to automatically understand the visual data of materials science," explains Holm.
The field of materials science usually relies on human experts to identify research images by hand. Using machine learning algorithms, Holm and her group have created a system that automatically recognizes and categorizes microstructural images of materials. Her goal is to make it more efficient for materials scientists to search, sort, classify, and identify important information in their visual data.
"In materials science, one of our fundamental data is pictures," explains Holm. "Images contain information that we recognize, even when we find it difficult to quantify numerically."
Holm's machine learning system has several different applications within the materials science field including research, industry, publishing, and academia. For example, the system could be used to create a visual search of a scientific journal archives so that a researcher could find out whether a similar image had ever been published. Similarly, the system can be used to automatically search and categorize image archives in industries or research labs. "Big companies can have archives of 600,000 or more research images. No one wants to look through those, but they want to use that data to better understand their products," explains Holm. "This system has the power to unlock those archives."
Holm and her group have been working on this research for about three years and are continuing to grow the project, especially as it relates to the metal 3-D printing field. For example, they are beginning to compile a database of experimental and simulated metal powder micrographs in order to better understand what types of raw materials are best suited for 3-D printing processes.
Holm published an article about this research in the December 2015 issue of Computational Materials Science titled "A computer vision approach for automated analysis and classification of microstructural image data."
More information: This material is based upon work supported by the National Science Foundation under Grant No. DMR-1507830. Any opinions, findings, and conclusions or recommendations expressed in this material are those of the author(s) and do not necessarily reflect the views of the National Science Foundation.