February 12, 2017 weblog
AI researchers get a sense of how self-interest rules
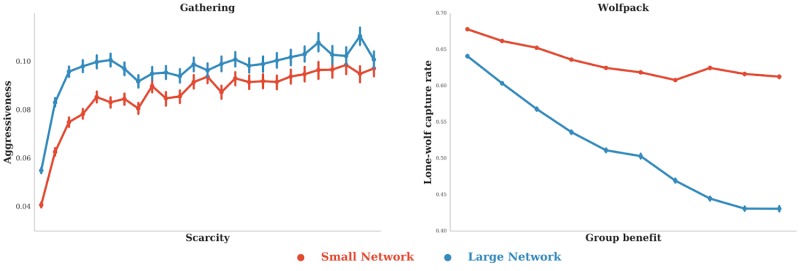
(Tech Xplore)—Interested in serious mind games, of the artificial intelligence variety? Park these phrases in your vocabulary list, courtesy of a DeepMind exploration: "deep multi-agent reinforcement learning" and "sequential social dilemmas."
Those phrases are to bring you closer to what the human thinkers at DeepMind are up to these days.
DeepMind, founded in London, is focused on artificial intelligence research and its application for positive impact.
Researchers there have been looking at how rational agents interact and arrive at cooperative behavior. They also want an understanding of times when agents turn on each other.
What's the significance of their interest? "The research may enable us to better understand and control the behaviour of complex multi-agent systems such as the economy, traffic, and environmental challenges," they said, in the DeepMind blog.
To test their AI agents, DeepMind developed two games, Gathering and Wolfpack. The researchers said in a paper they wrote on the subject that both games under study were implemented in a 2-D gridworld game engine.
The researchers explain that modern deep reinforcement learning methods take the perspective of an agent that must learn to maximize its cumulative long-term reward through trial-and-error interactions with its environment.
They posted videos earlier this month of two games discussed in their paper. Gathering gameplay is one of the games, and this is where two agents, Red and Blue, collect apples (green) and occasionally tag the other agent (yellow lines).
"We let the agents play this game many thousands of times and let them learn how to behave rationally using deep multi-agent reinforcement learning."
They said that when there were enough apples around, the agents learned to peacefully coexist and collect as many apples as they could. Those were in easy times and cooperation came easy. Then what happened? As the number of apples went down, the agents learned it may be better to tag the other agent to give themselves time on their own to collect the scarce apples.
So choices were made, to cooperate and collect apples, or to defect and try to tag the other agent.
The other game, Wolfpack, was where (Red) wolves chased the blue dot while avoiding (gray) obstacles. The researchers blogged that Wolfpack "requires close coordination to successfully cooperate," and here they found that "greater capacity to implement complex strategies leads to more cooperation between agents."
It represented the opposite of the finding with Gathering.
The five research scientists from DeepMind have authored the paper, "Multi-agent Reinforcement Learning in Sequential Social Dilemmas." They are Joel Leibo, Vinicius Zambaldi, Marc Lanctot, Janusz Marecki and Thore Graepel.
In their paper, they said they did three experiments: one for each game (Gathering and Wolfpack), and a third experiment investigating parameters that influence the emergence of cooperation versus defection.
What does their work mean in the real world? Nick Summers in Engadget put their work in perspective.
"The findings are important as humanity releases multiple AI into the world. It's likely some will clash and try to either co-operate or sabotage one another. What happens, for instance, if an AI is managing traffic flow across the city, while another is trying to reduce carbon emissions in the state?"
Summers also said that "Setting parameters, and being mindful of other agents, will be crucial if we're to balance the global economy, public health and climate change."
Parker Wilhelm in TechRadar: "As for what DeepMind can do with this data, researchers posit that their findings closely resemble the theory of Homo economicus - the idea that human nature is, generally speaking, rational yet ultimately self-interested."
More information: DeepMind blog: deepmind.com/blog/understanding-agent-cooperation/
Research paper: storage.googleapis.com/deepmin … -agent-rl-in-ssd.pdf
© 2017 Tech Xplore