May 26, 2017 weblog
AI exploration shifts focus from rewards to curiosity
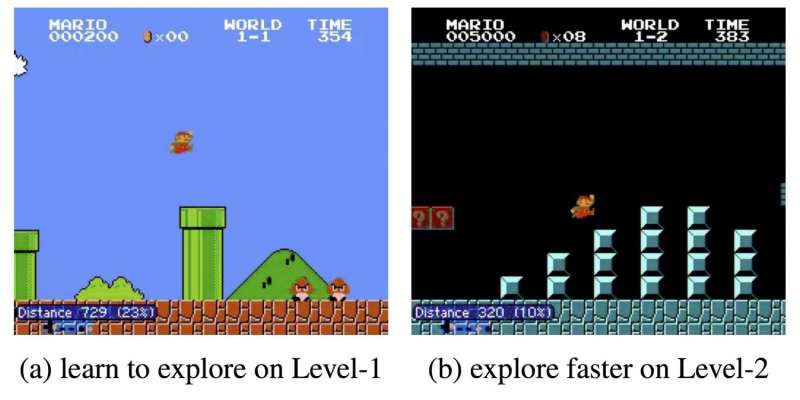
(Tech Xplore)—A paper titled "Curiosity Driven Exploration by Self-Supervised Prediction" has been prepared by a team of researchers at University of California Berkeley (on arXiv).
Do not be intimidated by the title, as the paper discusses their fascinating path into AI, in a swerve from customary reinforcement learning.
A video published earlier this month from co-author Pulkit Agrawal is a summary of their paper for the International Conference on Machine Learning.
They have been teaching computers to be curious. As Futurism put it, "Researchers have successfully given AI a curiosity implant."
But over to as "intrinsic curiosity" model which the authors mentioned in the video. What are they talking about?
Will Knight in MIT Technology Review had this to say. The model was developed by the researchers at the University of California, Berkeley, "to make their learning algorithm work even when there isn't a strong feedback signal."
The authors further explained on GitHub. "Idea is to train agent with intrinsic curiosity-based motivation (ICM) when external rewards from environment are sparse. Surprisingly, you can use ICM even when there are no rewards available from the environment, in which case, agent learns to explore only out of curiosity: 'RL without rewards'."
Futurism said that "This could be the bridge between AI and real world application." Tom Ward said, "Most current AIs are trained using 'Reinforcement Learning'—they are rewarded when they perform a task that helps them to reach a goal or complete a function."
That, said Will Knight, has been considered as a method with benefits, in that it made it possible "for machines to accomplish things that would be difficult to define in code."
At the same time, said Knight, it carried limitations. "Agrawal notes that it often takes a huge amount of training to learn a task."
The authors of the paper are Deepak Pathak, Pulkit Agrawal, Alexei Efros, Trevor Darrell, from the UC Berkeley. They highlight on the video posting the fact that in the real world, rewards are sparse or absent.
"In many real-world scenarios, rewards extrinsic to the agent are extremely sparse, or absent altogether. In such cases, curiosity can serve as an intrinsic reward signal to enable the agent to explore its environment and learn skills that might be useful later in its life."
An agent in their study, for example, learned how to move along corridors without any extrinsic rewards. Their proposed approach was evaluated in two environments: VizDoom and Super Mario Bros.
Results? Knight reported that in both games, "the use of artificial curiosity made the learning process more efficient."
"While the AI that was not equipped with the curiosity 'upgrade' banged into walls repeatedly, the curious AI explored its environment in order to learn more," said Ward in Futurism.
Why does this matter? If they did make the machines curious, will that result in better performance of complex tasks? It will be interesting to watch further work by these researchers. MIT Technology Review said, "The UC Berkeley team is keen to test it on robots that use reinforcement learning to work out how to do things like grasps awkward objects."
The researchers released the demo on the GitHub webpage. It was built upon TensorFlow and OpenAI Gym.
More information: Curiosity-driven Exploration by Self-supervised Prediction, arXiv:1705.05363 [cs.LG] arxiv.org/abs/1705.05363
Abstract
In many real-world scenarios, rewards extrinsic to the agent are extremely sparse, or absent altogether. In such cases, curiosity can serve as an intrinsic reward signal to enable the agent to explore its environment and learn skills that might be useful later in its life. We formulate curiosity as the error in an agent's ability to predict the consequence of its own actions in a visual feature space learned by a self-supervised inverse dynamics model. Our formulation scales to high-dimensional continuous state spaces like images, bypasses the difficulties of directly predicting pixels, and, critically, ignores the aspects of the environment that cannot affect the agent. The proposed approach is evaluated in two environments: VizDoom and Super Mario Bros. Three broad settings are investigated: 1) sparse extrinsic reward, where curiosity allows for far fewer interactions with the environment to reach the goal; 2) exploration with no extrinsic reward, where curiosity pushes the agent to explore more efficiently; and 3) generalization to unseen scenarios (e.g. new levels of the same game) where the knowledge gained from earlier experience helps the agent explore new places much faster than starting from scratch.
© 2017 Tech Xplore