November 3, 2017 weblog
When is a baseball espresso? Neural network tricked and it is no joke
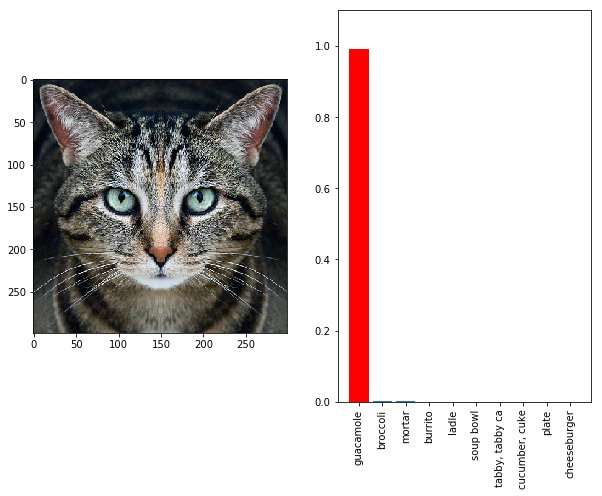
(Tech Xplore)—When you're working on a project where your intended turtle image is taken as a gun— who has been messing around? Turns out a team of researchers have been messing around for serious ends. They found a way to fool neural networks.
They made the networks misbehave in that they fiddled around using an algorithm that helped fool the networks. Their bragging rights:
"We've developed an approach to generate 3D adversarial objects that reliably fool neural networks in the real world, no matter how the objects are looked at." The team is reporting from LabSix—an independent, student-run AI research group composed of MIT undergraduate and graduate students.
Think "adversarial" objects in 3D. When they say "adversarial" they refer to "carefully perturbed inputs" causing misclassification.
Such as? A tabby cat, which they perturbed "to look like a guacamole to the Google's InceptionV3 image classifier."
This was achieved with a new algorithm, they said.
Details about their work are in "Synthesizing Robust Adversarial Examples," which is up on arXiv. The authors are Anish Athalye, Logan Engstrom, Andrew Ilyas and Kevin Kwok.
They said their method for constructing real-world 3D objects consistently fools a neural network across a wide distribution of angles and viewpoints.
In their work, they applied the algorithm to arbitrary physical 3D-printed adversarial objects, "demonstrating that our approach works end-to-end in the real world."
But then again, who would put all their faith in the way AI views the world? Dave Gershgorn in Quartz delivered a sobering reminder of all that a neural network is and is not.
"The brain-inspired artificial neural networks that computer scientists have built for companies like Facebook and Google simply learn to recognize complex patterns in images. If it identifies the pattern, say the shape of a cat coupled with details of a cat's fur, that's a cat to the algorithm.
So what the researchers pulled off, he continued, was to reverse-engineer the patterns that AI looks for in images via adversarial example.
"By changing an image of a school bus just 3%, one Google team was able to fool AI into seeing an ostrich," Gershgorn said.
What's the point? Swapna Krishna in Engadget: "It's important because this issue isn't limited to Google—it's a problem in all neural networks. By figuring out how people can fool these systems (and demonstrating that it can be relatively easily and reliably done), researchers can devise new ways to make AI recognition systems more accurate."
Gershgorn in Quartz: "Neural networks blow all previous techniques out of the water in terms of performance, but given the existence of these adversarial examples, it shows we really don't understand what's going on." He quoted co-author Athalye: "If we don't manage to find good defenses against these, there will come a time where they are attacked."
Adam Conner-Simons, MIT CSAIL, wrote about their work in CSAIL News: (The Computer Science and Artificial Intelligence Laboratory).
"The project builds on a growing body of work in 'adversarial examples.' For many years researchers have been able to show that changing pixels can fool neural networks, but such corner-cases have often been viewed more as an intellectual curiosity than as something to be concerned about in the real-world."
More information: — Synthesizing Robust Adversarial Examples, arXiv:1707.07397 [cs.CV] arxiv.org/abs/1707.07397
Abstract
Neural network-based classifiers parallel or exceed human-level accuracy on many common tasks and are used in practical systems. Yet, neural networks are susceptible to adversarial examples, carefully perturbed inputs that cause networks to misbehave in arbitrarily chosen ways. When generated with standard methods, these examples do not consistently fool a classifier in the physical world due to viewpoint shifts, camera noise, and other natural transformations. Adversarial examples generated using standard techniques require complete control over direct input to the classifier, which is impossible in many real-world systems. We introduce the first method for constructing real-world 3D objects that consistently fool a neural network across a wide distribution of angles and viewpoints. We present a general-purpose algorithm for generating adversarial examples that are robust across any chosen distribution of transformations. We demonstrate its application in two dimensions, producing adversarial images that are robust to noise, distortion, and affine transformation. Finally, we apply the algorithm to produce arbitrary physical 3D-printed adversarial objects, demonstrating that our approach works end-to-end in the real world. Our results show that adversarial examples are a practical concern for real-world systems.
— www.labsix.org/physical-object … at-fool-neural-nets/
© 2017 Tech Xplore