May 25, 2018 report
An algorithm for detecting when online conversations are likely to get ugly
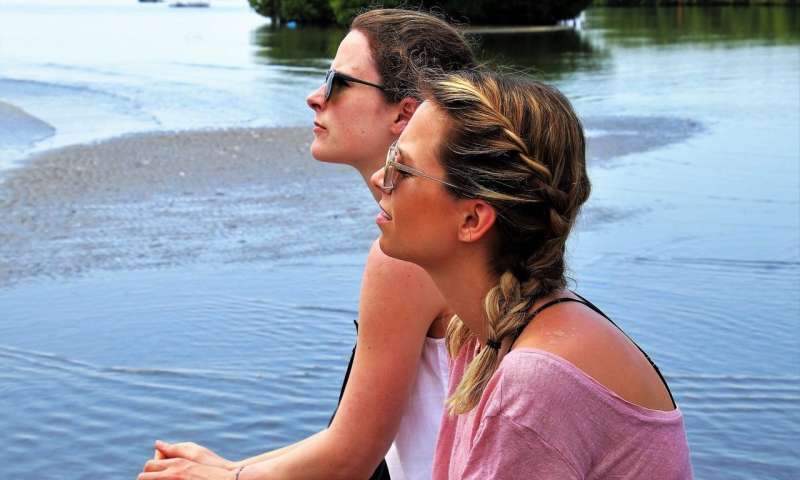
A team of researchers at Cornel University working with the Wikimedia Foundation has come up with a digital framework for detecting when an online discussion is likely to get ugly. In a paper uploaded to the arXiv preprint server, the team describes their approach and how well their algorithm worked during testing.
As the researchers note, online conversations can often degenerate into disagreements and often personal attacks. They further note that this is often the case when people enter an environment that involves criticism, such as Wikipedia. There, amateur editors are encouraged to offer critiques of work by others as a means of improving the content on the website. Unfortunately, a lot of people do not respond well to such criticism, and as a result, resort to posting nasty comments. The team at the Wikimedia Foundation would like to curb such conversations, because in addition to fostering bad feelings, it also gives the site a bad reputation. To address the problem, the team worked with the group at Cornell, who have been researching the same problem; namely, building a computer system that is able to recognize when a human conversation is likely to degenerate into nastiness, and to either curb it, or end the conversation for the people involved.
To solve this problem, the researchers looked at over 1,200 online conversations on the Wikipedia Talk pages looking for linguistic cues. In this context, cues were words that suggested demeanor and level of politeness. In so doing, they found that when people used cues such as "please" and "thanks," there was less of a chance of things getting ugly. There were also positive phrases, such as "I think" or "I believe" that suggested an attempt to keep things civil, which tended to keep things on an even keel. On the other hand, they also found less helpful cues, such as when conversations started with direct questions or the word "you." Such cues tended to lead to degradation in civility at some point and, the researchers suggest, are often seen by a reader as hostile and contentious.
The team then developed an algorithm that accepted cues as learned data and then parsed sentences searching for such cues and applying human-like intuition to them. The result, the team reports, was a computerized framework that could recognize early when a conversation was likely to degenerate into an ugly game of back and forth. They found the system to be 61.6 percent accurate. Humans doing the same test, however, scored 72 percent.
More information: Conversations Gone Awry: Detecting Early Signs of Conversational Failure, arXiv:1805.05345 [cs.CL] arxiv.org/abs/1805.05345
Abstract
One of the main challenges online social systems face is the prevalence of antisocial behavior, such as harassment and personal attacks. In this work, we introduce the task of predicting from the very start of a conversation whether it will get out of hand. As opposed to detecting undesirable behavior after the fact, this task aims to enable early, actionable prediction at a time when the conversation might still be salvaged.
To this end, we develop a framework for capturing pragmatic devices—-such as politeness strategies and rhetorical prompts—-used to start a conversation, and analyze their relation to its future trajectory. Applying this framework in a controlled setting, we demonstrate the feasibility of detecting early warning signs of antisocial behavior in online discussions.
© 2018 Phys.org