June 20, 2018 weblog
Assessment of death risks: It's Google's turn
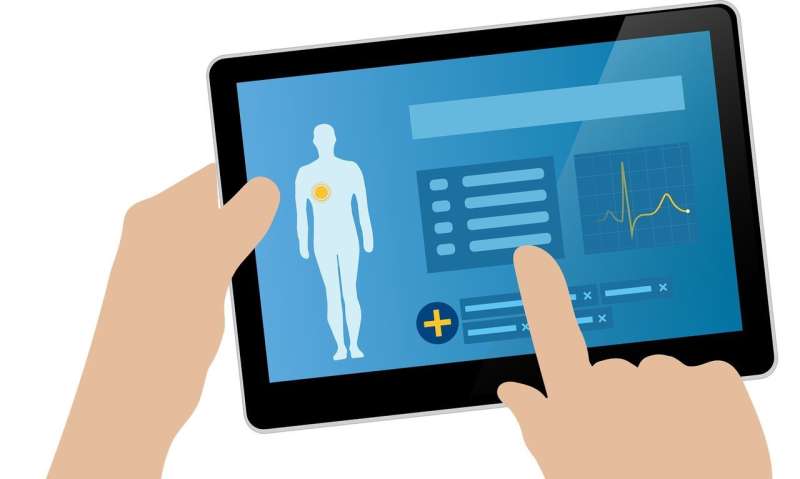
Realistic assessments of end of life may be more accurate because of AI and neural networks.
Google's scientists are looking at the AI potential for use in medicine and the results are making the rounds of tech-watching sites posting eye-catching headlines. Google is training machines to make accurate assessments about in-patient mortality. The reports referred to the company's Medical Brain team.
As Daily Mail pointed out, the AI was developed in collaboration with colleagues at UC San Francisco, Stanford Medicine and The University of Chicago Medicine.
For sure, they are not the first to explore the use of computer systems to learn from a clinical data database. The idea of using computer systems to learn from a "highly organized and recorded database" of clinical data has a long history, they said.
Problem is that predictive models built with EHR data use a median of only 27 variables, they wrote, rely on traditional generalized linear models, and are built using data at a single center. They on the other hand constructed a study of deep learning in a variety of prediction problems based on multiple general hospital data.
"If a clinical team had to investigate patients predicted to be at high risk of dying, the rate of false alerts at each point in time was roughly halved by our model," they reported.
"Scalable and accurate deep learning with electronic health records" is the title of their paper, published in npj Digital Medicine in May.
"The promise of digital medicine stems in part from the hope that, by digitizing health data, we might more easily leverage computer information systems to understand and improve care," they wrote.
The key to all this is predictive modeling with electronic health record (EHR) data. "We propose a representation of patients' entire raw EHR records based on the Fast Healthcare Interoperability Resources (FHIR) format. We demonstrate that deep learning methods using this representation are capable of accurately predicting multiple medical events from multiple centers without site-specific data harmonization."
The Los Angeles Times quoted one of the authors: "As much as 80% of the time spent on today's predictive models goes to the 'scut work' of making the data presentable," said Nigam Shah, an associate professor at Stanford University and co-author of the paper. However, with Google's approach, said Shah, "You can throw in the kitchen sink and not have to worry about it."
Victor Tangermann in Futurism said that "The neural network even includes handwritten notes, comments, and scribbles on old charts to make its predictions."
The authors kicked the tires of traditional modeling approaches and called out limitations they posed. They said such approaches dealt with complexity by choosing a limited number of commonly collected variables to consider. "This is problematic because the resulting models may produce imprecise predictions: false-positive predictions can overwhelm physicians, nurses, and other providers with false alarms and concomitant alert fatigue."
They spoke of deep learning and neural networks to unlock information needed for a more complete picture.
Futurism said, "In trials using data from two U.S. hospitals, researchers were able to show that these algorithms could predict a patient's length of stay and time of discharge, but also the time of death."
They included a total of 216,221 hospitalizations involving 114,003 unique patients.
"To the best of our knowledge, our models outperform existing EHR models in the medical literature for predicting mortality (0.92–0.94 vs 0.91), unexpected readmission (0.75–0.76 vs 0.69), and increased length of stay (0.85–0.86 vs 0.77)."
The authors commented on data availability. "The datasets analysed during the current study are not publicly available: due to reasonable privacy and security concerns, the underlying EHR data are not easily redistributable to researchers other than those engaged in the Institutional Review Board-approved research collaborations with the named medical centers."
More information: Alvin Rajkomar et al. Scalable and accurate deep learning with electronic health records, npj Digital Medicine (2018). DOI: 10.1038/s41746-018-0029-1
Abstract
Predictive modeling with electronic health record (EHR) data is anticipated to drive personalized medicine and improve healthcare quality. Constructing predictive statistical models typically requires extraction of curated predictor variables from normalized EHR data, a labor-intensive process that discards the vast majority of information in each patient's record. We propose a representation of patients' entire raw EHR records based on the Fast Healthcare Interoperability Resources (FHIR) format. We demonstrate that deep learning methods using this representation are capable of accurately predicting multiple medical events from multiple centers without site-specific data harmonization. We validated our approach using de-identified EHR data from two US academic medical centers with 216,221 adult patients hospitalized for at least 24 h. In the sequential format we propose, this volume of EHR data unrolled into a total of 46,864,534,945 data points, including clinical notes. Deep learning models achieved high accuracy for tasks such as predicting: in-hospital mortality (area under the receiver operator curve [AUROC] across sites 0.93–0.94), 30-day unplanned readmission (AUROC 0.75–0.76), prolonged length of stay (AUROC 0.85–0.86), and all of a patient's final discharge diagnoses (frequency-weighted AUROC 0.90). These models outperformed traditional, clinically-used predictive models in all cases. We believe that this approach can be used to create accurate and scalable predictions for a variety of clinical scenarios. In a case study of a particular prediction, we demonstrate that neural networks can be used to identify relevant information from the patient's chart.
© 2018 Tech Xplore