August 31, 2018 report
Machine learning enables physics-inspired metrics for analyzing art
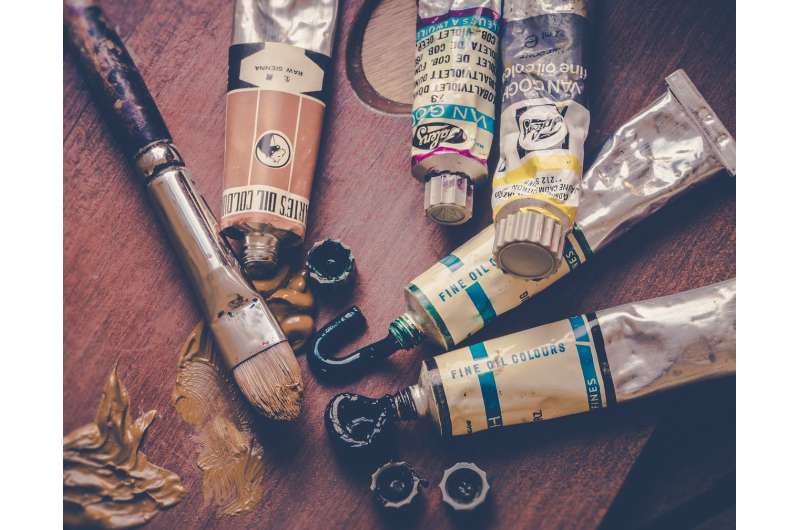
An international research collaborative reports that a systematized AI analysis of artwork produced over the last millenium yields revealing information about historical evolutionary artistic trends. Additionally, the results map well to canonical concepts about styles and periods of art history.
Art analysis is usually comparative, and has historically been conducted by individual researchers, which places constraints on the scale of studies. It is impractical for a single scholar to compare more than a handful of paintings at a time. However, in recent decades, a vast amount of historical artwork has been digitized and made freely available, enabling quantitative approaches to art analysis that were previously unfeasible, if not impossible.
In their new study, published by the Proceedings of the National Academy of Sciences, the researchers analyzed a dataset of 137,364 pieces of visual art, mostly paintings, hosted by the online encyclopedia WikiArt. The site contains artworks by over 2,000 artists in over 100 styles.
Each file was converted to a matrix representation. By applying machine learning algorithms, the researchers analyzed the relationships between adjacent pixels, and calculated two measures of complexity: the normalized permutation entropy H, and the statistical complexity C.
The H value quantifies the degree of disorder in the pixel arrangement of an image. For instance, a value close to zero indicates a regular image such as those produced by minimalist painters. A value close to one indicates pixels that seem irregular or more disordered, such as the drip paintings of Jackson Pollock.
The statistical complexity C is a measure of the structural complexity of the work. Paintings that present extremes of either disorder or order in pixel arrangement yield zero, as such works have low structural complexity. The value is positive when the system detects more complex spatial patterns.
Combining these two measures produces a complexity–entropy plane, which the authors point out is a technique that has been applied in many other fields. Not only could these measures predict the style and period of the paintings within a certain margin of error; their analysis revealed a clear trajectory of art over 1000 years with transitions in the complexity–entropy plane that correspond to the canonical periods in art literature.
Specifically, the researchers could clearly see distinct changes in entropy and complexity corresponding to the periods before before and after Modern art, and the transition from Modern art to Postmodern art. They plot these transitions on a timeline, and report that "it is not difficult to envisage that the transition from Modern to Postmodern was driven by the end of World War II, the event that usually marks the beginning of Postmodernism in history books."
The researchers point out that because they restricted their analysis to these two measures of complexity, it is not possible to fully capture the informational richness that is likely to be encoded in art. "However," they write, "our results nevertheless demonstrate that simple physics-inspired metrics can be connected to concepts proposed by art historians and, more importantly, that these measures do carry relevant information about artworks, their style, and evolution."
More information: History of art paintings through the lens of entropy and complexity. PNAS. doi.org/10.1073/pnas.1800083115
Abstract
Art is the ultimate expression of human creativity that is deeply influenced by the philosophy and culture of the corresponding historical epoch. The quantitative analysis of art is therefore essential for better understanding human cultural evolution. Here, we present a large-scale quantitative analysis of almost 140,000 paintings, spanning nearly a millennium of art history. Based on the local spatial patterns in the images of these paintings, we estimate the permutation entropy and the statistical complexity of each painting. These measures map the degree of visual order of artworks into a scale of order–disorder and simplicity–complexity that locally reflects qualitative categories proposed by art historians. The dynamical behavior of these measures reveals a clear temporal evolution of art, marked by transitions that agree with the main historical periods of art. Our research shows that different artistic styles have a distinct average degree of entropy and complexity, thus allowing a hierarchical organization and clustering of styles according to these metrics. We have further verified that the identified groups correspond well with the textual content used to qualitatively describe the styles and the applied complexity–entropy measures can be used for an effective classification of artworks.
© 2018 Tech Xplore