January 21, 2019 feature
Researchers develop a machine learning method to identify fake honey
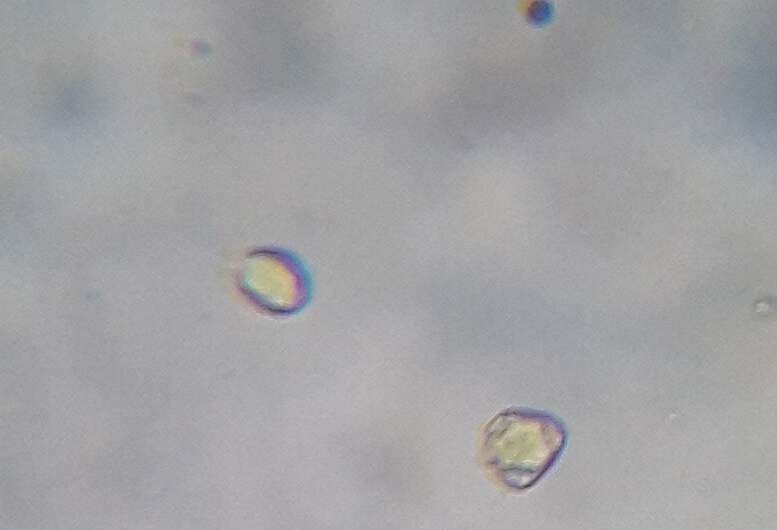
A team of researchers at Imperial College London and UCL have recently developed a new method to authenticate honey using machine learning and microscopy. Their technique, outlined in a paper pre-published on arXiv, could detect diluted or mislabeled honey at a far lower cost than existing methods.
Honey is produced by bees after they collect nectar from flowers, break it down into simple sugars and store it inside honeycombs. Honey is currently the third most counterfeited food product globally. It is often mislabeled, which entails selling one type of honey for another, or is diluted it with other substances, such as sugar syrup.
"Honey is made by bees from plants," Gerard Glowacki, one of the researchers who carried out the study, told TechXplore. "Plants have pollen, and each plant has a different pollen. If Manuka honey, for instance, has no Manuka pollen or no pollen at all, then it's not Manuka honey."
Fake honey costs significantly less to produce, and this can adversely affect producers of genuine honey, forcing them to lower their profit margins or sometimes to leave the market altogether. In addition, beekeeping practices in the production of fake honey are often sub-par compared to practices in genuine honey farming, which can result in the mistreatment of bee colonies. Effective and low-cost methods to authenticate honey could help to identify fake honey quickly, so that it can be removed from the market or re-labeled correctly.
"Melissopalynology, authenticating honey from its botanical sources, has been around for a good few decades, with a reputation of being a slow and specialist process," Peter He, one of the researchers who carried out the study, told TechXplore. "We thought that we'd be able to speed things up with an operator that didn't suffer from human things like tiredness, forgetfulness and boredom."
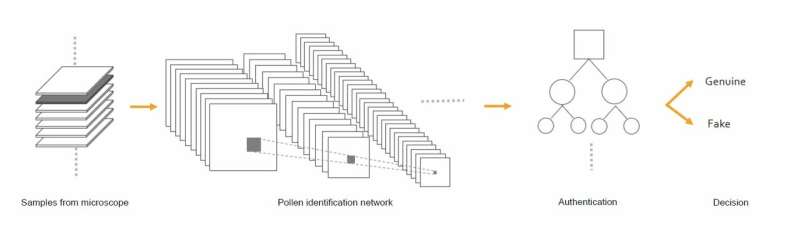
The most commonly employed honey authentication methods include quantitative polymerase chain reaction (qPCR), nuclear magnetic resonance spectroscopy (NMR), liquid chromatography mass spectrometry (LC-MS), near-infrared spectroscopy (NIR) and microscopy. Researchers have also developed other tests for the identification of specific types of honey, but most of these have so far proved to be ineffective.
The current state-of-the-art technique to authenticate manuka honey, a widely sought after type of honey made from the nectar of manuka flowers and typically produced in New Zealand, is based on four chemical markers and the use of a test for the DNA of manuka pollen. This method, however, can only be used to authenticate manuka honey and is not applicable to other types of honey.
Most honey authentication procedures are carried out in labs by specialists and require specialized equipment, hence they are often very expensive. In their study, Glowacki, He and their colleague Alexis Gkantiragas developed a new method to authenticate honey using machine learning-augmented microscopy, which could be far cheaper than existing procedures.
"We identify the pollen in honey samples using standard deep learning techniques," Gkantiragas explained. "From this, we can apply more quantitative approaches to analyze things such as the distribution and density of the pollen. We can then identify the honey's geographical and/or botanical origin."
The researchers collected samples of different types of honey and spread them across glass slides. These slides were covered and analyzed using a bright-field microscope, capturing approximately 2500 microscopic images of pollen.
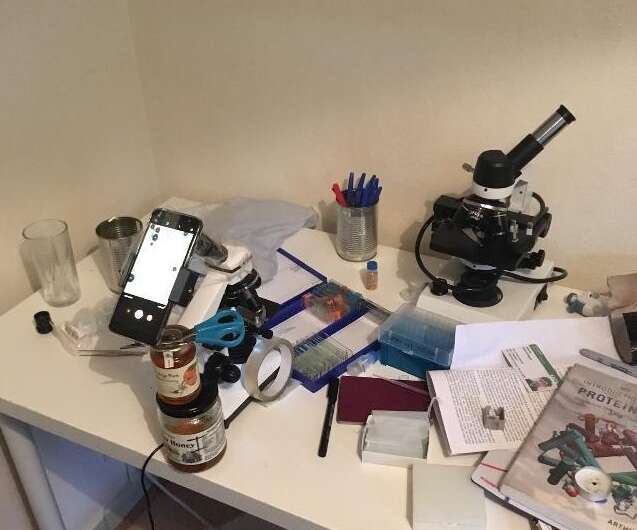
After carefully labeling and annotating these images, the researchers used them to train their machine learning model. Their model consists of a segmentation network, trained to detect and segment pollen, as well as an authentication network, trained to classify different types of honey.
"It's currently hard to tell fake from real honey," Gkantiragas said. "Sugar testing can be fooled by using different sugars. NMR is expensive and needs professionals. Our equipment costs pocket money, is straightforward to use and has the potential to be deployed at scale."
In preliminary evaluations, the researchers found that their honey authentication method could effectively detect diluted and mislabeled honey. However, it is unable to identify contamination with heavy metals, pesticides or antibiotics, hence it might need to be used in combination with other chemical tests. In addition, their method cannot be used to authenticate ultra-filtered honey samples in which no pollen is present.
Although the results gathered by the researchers are promising, their system needs to be developed further before it can be applied on a larger scale. For instance, the researchers need to collect a wider pollen dataset in order to better capture the diversity of pollens in honey.
"An important step in scaling the system from research to the real world would be robustifying the system to be hardware-agnostic," He explained. "We're looking at, among other things, adversarial training methods to ensure that our feature representations are top notch."
The researchers are planning to continue working on their system to ensure that it can effectively authenticate honey in real-world scenarios. In future, they might even consider piloting a decentralized certification scheme based on their technology.
More information: Honey authentication with machine learning augmented bright-field microscopy. arXiv:1901.00516 [cs.LG]. arxiv.org/abs/1901.00516
© 2019 Science X Network