May 21, 2019 feature
CycleMatch: a new approach for matching images and text
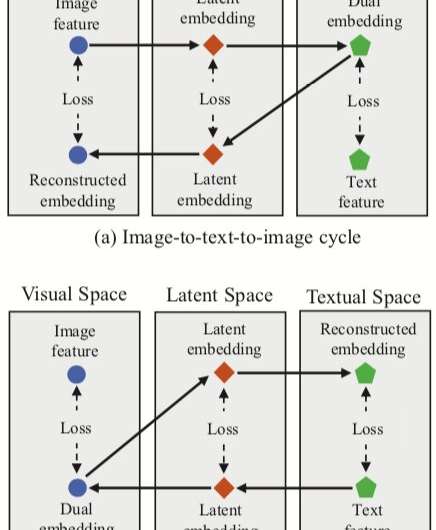
Researchers at Leiden University and the National University of Defense Technology (NUDT), in China, have recently developed a new approach for image-text matching, called CycleMatch. Their approach, presented in a paper published in Elsevier's Pattern Recognition journal, is based on cycle-consistent learning, a technique that is sometimes used to train artificial neural networks on image-to-image translation tasks. The general idea behind cycle-consistency is that when transforming source data into target data and then vice versa, one should finally obtain the original source samples.
When it comes to developing artificial intelligence (AI) tools that perform well in multi-modal or multimedia-based tasks, finding ways to bridge images and text representations is of crucial importance. Past studies have tried to achieve this by uncovering semantics or features that are relevant to both vision and language.
When training algorithms on correlations between different modalities, however, these studies have often neglected or failed to address intra-modal semantic consistency, which is the consistency of semantics for the individual modalities (i.e. vision and language). To address this shortcoming, the team of researchers at Leiden University and NUDT proposed an approach that applies cycle-consistent embeddings to a deep neural network for matching visual and textual representations.
"Our approach, named as CycleMatch, can maintain both inter-modal correlations and intra-modal consistency by cascading dual mappings and reconstructed mappings in a cyclic fashion," the researchers wrote in their paper. "Moreover, in order to achieve a robust inference, we propose to employ two late-fusion approaches: average fusion and adaptive fusion."
The approach devised by the researchers integrates three feature embeddings (dual, reconstructed and latent embeddings) with a neural network for image-text matching. The method has two cycle branches, one starting from an image feature in the visual space and one from a text feature in the textual space.
For each of these cycles, their approach achieves a dual mapping, translating an input feature in the source space into a dual embedding in the target space. The researchers then apply reconstructed mapping, trying to translate this dual embedding back to the source space.
Their approach also allows the researchers to acquire a 'latent space' during both dual and reconstructed mappings, and subsequently correlate latent embeddings. Contrarily to other techniques for image-text matching, therefore, their method can learn both inter-modal mappings (i.e. image-to-text and text-to-image) and intra-modal mappings (image-to-image and text-to-text).
To evaluate their approach, the researchers carried out a series of experiments using two renowned multi-modal datasets, Flickr30K and MSCOCO. Their method achieved state-of-the-art results, outperforming traditional approaches and leading to significant improvements in cross-modal retrieval.
These findings suggest that cycle-consistent embeddings could enhance the performance of neural networks in multi-modal tasks, such as image-text matching, allowing them to acquire both inter-modal and intra-modal mappings. In their future work, the researchers plan to develop their approach further, by taking into account local relations in matching images and text (e.g. semantic correlations between visual regions and phrases).
More information: Yu Liu et al. CycleMatch: A cycle-consistent embedding network for image-text matching, Pattern Recognition (2019). DOI: 10.1016/j.patcog.2019.05.008
© 2019 Science X Network