June 11, 2019 feature
A new approach for unsupervised paraphrasing without translation
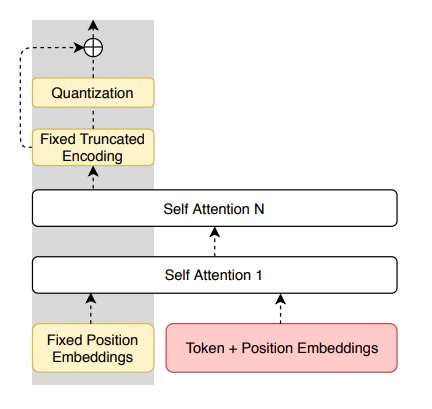
In recent years, researchers have been trying to develop methods for automatic paraphrasing, which essentially entails the automated abstraction of semantic content from text. So far, approaches that rely on machine translation (MT) techniques have proved particularly popular due to the lack of available labeled datasets of paraphrased pairs.
Theoretically, translation techniques might appear like effective solutions for automatic paraphrasing, as they abstract semantic content from its linguistic realization. For instance, assigning the same sentence to different translators might result in different translations and a rich set of interpretations, which could be useful in paraphrasing tasks.
Although many researchers have developed translation-based methods for automated paraphrasing, humans do not necessarily need to be bilingual to paraphrase sentences. Based on this observation, two researchers at Google Research have recently proposed a new paraphrasing technique that does not rely on machine translation methods. In their paper, pre-published on arXiv, they compared their monolingual approach to other techniques for paraphrasing: a supervised translation and an unsupervised translation approach.
"This work proposes to learn paraphrasing models from an unlabeled monolingual corpus only," Aurko Roy and David Grangier, the two researchers who carried out the study, wrote in their paper. "To that end, we propose a residual variant of vector-quantized variational auto-encoder."
The model introduced by the researchers is based on vector-quantized auto-encoders (VQ-VAE) that can paraphrase sentences in a purely monolingual setting. It also has a unique feature (i.e. residual connections parallel to the quantized bottleneck), which enables better control over the decoder entropy and eases optimization.
"Compared to continuous auto-encoders, our method permits the generation of diverse, but semantically close sentences from an input sentence," the researchers explained in their paper.
In their study, Roy and Grangier compared their model's performance with that of other MT-based approaches on paraphrase identification, generation and training augmentation. They specifically compared it with a supervised translation method trained on parallel bilingual data and an unsupervised translation method trained on non-parallel text in two different languages. Their model, on the other hand, only requires unlabeled data in a single language, the one it is paraphrasing sentences in.
The researchers found that their monolingual approach outperformed unsupervised translation techniques in all tasks. Comparisons between their model and supervised translation methods, on the other hand, yielded mixed results: the monolingual approach performed better in identification and augmentation tasks, while the supervised translation method was superior for paraphrase generation.
"Overall, we showed that monolingual models can outperform bilingual ones for paraphrase identification and data-augmentation through paraphrasing," the researchers concluded. "We also reported that generation quality from monolingual models can be higher than models based on unsupervised translation, but not supervised translation."
Roy and Grangier's findings suggest that using bilingual parallel data (i.e. texts and their possible translations in other languages) is particularly advantageous when generating paraphrases and leads to remarkable performance. In situations where bilingual data is not readily available, however, the monolingual model proposed by them could be a useful resource or alternative solution.
More information: Unsupervised paraphrasing without translation. arXiv:1905.12752 [cs.LG]. arxiv.org/abs/1905.12752
© 2019 Science X Network