The next step in AI? Mimicking a baby's brain
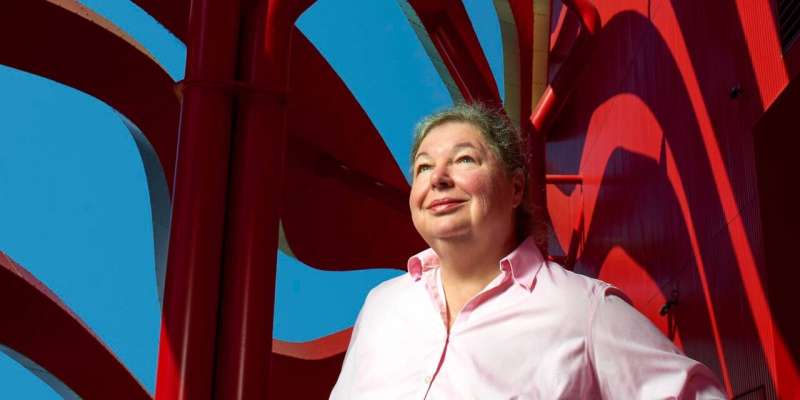
The phrase "positive reinforcement," is something you hear more often in an article about child rearing than one about artificial intelligence. But according to Alice Parker, Dean's Professor of Electrical Engineering in the Ming Hsieh Department of Electrical and Computer Engineering, a little positive reinforcement is just what our AI machines need. Parker has been building electronic circuits for over a decade to reverse-engineer the human brain to better understand how it works and ultimately build artificial systems that mimic it. Her most recent paper, co-authored with Ph.D. student Kun Yue and colleagues from UC Riverside, was just published in the journal Science Advances and takes an important step towards that ultimate goal.
The AI we rely on and read about today is modeled on traditional computers; it sees the world through the lens of binary zeros and ones. This is fine for making complex calculations but, according to Parker and Yue, we're quickly approaching the limits of the size and complexity of problems we can solve with the platforms our AI exists on. "Since the initial deep learning revolution, the goals and progress of deep-learning based AI as we know it has been very slow," Yue says. To reach its full potential, AI can't simply think better—it must react and learn on its own to events in real time. And for that to happen, a massive shift in how we build AI in the first place must be conceived.
To address this problem, Parker and her colleagues are looking to the most accomplished learning system nature has ever created: the human brain. This is where positive reinforcement comes into play. Brains, unlike computers, are analog learners and biological memory has persistence. Analog signals can have multiple states (much like humans). While a binary AI built with similar types of nanotechnologies to achieve long-lasting memory might be able to understand something as good or bad, an analog brain can understand more deeply that a situation might be "very good," "just okay," "bad" or "very bad." This field is called neuromorphic computing and it may just represent the future of artificial intelligence.
When humans are exposed to something new and potentially useful our neurons get a spike of dopamine and the connections surrounding those neurons strengthen. "Think of an infant sitting in a high-chair," Parker says. "She might be waving her arms around wildly because her undeveloped neurons are just randomly firing." Eventually one of those wild movements leads to a positive result—say, knocking her cup over and making a mess. All of a sudden, the neurons that made that motion get a response and strengthen. Done regularly enough, the baby's brain begins to associate that spike with something worth internalizing. And just like that, our little baby has learned that an arm motion causes an entertaining result and that learning persists over time. This is exactly what neuromorphic computing is trying to do: teach AI to learn from real-world experiences exactly as we do.
To do this, Parker and Yue have designed their own neuromorphic circuits and combined them with nanodevices called Magnetic Domain Wall Analog Memristors (MAM). They then run simulations to show that their neural circuits learn like a brain. This MAM device is so complex that an entire article could be written on it alone. But for now, the most important thing to know is that it's an extremely small device which helps indefinitely remember the positive reinforcement "spike" that the artificial neurons receive. You can think of Parker's neuromorphic circuits combined with the MAM exactly like that little baby's brain. In that sense, Parker and Yue are kind of like the little AI baby's parents…teaching it new things and positively reinforcing it when it does something right.
For the time being, what we have is a little bit like a real baby's brain. Undeveloped and definitively not ready to make decisions on its own. But, also very much like a real baby, with enough work, investment, and love from the researchers, this technology will change the way AI works in the real world.
Of course, Parker's work is never really finished. "Our next step, working with DARPA, is to teach our system to learn something new without forgetting previous lessons," Parker says. Their work may represent one small step towards the ultimate goal of neuromorphic AI, but like any good researcher or parent, Parker appreciates the importance of baby steps.
More information: Kun Yue et al. A brain-plausible neuromorphic on-the-fly learning system implemented with magnetic domain wall analog memristors, Science Advances (2019). DOI: 10.1126/sciadv.aau8170