How CERN machine-learning techniques could improve autonomous vehicles
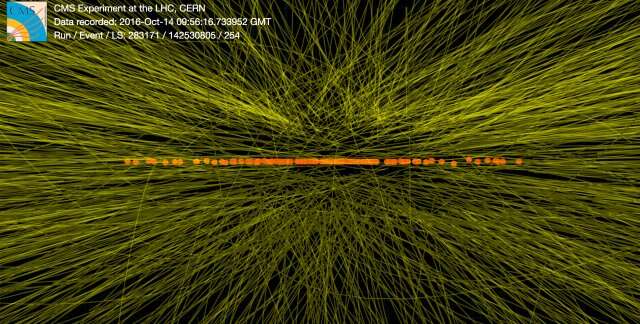
With about one billion proton–proton collisions per second at the Large Hadron Collider (LHC), the LHC experiments need to sift quickly through the wealth of data to choose which collisions to analyse. To cope with an even higher number of collisions per second in the future, scientists are investigating computing methods such as machine-learning techniques. A new collaboration is now looking at how these techniques deployed on chips known as field-programmable gate arrays (FPGAs) could apply to autonomous driving, so that the fast decision-making used for particle collisions could help prevent collisions on the road.
FPGAs have been used at CERN for many years and for many applications. Unlike the central processing unit of a laptop, these chips follow simple instructions and process many parallel tasks at once. With up to 100 high-speed serial links, they are able to support high-bandwidth inputs and outputs. Their parallel processing and re-programmability make them suitable for machine-learning applications.
The challenge, however, has been to fit complex deep-learning algorithms—a particular class of machine-learning algorithms—in chips of limited capacity. This required software developed for the CERN-based experiments, called "hls4ml," which reduces the algorithms and produces FPGA-ready code without loss of accuracy or performance, allowing the chips to execute decision-making algorithms in micro-seconds.
A new collaboration between CERN and Zenuity, the autonomous driving software company headquartered in Sweden, plans to use the techniques and software developed for the experiments at CERN to research their use in deploying deep learning on FPGAs, a particular class of machine-learning algorithms, for autonomous driving. Instead of particle-physics data, the FPGAs will be used to interpret huge quantities of data generated by normal driving conditions, using readouts from car sensors to identify pedestrians and vehicles. The technology should enable automated drive cars to make faster and better decisions and predictions, thus avoiding traffic collisions.
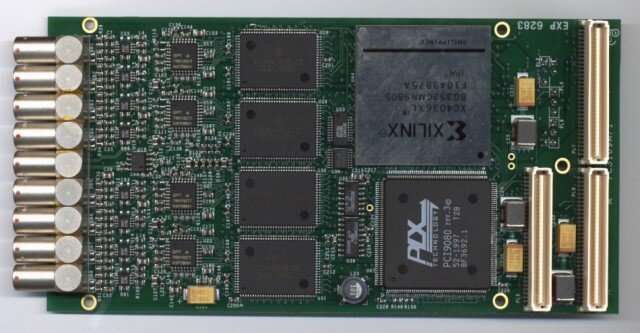
More information: To find out more about CERN technologies and their potential applications, visit kt.cern/technologies