September 26, 2019 feature
FoodTracker: An AI-powered food detection mobile application
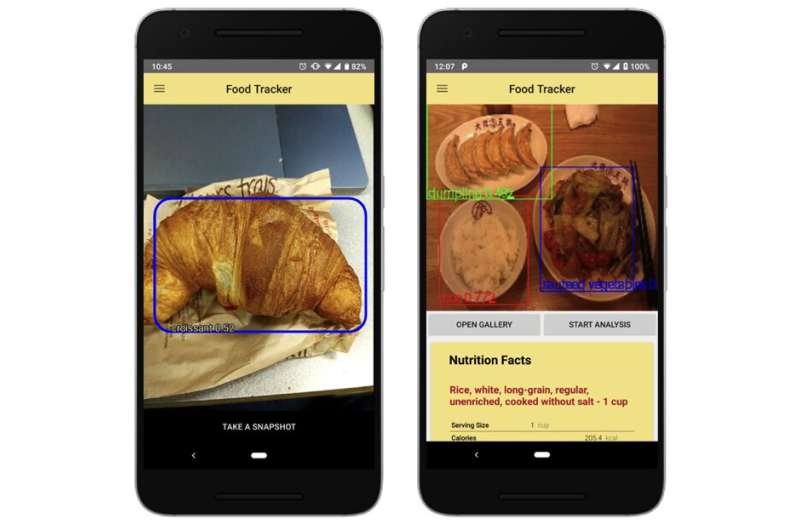
A research team at McGill University in Canada has developed a mobile application that can recognize food items inside an overall meal in real-time, providing useful nutrition-related information. The team outlined the new mobile application, called FoodTracker, in a recent paper pre-published on arXiv and presented at the 16th International Conference on Machine Vision Applications in Tokyo.
"Our lab focuses on healthcare-related applications on embedded systems," Zelijko Zilic one of the researchers who carried out the study, told TechXplore. "We aim to bring automation into food journaling, so that people who care about they daily consumption or patients can keep tracking meal items and nutrition contents in their daily life. Towards that goal, we have been providing apps (DiaBeatMove and CarbAndMove) for iPhone that assist diabetics and pre-diabetics in managing the exercise, nutrition, insulin and health-related aspects of their life."
The rise in obesity rates and issues associated with poor nutrition both in the US and other countries worldwide has encouraged numerous researchers to develop mobile applications or online platforms that promote healthier lifestyle choices. In their recent study, Zilic and his colleagues specifically set out to develop an application for smartphones that can rapidly and effectively recognize the food that a user is consuming in real-time, offering nutrition facts for each component of a meal.
FoodTracker, the mobile app developed by the researchers, is very easy to use. When a user points his smartphone camera to a plate containing his/her meal, the app rapidly recognizes its different ingredients.
"The key advantage is our FoodTracker app, is it that it does not rely on any manual data entry—achieve automatic food monitoring and nutrition analysis, solely based on images from mobile cameras," Jianing Sun, another researcher involved in the study, told TechXplore.
First of all, Zilic, Sun and their colleagues developed a model that combines a deep convolutional neural network (CNN) with YOLO, a state-of-the-art detection strategy. They trained this model using a wide database of food images and found that it has an average precision in detecting food items based on images of almost 80 percent.
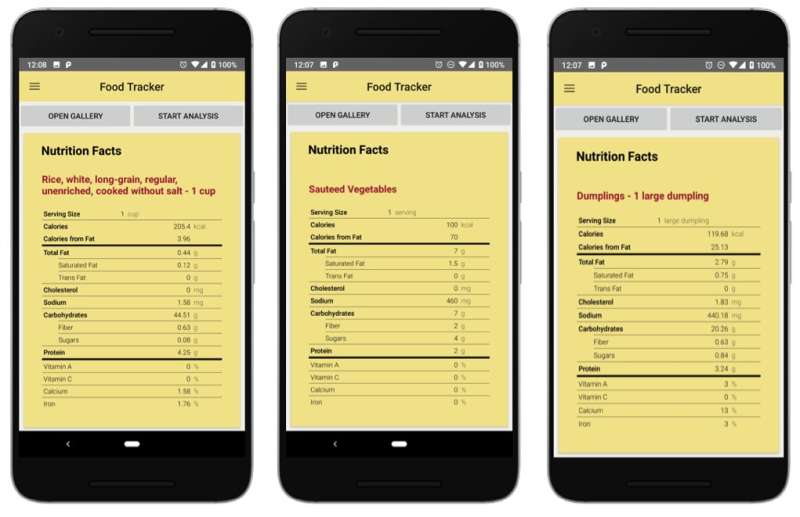
"Using our scheme, we showed that even modestly powered smartphones can undertake the required food recognition," Zilic said. "Among the most meaningful findings are the short inference time, low runtime memory, and high accuracy of FoodTracker, which make it very practical and easy to use."
Subsequently, the researchers integrated their model into the FoodTracker application, with an additional feature for nutrition analysis. This allows the app to provide important nutrition-related information (e.g. calories, amounts, etc.) for each food component detected by the CNN-based model.
Initial evaluations suggest that the FoodTracker app is a promising tool for the real-time identification of food and for providing nutrition guidance. Moreover, despite its AI-component, the app can also be used on mobile devices with negligible inference time and it has small memory requirements.
"When I was in Japan to present this work, I found there were many industrial researchers are working on food detection with recently emerging computer vision techniques," Sun said. "I was even invited to an international food tech company that has business in more than 70 countries. I feel that people are paying increasing attention to their daily consumption for health purposes."
In the future, mobile applications such as FoodTracker could broaden people's knowledge about nutrition, supporting them in becoming more aware of the food they consume on a daily basis and perhaps even helping them to improve their eating habits. Zilic, Sun and their colleagues are now planning to integrate the nutrition-related guidance provided by the app with other modules that encourage a healthier lifestyle.
"The fully automatic application that can detect food objects and extract nutrition contents is very complicated, especially when it comes to real life application," Katarzyna Radecka, another researcher who carried out the study, told TechXplore. "Our work takes an initial step towards it but there are for sure more work should be followed, e.g. multi-task learning, robustness and better generalization, volume estimation. We believe that even a partial solution to these problems could be of great value to society."
More information: FoodTracker: A real-time food detection mobile application by deep convolutional neural networks. arXiv:1909.05994 [cs.CV]. arxiv.org/abs/1909.05994
© 2019 Science X Network