Mobile phone signals provide occupancy numbers for buildings
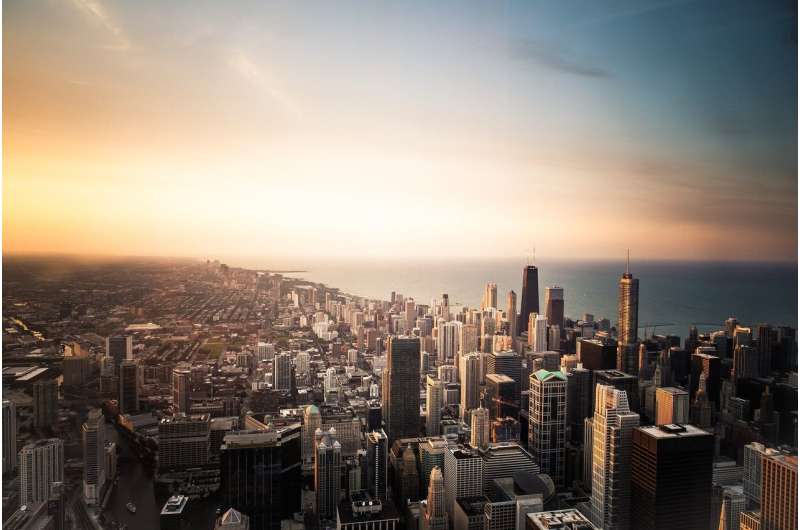
Buildings currently consume about 40 percent of all the electricity used in the United States, most of them located in urban areas that are growing rapidly. Because electricity generation is the largest source of greenhouse gas emissions in the country, making urban buildings more energy efficient could help mitigate global climate change.
In order to achieve efficient buildings at a city-wide scale, accurate occupancy estimations are crucial. These estimates need to take into account the fact that people move around their cities throughout the day, from home to work, which drives energy consumption for different building types. Now, a model developed by researchers from Berkeley Engineering, Berkeley Lab and MIT can do just that. A paper describing the tool, which uses passively collected cellphone data to improve urban scale building occupancy and mobility estimates, was recently published in Nature Communications.
"Understanding building occupancy at an urban-scale allows us to plan better for collective energy use. Like traffic apps that tell you the current state of road congestion, we envision a model that could potentially tell users what the energy demands are in different places and therefore identify bespoke efficiency measures. The tool could also potentially connect to smart-devices that automatically adjust to the energy demand," said Marta Gonzalez, professor of civil and environmental engineering at Berkeley and co-author of the paper.
While passive data sources like Bluetooth, Wi-Fi and cameras have been used to understand the dynamics of a city, Gonzalez and her collaborators argue that these sources are not available at a sufficient scale to do that job comprehensively. These limited sources cannot accurately predict the simultaneous occupancy of thousands of different buildings. This is why the researchers propose using passively collected mobile phone data to infer building occupancy at a city-level.
As a proof-of-concept, they integrated the call records of 1.92 million anonymous mobile phone users in the Boston metropolitan area with an existing framework called TimeGeo, which identified urban mobility patterns. In this data, they looked for people who made consecutive mobile phone calls within the same location of 300 meters and had conversations lasting about 10 minutes. These "stay points" were characterized as home, work or other.
In this data, which was collected between late February and March 2010, they found 200,000 people who had more than 50 stays and at least 10 home stays, which are calls that occurred at home designated buildings. From this selection, they extracted mobility parameters that were then applied to a simulation that modeled the mobility of 3.54 million people in the Boston area, including 2.10 million workers and 1.44 million non-workers. Finally, using information on building use types and opening hours from digital maps, these people were probabilistically assigned to buildings.
"We found that the typical maximum daily occupancy in commercial buildings is about 20-30 percent of the assumed capacity by building types, and that residential occupancy is highly neighborhood dependent, with some areas experiencing much higher occupancy per unit of floor space, like apartment buildings surrounding universities, than others, like single family homes in affluent areas," said Gonzalez.
The researchers note that the occupancy difference between current assumptions and mobile phone-based estimates arise because the current estimates treat buildings in isolation, whereas their research takes into account that people can visit many buildings.
And when these mobile phone-based occupancy estimates were integrated with a state-of-the-art Urban Building Energy Model (UBEM), developed in the MIT Sustainable Design Lab, to understand their impact on energy use predictions, the researchers found that energy consumption differed as much as 15 percent for residential buildings and 20 percent for commercial buildings when compared to current standard methods.
"This highlights a need for new occupancy-to-load models that can be applied at urban scale to the diverse set of city building types," said Gonzalez.
More information: Edward Barbour et al. Planning for sustainable cities by estimating building occupancy with mobile phones, Nature Communications (2019). DOI: 10.1038/s41467-019-11685-w