Team studies calibrated AI and deep learning models to more reliably diagnose and treat disease
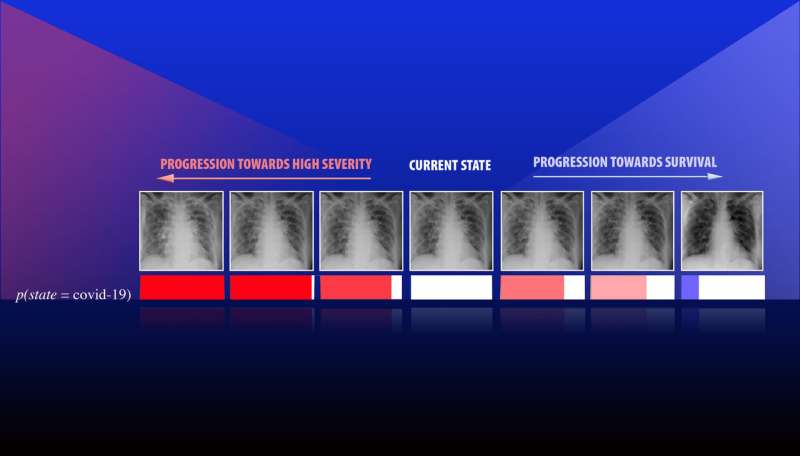
As artificial intelligence (AI) becomes increasingly used for critical applications such as diagnosing and treating diseases, predictions and results regarding medical care that practitioners and patients can trust will require more reliable deep learning models.
In a recent preprint (available through Cornell University's open access website arXiv), a team led by a Lawrence Livermore National Laboratory (LLNL) computer scientist proposes a novel deep learning approach aimed at improving the reliability of classifier models designed for predicting disease types from diagnostic images, with an additional goal of enabling interpretability by a medical expert without sacrificing accuracy. The approach uses a concept called confidence calibration, which systematically adjusts the model's predictions to match the human expert's expectations in the real world.
"Reliability is an important yardstick as AI becomes more commonly used in high-risk applications, where there are real adverse consequences when something goes wrong," explained lead author and LLNL computational scientist Jay Thiagarajan. "You need a systematic indication of how reliable the model can be in the real setting it will be applied in. If something as simple as changing the diversity of the population can break your system, you need to know that, rather than deploy it and then find out."
In practice, quantifying the reliability of machine-learned models is challenging, so the researchers introduced the "reliability plot," which includes experts in the inference loop to reveal the trade-off between model autonomy and accuracy. By allowing a model to defer from making predictions when its confidence is low, it enables a holistic evaluation of how reliable the model is, Thiagarajan explained.
In the paper, the researchers considered dermoscopy images of lesions used for skin cancer screening—each image associated with a specific disease state: melanoma, melanocytic nevus, basal cell carcinoma, actinic keratosis, benign keratosis, dermatofibroma and vascular lesions. Using conventional metrics and reliability plots, the researchers showed that calibration-driven learning produces more accurate and reliable detectors when compared to existing deep learning solutions. They achieved 80 percent accuracy on this challenging benchmark, in contrast to 74 percent by standard neural networks.
However, more important than increased accuracy, prediction calibration provides a completely new way to build interpretability tools in scientific problems, Thiagarajan said. The team developed an introspection approach, where the user inputs a hypothesis about the patient (such as the onset of a certain disease) and the model returns counterfactual evidence that maximally agrees with the hypothesis. Using this "what-if" analysis, they were able to identify complex relationships between disparate classes of data and shed light on strengths and weaknesses of the model that would not otherwise be apparent.
"We were exploring how to make a tool that can potentially support more sophisticated reasoning or inferencing," Thiagarajan said. "These AI models systematically provide ways to gain new insights by placing your hypothesis in a prediction space. The question is, 'How should the image look if a person has been diagnosed with a condition A versus condition B?' Our method can provide the most plausible or meaningful evidence for that hypothesis. We can even obtain a continuous transition of a patient from state A to state B, where the expert or a doctor defines what those states are."
Recently, Thiagarajan applied these methods to study chest X-ray images of patients diagnosed with COVID-19, arising due to the novel SARS-CoV-2 coronavirus. To understand the role of factors such as demography, smoking habits and medical intervention on health, Thiagarajan explained that AI models must analyze much more data than humans can handle, and the results need to be interpretable by medical professionals to be useful. Interpretability and introspection techniques will not only make models more powerful, he said, but they could provide an entirely novel way to create models for health care applications, enabling physicians to form new hypotheses about disease and aiding policymakers in decision-making that affects public health, such as with the ongoing COVID-19 pandemic.
"People want to integrate these AI models into scientific discovery," Thiagarajan said. "When a new infection comes like COVID, doctors are looking for evidence to learn more about this novel virus. A systematic scientific study is always useful, but these data-driven approaches that we produce can significantly complement the analysis that experts can do to learn about these kinds of diseases. Machine learning can be applied far beyond just making predictions, and this tool enables that in a very clever way."
More information: Calibrating Healthcare AI: Towards Reliable and Interpretable Deep Predictive Models: arXiv:2004.14480 [cs.LG] arxiv.org/abs/2004.14480