Technology for cloud efficiency for databases during data-intensive COVID-19 pandemic
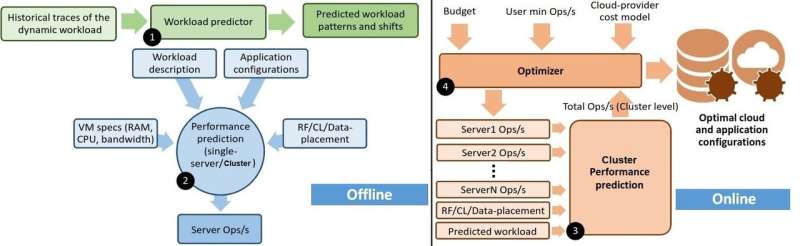
A Purdue University data science and machine learning innovator wants to help organizations and users get the most for their money when it comes to cloud-based databases. Her same technology may help self-driving vehicles operate more safely on the road when latency is the primary concern.
Somali Chaterji, a Purdue assistant professor of agricultural and biological engineering who directs the Innovatory for Cells and Neural Machines [ICAN], and her team created a technology called OPTIMUSCLOUD.
The system is designed to help achieve cost and performance efficiency for cloud-hosted databases, rightsizing resources to benefit both the cloud vendors who do not have to aggressively over-provision their cloud-hosted servers for fail-safe operations and to the clients because the data center savings can be passed on them.
"It also may help researchers who are crunching their research data on remote data centers, compounded by the remote working conditions during the pandemic, where throughput is the priority," Chaterji said. "This technology originated from a desire to increase the throughput of data pipelines to crunch microbiome or metagenomics data."
The Purdue technology works with the three major cloud database providers: Amazon's AWS, Google Cloud, and Microsoft Azure. Chaterji said it would work with other more specialized cloud providers such as Digital Ocean and FloydHub, with some engineering effort.
It is benchmarked on Amazon's AWS cloud computing services with the NoSQL technologies Apache Cassandra and Redis.
"Let's help you get the most bang for your buck by optimizing how you use databases, whether on-premise or cloud-hosted," Chaterji said. "It is no longer just about computational heavy lifting, but about efficient computation where you use what you need and pay for what you use."
Chaterji said current cloud technologies using automated decision making often only work for short and repeat tasks and workloads. She said her team created an optimal configuration to handle long-running, dynamic workloads, whether it be workloads from the ubiquitous sensor networks in connected farms or high-performance computing workloads from scientific applications or the current COVID-19 simulations from different parts of the world in a rush to find the cure against the virus.
"Our right-sizing approach is increasingly important with the myriad applications running on the cloud with the diversity of the data and the algorithms required to draw insights from the data and the consequent need to have heterogeneous servers that drastically vary in costs to analyze the data flows," Chaterji said. "The prices for on-demand instances on Amazon EC2 vary by more than a factor of five-thousand, depending on the virtual memory instance type you use."
The Purdue team's technology has been accepted for publication at the 2020 USENIX Annual Technical Conference, taking place as a virtual event in July.
Chaterji said OPTIMUSCLOUD has numerous applications for databases used in self-driving vehicles (where latency is a priority), health care repositories (where throughput is a priority), and Internet of Things (IoT) infrastructures in farms or factories.
OPTIMUSCLOUD is a software that is run with the database server. It uses machine learning and data science principles to develop algorithms that help jointly optimize the virtual machine selection and the database management system options.
"Also, in these strange times when both traditionally compute-intensive laboratories such as ours and wet labs are relying on compute storage, such as to run simulations on the spread of COVID-19, throughput of these cloud-hosted VMs is critical and even a slight improvement in utilization can result in huge gains," Chaterji said. "Consider that currently, even the best data centers run at lower than 50% utilization and so the costs that are passed down to end-users are hugely inflated."
The other members of the team that developed OPTIMUSCLOUD are Saurabh Bagchi, a Purdue professor of electrical and computer engineering and computer science (by courtesy); Ashraf Mahgoub, a Ph.D. student in computer science; and Karthik Shankar, an undergraduate researcher in Chaterji's lab headed to Carnegie Mellon for graduate school in computer science.
"Our system takes a look at the hundreds of options available and determines the best one normalized by the dollar cost," Chaterji said. "When it comes to cloud databases and computations, you don't want to buy the whole car when you only need a tire, especially now when every lab needs a tire to cruise."