Scientists use artificial intelligence in new way to strengthen power grid resiliency
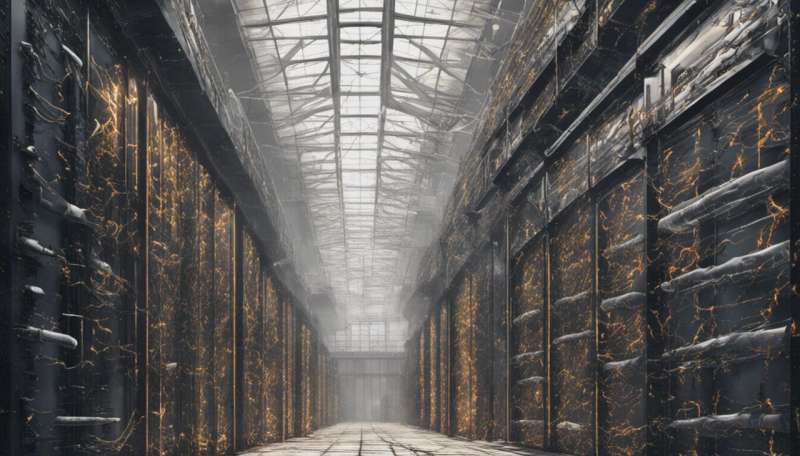
A new artificial neural network model created by Argonne scientists handles both static and dynamic features of a power system with a relatively high degree of accuracy.
America's power grid system is not only large but dynamic, which makes it especially challenging to manage. Human operators know how to maintain systems when conditions are static. But when conditions change quickly, due to sudden faults for example, operators lack a clear way of anticipating how the system should best adapt to meet system security and safety requirements.
At the U.S. Department of Energy's (DOE) Argonne National Laboratory a research team has developed a novel approach to help system operators understand how to better control power systems with the help of artificial intelligence. Their new approach could help operators control power systems in a more effective way, which could enhance the resilience of America's power grid, according to a recent article in IEEE Transactions on Power Systems.
Converging dynamic and static calculations
The new approach allows operators to make decisions considering both static and dynamic features of a power system in a single decision-making model with better accuracy—a historically tough challenge.
"The decision to turn a generator off or on and determine its power output level is an example of a static decision, an action that does not change within a certain amount of time. Electrical frequency, though—which is related to the speed of a generator—is an example of a dynamic feature, because it could fluctuate over time in case of a disruption (e.g., a load tripped) or an operation (e.g., a switch closed)," said Argonne computational scientist Feng Qiu, who co-authored the study. "If you put dynamic and static formulations together in the same model, it's essentially impossible to solve."
In power systems, operators must hold frequency within a certain range of values to meet safety limits. Static conditions, such as the number of generators online, affect system ability of holding frequency and other dynamic features.
Most analysts calculate static and dynamic features separately, but the results fall short. Meanwhile, others have tried to develop simple models that can bridge both types of calculations, but these models are limited in their scalability and accuracy, particularly as systems become more complex.
Artificial neural networks connect the dots between static and dynamic features
Rather than trying to fit existing static and dynamic formulas together, Qiu and his peers developed an approach for creating new formulas that could bridge the two. Their approach centers on using an artificial intelligence tool known as a neural network.
"A neural network can create a map between a specific input and a specific output," said Yichen Zhang, Argonne postdoctoral appointee and lead author of the study. "If I know the conditions we start with and those we end with, I can use neural networks to figure out how those conditions map to each other."
While their neural network approach can apply to bulk-power systems, the team tested it on a microgrid system, a controllable network of distributed energy resources, such as diesel generators and solar photovoltaic panels.
The team used the neural network to track how a set of static conditions within the microgrid system mapped to a set of dynamic conditions or values. More specifically, researchers used it to optimize the static resources within their microgrid so the electrical frequency stayed within a safe range.
Simulation data served as the inputs and outputs for training their neural network. The inputs were static data and outputs were dynamic responses, specifically the range of frequencies that are safe. When the researchers passed both sets of data into the neural network, it "learned" to map estimated dynamic responses for a set of static conditions.
"The neural network transformed the complex dynamic equations that we typically cannot combine with static equations into a new form that we can solve together," Qui said.
Opening doors for new types of analyses
Researchers, analysts and operators can use the Argonne scientists' approach as a starting point. For example, operators could potentially use it to anticipate when they can turn on and off generation resources, while at the same time ensuring that all the resources that are online are able to withstand certain disruptions.
"This is the kind of scenario that system operators have always wanted to analyze, but were unable before to because of the challenges of calculating static and dynamic features together," said Argonne postdoctoral appointee and co-author Tianqi Hong. "Now we think this work makes this type of analysis possible."
"We're excited by the potential for this type of analytical approach," said Mark Petri, Argonne's Electric Power Grid Program director. "For instance, this could provide a better way for operators to quickly and safely restore power after an outage, a problem challenged by complex operational decisions entangled with system dynamics, making the electric grid more resilient to external hazards."
More information: Yichen Zhang et al. Approximating Trajectory Constraints with Machine Learning Microgrid Islanding with Frequency Constraints, IEEE Transactions on Power Systems (2020). DOI: 10.1109/TPWRS.2020.3015913