Team shows how Turing-like patterns fool neural networks
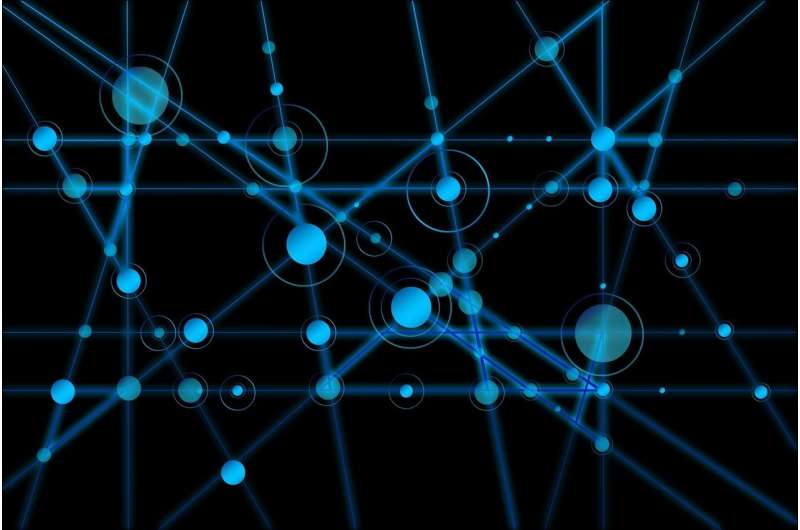
Skoltech researchers were able to show that patterns that can cause neural networks to make mistakes in recognizing images are, in effect, akin to Turing patterns found all over the natural world. In the future, this result can be used to design defenses for pattern recognition systems currently vulnerable to attacks. The paper, available as an arXiv preprint, was presented at the 35th AAAI Conference on Artificial Intelligence (AAAI-21).
Deep neural networks, smart and adept at image recognition and classification as they already are, can still be vulnerable to what's called adversarial perturbations: small but peculiar details in an image that cause errors in neural network output. Some of them are universal: that is, they interfere with the neural network when placed on any input.
These perturbations can represent a significant security risk: for instance, in 2018, one team published a preprint describing a way to trick self-driving vehicles into "seeing" benign ads and logos on them as road signs. The fact that most known defenses a network can have against such an attack can be easily circumvented exacerbates this problem.
Professor Ivan Oseledets, who leads the Skoltech Computational Intelligence Lab at the Center for Computational and Data-Intensive Science and Engineering (CDISE), and his colleagues further explored a theory that connects these universal adversarial perturbations (UAPs) and classical Turing patterns, first described by the outstanding English mathematician Alan Turing as the driving mechanism behind a lot of patterns in nature, such as stripes and spots on animals.
The research started serendipitously when Oseledets and Valentin Khrulkov presented a paper on generating UAPs at the Conference on Computer Vision and Pattern Recognition in 2018. "A stranger came by and told us that this patterns look like Turing patterns. This similarity was a mystery for several years, until Skoltech master students Nurislam Tursynbek, Maria Sindeeva and Ph.D. student Ilya Vilkoviskiy formed a team that was able to solve this puzzle. This is also a perfect example of internal collaboration at Skoltech, between the Center for Advanced Studies and Center for Data-Intensive Science and Engineering," Oseledets says.
The nature and roots of adversarial perturbations are still mysterious for researchers. "This intriguing property has a long history of cat-and-mouse games between attacks and defenses. One of the reasons why adversarial attacks are hard to defend against is lack of theory. Our work makes a step towards explaining the fascinating properties of UAPs by Turing patterns, which have solid theory behind them. This will help construct a theory of adversarial examples in the future," Oseledets notes.
There is prior research showing that natural Turing patterns—say, stripes on a fish—can fool a neural network, and the team was able to show this connection in a straightforward way and provide ways of generating new attacks. "The simplest setting to make models robust based on such patterns is to merely add them to images and train the network on perturbed images," the researcher adds.
More information: Chawin Sitawarin et al, Rogue Signs: Deceiving Traffic Sign Recognition with Malicious Ads and Logos, arXiv:1801.02780v3 [cs.CR], arxiv.org/abs/1801.02780