October 14, 2021 feature
A new model to enable multi-object tracking in unmanned aerial systems
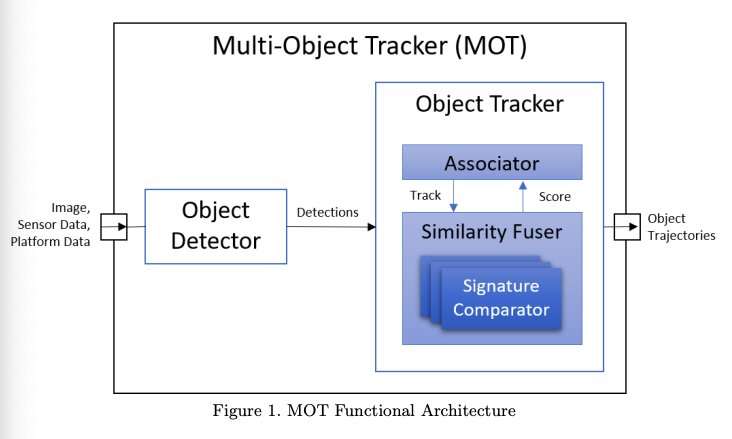
To efficiently navigate their surrounding environments and complete missions, unmanned aerial systems (UASs) should be able to detect multiple objects in their surroundings and track their movements over time. So far, however, enabling multi-object tracking in unmanned aerial vehicles has proved to be fairly challenging.
Researchers at Lockheed Martin AI Center have recently developed a new deep learning technique that could allow UASs to track multiple objects in their surroundings. Their technique, presented in a paper pre-published on arXiv, could aid the development of better performing and more responsive autonomous flying systems.
"We present a robust object tracking architecture aimed to accommodate for the noise in real-time situations," the researchers wrote in their paper. "We propose a kinematic prediction model, called deep extended Kalman filter (DeepEKF), in which a sequence-to-sequence architecture is used to predict entity trajectories in latent space."
The kinematic prediction model created by Wanlin Xie, Jaime Ide and their colleagues at Lockheed Martin AI Center essentially uses an acquired image embedding and a computational attention mechanism to weigh the 'importance' of different parts of an image for predicting changes and future states. Subsequently, the model utilizes similarity measures to calculate distances between objects, by analyzing images using a convolutional neural network (CNN) encoder, pre-trained using Siamese neural networks.
A siamese neural network is an AI technique in which two identical neural networks generate feature vectors for each individual data input and compare these vectors. These approaches can be particularly useful in situations where researchers are trying to detect anomalies or differences in images, as well as for face and object recognition applications.
The researchers evaluated their deep learning technique using annotated video footage collected by a camera integrated on a fixed-wing UAS. These labeled video sequences contained a series of moving objects, including people and vehicles.
"We wanted to precisely diagnose how well our model can accurately and consistently keep track of distinct object entities over continuous periods of time," the researchers wrote in their paper. "We look at several performance measures including absence prediction, prediction recall plots, longevity of tracking, etc."
A Kalman filter (KF) is an algorithm that can estimate some unknown variables, when it is fed a series of measurements collected over time. The multi-object tracking approach proposed by the researchers is a more advanced version of a KF, which also integrates deep learning techniques.
In initial evaluations, the DeepEKF architecture developed by Xie, Ide and their colleagues achieved remarkable results, outperforming standard KF algorithms for multi-object tracking. In the future, their framework could thus be used to enhance the capabilities of a variety of UASs.
"Although we report proof of concept results, further training of the DeepEKF as well as of the Siamese networks are necessary as we collect more data," the researchers wrote in their paper. "In particular, we plan to add a more extensive evaluation for the long-term tracking (re-identification) component. Another promising venue is to dynamically combine the different kinematic and visual scores within the similarity fuser component given the environment and track states."
More information: Multi-object tracking with deep learning ensemble for unmanned aerial system applications. arXiv:2110.02044 [cs.CV]. arxiv.org/abs/2110.02044
© 2021 Science X Network