Machine learning method could speed the search for new battery materials
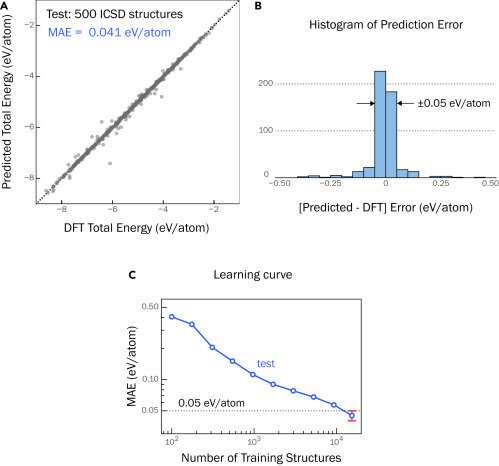
To discover materials for better batteries, researchers must wade through a vast field of candidates. New research demonstrates a machine learning technique that could more quickly surface ones with the most desirable properties.
The study could accelerate designs for solid-state batteries, a promising next-generation technology that has the potential to store more energy than lithium-ion batteries without the flammability concerns. However, solid-state batteries encounter problems when materials within the cell interact with each other in ways that degrade performance.
Researchers from the National Renewable Energy Laboratory (NREL), the Colorado School of Mines, and the University of Illinois demonstrated a machine learning method that can accurately predict the properties of inorganic compounds. The work is led by NREL and part of DIFFERENTIATE, an initiative funded by the U.S. Department of Energy's Advanced Research Projects Agency–Energy (ARPA-E) that aims to speed energy innovation by incorporating artificial intelligence.
The compounds of interest are crystalline solids with atoms arranged in repeating, three-dimensional patterns. One way to measure the stability of these crystal structures is by calculating their total energy—lower total energy translates to higher stability. A single compound can have many different crystal structures. To find the one with the lowest energy—the ground-state structure—researchers rely on computationally expensive, high-fidelity numerical simulations.
Solid-state batteries lose capacity and voltage if competing phases form at the interface between the electrode and the electrolyte. Finding pairs of materials that are compatible requires researchers to ensure that the materials will not decompose. But the field of candidates is wide: Estimates suggest there are millions or even billions of plausible solid-state compounds waiting to be discovered.
"You can't do these very detailed simulations on a huge swath of this potential crystal structure space," said Peter St. John, an NREL researcher and lead principal investigator of the ARPA-E project. "Each one is a very intensive calculation that takes minutes to hours on a big computer." Humans must then comb through the resulting data to manually identify new prospective materials.
To accelerate the process, the researchers used a form of machine learning called a graph neural network. A graph neural network is an algorithm that can be trained to detect and highlight patterns in data. Here, the "graph" is essentially a map of each crystal structure. The algorithm analyzes each crystal structure and then predicts its total energy.
However, the success of any neural network will depend on the data it uses to learn. Scientists have already identified more than 200,000 inorganic crystal structures, but there are many, many more possibilities. Some crystal structures look stable at first—until comparison to a lower-energy compound reveals otherwise. The researchers came up with hypothetical, higher-energy crystals that could help hone the machine learning model's ability to distinguish between structures that simply appear stable and ones that actually are.
"To train a model that can correctly predict whether a structure is stable or not, you can't just feed it the ground-state structures that we already know about. You have to give it these hypothetical higher-energy structures so that the model can distinguish between the two," St. John said.
To train their graph neural network, researchers created theoretical examples based not on nature but on quantum mechanical calculations. By including both ground-state and high-energy crystals in the training data, the researchers were able to get far more accurate results compared with a model trained only on ground-state structures. The researchers' model had five times lower average error than the comparison case.
The study, "Predicting energy and stability of known and hypothetical crystals using graph neural network," was published in the journal Patterns on November 12. Co-authors with St. John are Prashun Gorai, Shubham Pandey, and Vladan Stevanović of the Colorado School of Mines, and Jiaxing Qu of the University of Illinois. The researchers used NREL's Eagle high-performance computing system to run their calculations.
The approach could revolutionize the speed with which researchers can discover new materials with valuable properties, allowing them to quickly surface the most promising crystal structures. The work is broadly relevant, said Gorai, a research professor at the Colorado School of Mines, who holds a joint appointment at NREL.
"The scenario where two solids come into contact with each other occurs in many different applications—photovoltaics, thermoelectrics, all sorts of functional devices," Gorai said. "Once the model is successful, it can be deployed for many applications beyond solid-state batteries."
More information: Shubham Pandey et al, Predicting energy and stability of known and hypothetical crystals using graph neural network, Patterns (2021). DOI: 10.1016/j.patter.2021.100361