Report: There's more to AI bias than biased data
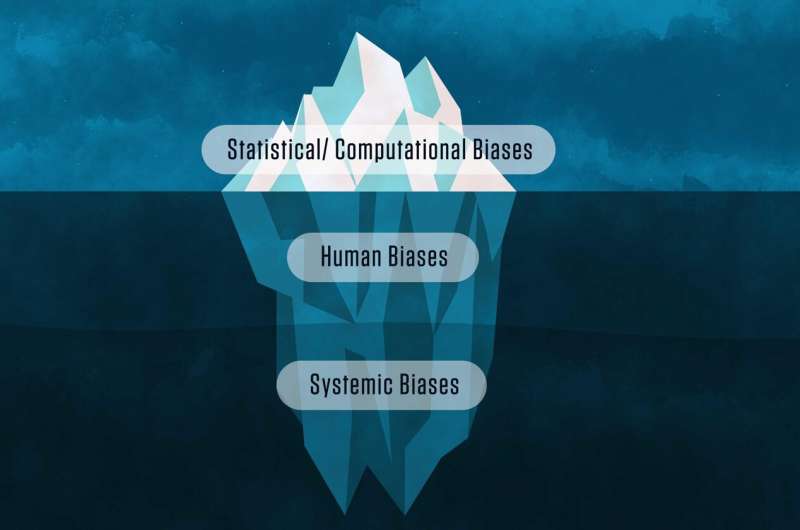
As a step toward improving our ability to identify and manage the harmful effects of bias in artificial intelligence (AI) systems, researchers at the National Institute of Standards and Technology (NIST) recommend widening the scope of where we look for the source of these biases—beyond the machine learning processes and data used to train AI software to the broader societal factors that influence how technology is developed.
The recommendation is a core message of a revised NIST publication, "Towards a Standard for Identifying and Managing Bias in Artificial Intelligence," which reflects public comments the agency received on its draft version released last summer. As part of a larger effort to support the development of trustworthy and responsible AI, the document offers guidance connected to the AI Risk Management Framework that NIST is developing.
According to NIST's Reva Schwartz, the main distinction between the draft and final versions of the publication is the new emphasis on how bias manifests itself not only in AI algorithms and the data used to train them, but also in the societal context in which AI systems are used.
"Context is everything," said Schwartz, principal investigator for AI bias and one of the report's authors. "AI systems do not operate in isolation. They help people make decisions that directly affect other people's lives. If we are to develop trustworthy AI systems, we need to consider all the factors that can chip away at the public's trust in AI. Many of these factors go beyond the technology itself to the impacts of the technology, and the comments we received from a wide range of people and organizations emphasized this point."
Bias in AI can harm humans. AI can make decisions that affect whether a person is admitted into a school, authorized for a bank loan or accepted as a rental applicant. It is relatively common knowledge that AI systems can exhibit biases that stem from their programming and data sources; for example, machine learning software could be trained on a dataset that underrepresents a particular gender or ethnic group. The revised NIST publication acknowledges that while these computational and statistical sources of bias remain highly important, they do not represent the full picture.
A more complete understanding of bias must take into account human and systemic biases, which figure significantly in the new version. Systemic biases result from institutions operating in ways that disadvantage certain social groups, such as discriminating against individuals based on their race. Human biases can relate to how people use data to fill in missing information, such as a person's neighborhood of residence influencing how likely authorities would consider the person to be a crime suspect. When human, systemic and computational biases combine, they can form a pernicious mixture—especially when explicit guidance is lacking for addressing the risks associated with using AI systems.
To address these issues, the NIST authors make the case for a "socio-technical" approach to mitigating bias in AI. This approach involves a recognition that AI operates in a larger social context—and that purely technically based efforts to solve the problem of bias will come up short.
"Organizations often default to overly technical solutions for AI bias issues," Schwartz said. "But these approaches do not adequately capture the societal impact of AI systems. The expansion of AI into many aspects of public life requires extending our view to consider AI within the larger social system in which it operates."
Socio-technical approaches in AI are an emerging area, Schwartz said, and identifying measurement techniques to take these factors into consideration will require a broad set of disciplines and stakeholders.
"It's important to bring in experts from various fields—not just engineering—and to listen to other organizations and communities about the impact of AI," she said.
More information: Reva Schwartz et al, Towards a Standard for Identifying and Managing Bias in Artificial Intelligence, (2022). DOI: 10.6028/NIST.SP.1270
This story is republished courtesy of NIST. Read the original story here.