Detecting distributed denial of service attacks
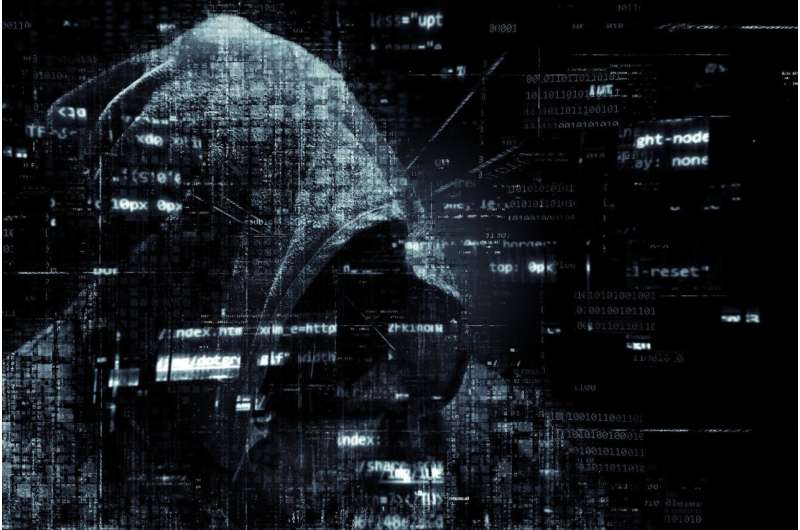
The distributed denial-of-service (DDOS) attack may well be familiar to anyone who has spent time running online services, such as websites. It is a malicious attack on the servers running the system that simply bombards the computers with requests that overwhelm it and prevent legitimate users from accessing the resources.
The DDOS attack is used by malicious third parties for various reasons. The aim may well be to simply block any legitimate use and may be done to sabotage a company, organization, or government in some way. The DDOS attack might also be used by third parties hoping to gain access to normally hidden information. The attack and the organization's response to it, can often allow breaches of firewalls and other security measures allowing those third parties to steal information from the servers or even take control of the systems.
DDOS attacks can be carried out by mass coordinated activity of individuals running computers with that intention. They can also be carried out by a malicious third party with control of a botnet (a network of hijacked computers). New work in the International Journal of Networking and Virtual Organizations is using deep learning to detect DDOS attacks and so allow service providers to ameliorate their effects.
Hanene Mennour and Sihem Mostefai of the University Abdelhamid Mehri in Constantine, Algeria, explain that DDOS are unremitting and given the current state of world affairs, there is an increasingly pressing need to find ways to detect and block these attacks. The researchers have built and tested a built a deep convolutional neural network (CNN), a stacked long short-term memory (S-LSTM) neural network which they explain is a distinct artificial recurrent neural network (RNN), and a third system that is a hybrid of the CNN and the LSTM systems.
The team tested against three benchmarking tools—CICIDS2017, CICDDoS2019, and BoT-IoT. They found, perhaps not surprisingly, that the scalable hybrid tool was the most effective in detecting a DDOS attack than either of the separate modules. Moreover, comparison with other approaches shows that this novel tool has lower computational complexity and can also outperform earlier approaches in almost all metrics.
More information: Hanene Mennour, Sihem Mostefai, Deep learning-based distributed denial-of-service detection, International Journal of Networking and Virtual Organisations (2022). DOI: 10.1504/IJNVO.2022.10045916