February 10, 2023 feature
This article has been reviewed according to Science X's editorial process and policies. Editors have highlighted the following attributes while ensuring the content's credibility:
fact-checked
peer-reviewed publication
trusted source
proofread
A deep reinforcement learning model that allows AI agents to track odor plumes
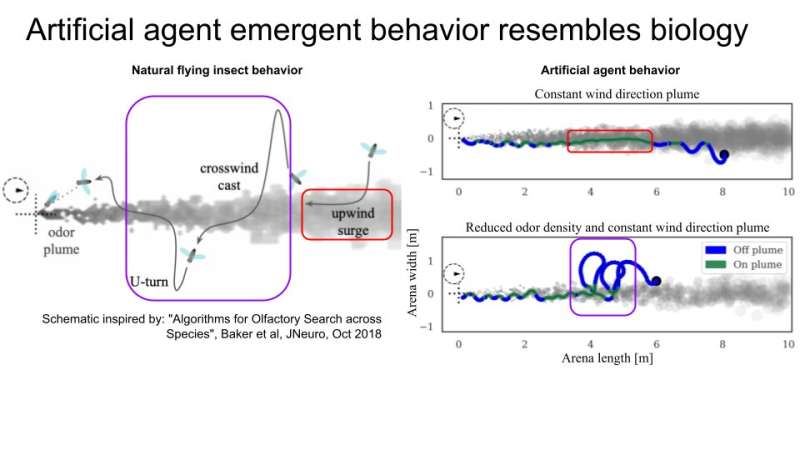
For a long time, scientists and engineers have drawn inspiration from the amazing abilities of animals and have sought to reverse engineer or reproduce these in robots and artificial intelligence (AI) agents. One of these behaviors is odor plume tracking, which is the ability of some animals, particularly insects, to home in on the source of specific odors of interest (e.g., food or mates), often over long distances.
A new study by researchers at University of Washington and University of Nevada, Reno has taken an innovative approach using artificial neural networks (ANNs) in understanding this remarkable ability of flying insects. Their work, recently published in Nature Machine Intelligence, exemplifies how artificial intelligence is driving groundbreaking new scientific insights.
"We were motivated to study a complex biological behavior, odor plume-tracking, that flying insects (and other animals) use to find food or mates," Satpreet H. Singh, the lead author on the study, told Tech Xplore. "Biologists have experimentally studied many aspects of insect plume tracking in great detail, as it is a critical behavior for insect survival and reproduction. "
While plume tracking is a crucial biological ability, it is also a remarkable example of biological intelligence, as it entails the integration of memories about current and previously experienced odors, as well as the processing of intermittent or unreliable olfactory cues and wind sensory signals to allowing the insects to rapidly adapt their flight trajectories.
"And they do this without having a global map of the environment they are flying within," Singh added.
If reliably reproduced in robots or artificial agents, odor plume tracking could allow researchers to make better robots that can detect and track harmful gas leaks, wildfires, and other environmental threats.
"Instead of running a traditional laboratory wind-tunnel experiment, we used a complementary 'in-silico' approach using artificial neural networks," Singh explained. "This helped us develop an integrative understanding of plume tracking across multiple levels, including emergent behavior, neural representation and neural dynamics."
Many neuroscientists have started using artificial neural networks (ANNs) trained on labeled data to study and artificially replicate biological processes. In their study, Singh and his colleagues instead used deep-reinforcement learning (DRL), an algorithmic toolkit that has only begun to gain traction in neuroscience, that uses simulations rather than labeled data to train ANNs.
"Some highly successful recent applications of DRL outside of neuroscience include DeepMind's famous Go playing AI, or some of OpenAI's recent GPT language models," Singh said. "Like in animal training, DRL uses simulated 'rewards' and 'punishments' to train ANNs agents that can autonomously complete tasks."
To train their plume-tracking agents using DRL, the researchers first simulated an odor emanating from a source located within a windy arena with a total area of approximately 120 m2. When their agents identified where the source of the odor was located, they received a reward. In contrast, if they lost track of the odor plume and left the arena, they were "punished."
"After training, we took advantage of the flexibility of our simulator to generate plumes with a variety of odor concentrations and wind patterns, to see how the agent precisely behaves under varying conditions," Singh said. "Reproducing such fine-grained control over plume configurations in a real wind-tunnel would be a rather laborious effort."
Singh and his colleagues were also able to observe the activity of their artificial neural network's individual units (i.e., artificial neurons) as it tracked odor plumes. Such individual neuron recordings during tracking have not yet been collected in insects during free-form flight, as they are unattainable using existing technologies.
"The behavior that emerges in our trained artificial agents bears a striking resemblance to the behavior modules biologists have previously observed in flying insects performing plume tracking," Singh said.
The recordings gathered by the researchers suggest that their model might replicate the biological processes underpinning odor plume tracking in animals. Subsequently, Singh and his colleagues simulated plume configurations that could be recreated in future wind tunnel real-world experiments.
These simulations allowed them to generate different hypotheses of how artificial agents might behave when tracking plumes in changing wind conditions. They specifically looked at instances in which the direction of the wind is frequently changing.
"Using simultaneous behavioral and neural observations from our ANN agents, we developed intuitions and insight into the algorithms and neural computations that support plume tracking," Singh explained. "As an example, we see that the neural activity encodes variables like the time-since-the-last-odor-encounter, which were previously hypothesized to be important to plume tracking. These similarities between previous experimental and computational results suggest the fundamental importance of these quantities for successful plume tracking."
In addition to enabling key technological advancements, ANN agents can be reverse engineered to better understand how they work, which could in turn potentially inform neuroscience research. The model created by Singh and his colleagues could thus potentially also be used by neuroscientists to study the biological processes behind odor plume tracking.
In the future, the researchers hope that their model will inspire the creation of robotic agents that can track odors during search-and-rescue missions, environmental monitoring efforts, and other applications. In their next studies, they plan to develop their model further, by improving the physical and biological fidelity of their simulations and agents so that they better represent real-world odor plumes. In addition, they hope to artificially reproduce other physiological characteristics and capabilities of flying insects.
"More theoretical work will also be required to understand our artificial neural networks, to further understand the computations that produce the emergent behavior," Singh added. "Finally, our agents perform a single task, plume tracking, while flying insects have a much richer behavioral repertoire. Developing the simulations and agent-training paradigms that could reproduce such rich biological complexity is a formidable engineering challenge that should inspire future work."
More information: Satpreet H. Singh et al, Emergent behaviour and neural dynamics in artificial agents tracking odour plumes, Nature Machine Intelligence (2023). DOI: 10.1038/s42256-022-00599-w
© 2023 Science X Network