This article has been reviewed according to Science X's editorial process and policies. Editors have highlighted the following attributes while ensuring the content's credibility:
fact-checked
peer-reviewed publication
trusted source
proofread
Deep-learning tool boosts X-ray imaging resolution and hydrogen fuel cell performance
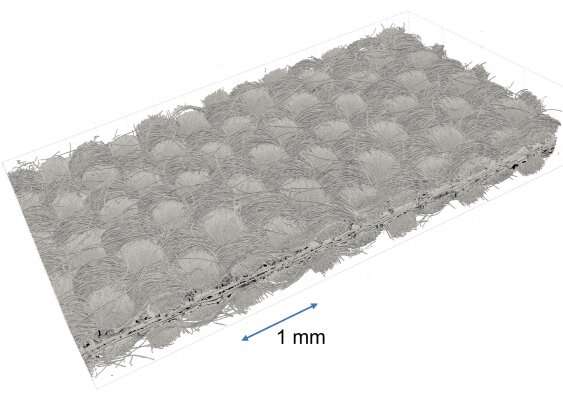
Researchers from UNSW Sydney have developed an algorithm that produces high-resolution modeled images from lower-resolution micro X-ray computerized tomography (CT).
The new process, detailed in a paper published in Nature Communications, has been tested on individual hydrogen fuel cells to accurately model the interior in precise detail and potentially improve their efficiency.
But the researchers say it could also be used in the future on human X-rays to give medical professionals a better understanding of tiny cellular structures inside the body, which could allow for better and faster diagnosis of a wide range of diseases.
The team, featuring Professor Ryan Armstrong, Professor Peyman Mostaghimi, Dr. Ying Da Wang, and Kunning Tang from the School of Mineral and Energy Resources Engineering and Prof Chuan Zhao and Dr. Quentin Meyer from the School of Chemistry, developed the algorithm to improve the understanding of what is happening inside a Proton Exchange Membrane Fuel Cell (PEMFC).
PEMFCs use hydrogen fuel to generate electricity and are a quiet, and clean energy source that can power homes, vehicles, and industries.
These fuel cells convert the hydrogen, via an electrochemical process, into electricity with the only by-product of the reaction being pure water.
However, the PEMFCs can become inefficient if the water cannot properly flow out of the cell and subsequently "floods" the system. Until now, it has been very hard for engineers to understand the precise ways in which water drains, or indeed pools, inside the fuel cells due to their very small size and very complex structures.
Improved resolution
The solution created by the UNSW researchers allows for deep learning to create a detailed 3D model by utilizing a lower-resolution X-ray image of the cell, while extrapolating data from an accompanying high-res scan of a small sub-section of it.
In more basic terms, it's the equivalent of taking a blurry aerial photo of an entire town from an airplane, along with a very detailed photo of just a few streets, and then being able to accurately predict the lay-out of every road in the entire area.
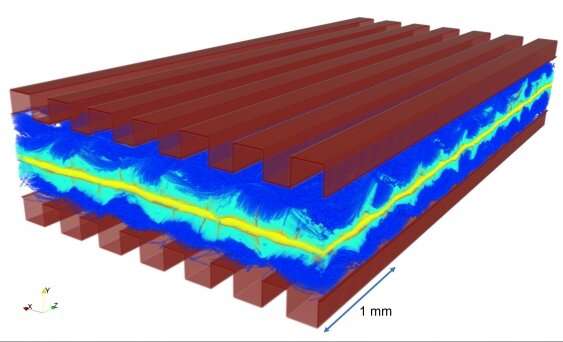
"One of the reasons this research is so novel is that we are pushing the limit of what can be produced from imaging," says Professor Armstrong.
"It is very typical that when you use a piece of hardware, whether it's a microscope or a CT scanner, the resolution of an image gets worse the more you zoom out.
"Our machine learning technique resolves that problem, and the methodology is broadly applicable where any imaging is taking place, such as medical applications, or the oil and gas industry, or chemical engineering.
"We have done preliminary super-resolution work with radiologists previously and we could surmise that by obtaining a higher resolution image from a larger field of view that it may be possible to diagnose diseases, such as tumor cells, earlier, when they are smaller."
DualEDSR algorithm
Dr. Wang says in the published study their super-resolution algorithm, known as DualEDSR, improves the field of view by about 100 times compared to the high-res image.
And he agrees that implementation in medical imaging is an exciting future development.
"If you look at what we are doing now and apply it to the medical field, then it would be very interesting to be able to image blood vessels and the flow of red blood cells through the capillary network in even more detail," he says.
"These beyond-hardware imaging and modeling methods extend beyond fuel cell imaging to enable higher resolution imaging of larger fields of view than previously practical."
One limitation to the modeling process as detailed in the study is the fact the larger-scale low-res image and the smaller-scale higher-res image need to be taken at the same location, by the same machine.
These are known as "Region of Interest" scanners and are specialized pieces of equipment that may not currently be available at many facilities.
However, the team hopes that further research will allow deep learning techniques to produce similar results in future when presented with images that were not taken at the same location and potentially not even using exactly the same instrument or material.
Fast-fueling PEMFCs
For now, the researchers are able to provide a detailed 3D model of the inside of a PEMFC in order for manufacturers to improve the management of the water produced and make the fuel cells more efficient.
During training and testing of DualEDSR, the algorithm achieved 97.3% accuracy when producing high-res modeling from low-res imagery. It also produced a high-resolution model in just 1 hour, compared to the 1,188 hours (the equivalent of 50 days non-stop) it would have taken to obtain high-res images of the whole section of the fuel cell using a micro-CT scanner.
"From our model we can quickly and precisely see where the water tends to accumulate and therefore, we can help to solve those problems in future designs," says Dr. Meyer.
"Within the industry it is known that there is a huge untapped performance improvement that could be made using these cells, just by improved water management, and that is estimated to be a 60% increase overall.
"For the past 20 years, up until now, it has been very hard to have an accurate model of these fuel cells because of the complexity of both the materials, and the way gases and liquids are transported, as well as the electrochemical reactions taking place.
"Our cross-disciplinary team has enabled us to do just that, bringing so many different expertises to the table. That's what research is about."
More efficient PEMFCs could be an important element in providing clean and environmentally-friendly electricity in the future, given the fact they emit only water and heat. In addition, they are compact and lightweight, making them suitable for use in vehicles.
They can also be rapidly refueled, as quickly as refueling a car with petrol, giving them a key advantage over battery-powered EVs which can take many hours even with a rapid charger.
More information: Ying Da Wang et al, Large-scale physically accurate modelling of real proton exchange membrane fuel cell with deep learning, Nature Communications (2023). DOI: 10.1038/s41467-023-35973-8