February 24, 2023 feature
This article has been reviewed according to Science X's editorial process and policies. Editors have highlighted the following attributes while ensuring the content's credibility:
fact-checked
trusted source
proofread
A universal domain adaptation technique for remote sensing image classification
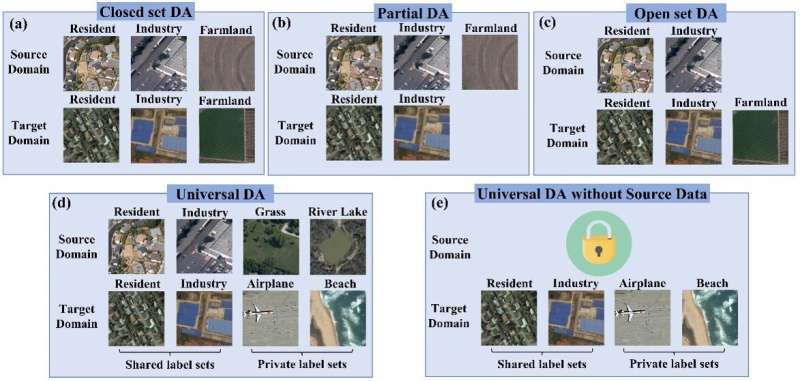
Domain adaptation approaches are techniques designed to improve the performance of computational models in specific target domains. These techniques are particularly valuable for tackling problems for which there is only a limited amount of relevant annotated data and for which training machine learning algorithms is thus particularly challenging.
Researchers at Technical University of Munich (TUM) recently developed a universal domain adaptation (UniDA) approach that could improve the performance of models trained to classify images taken by remote sensors. Their paper, published in IEEE Transactions on Geoscience and Remote Sensing, introduces a UniDA technique for remote sensing image scene classification as well as a new model adaptation (MA) network and source data generation-based model adaptation (SDG-MA) network for UniDA without source data.
"Most existing domain adaptation (DA) approaches tackle the domain gap between different domains based on the knowledge of the relationship between the source and target label space (category gap), such as closed-set DA, partial DA, and open-set DA," Yilei Shi, one of the researchers who carried out the study, told Tech Xplore.
"These approaches are usually not well-suited for practical remote sensing image classification, as they rely on rich prior knowledge about the relationship between source label sets and target domains, and the source data is often not accessible due to privacy or confidentiality issues. To this end, we propose a practical UniDA setting for remote sensing image scene classification that requires no prior knowledge on the label sets."
Shi and his colleagues developed a dual-stage framework that achieves UniDA both in the presence and absence of relevant source data. This framework completes two stages, namely the source data generation and the purpose of model adaptation stage.
During the first of these stages, the framework estimates the conditional distribution of the source data. Based on these estimates, it generates synthetic source images that match both the content and style of images relevant to a given task in cases where the source data is unavailable.
"With this synthetic source data in hand, it becomes a UniDA task to classify a target sample correctly if it belongs to any category in the source label set or mark it as 'unknown' otherwise," Shi explained. "In the second stage, a novel transferable weight that distinguishes the shared and private label sets in each domain promotes the adaptation in the automatically discovered shared label set and recognizes the 'unknown' samples successfully."
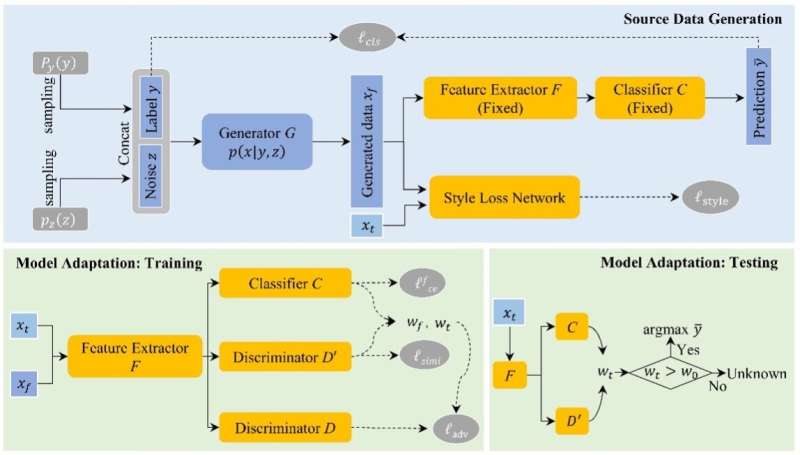
Shi and his colleagues evaluated their UniDA technique in a series of tests. They found that it could effectively improve the performance of models in remote sensing image scene classification tasks, irrespective of whether labeled training data was available or not.
"Our proposed UniDA setting for remote sensing image scene classification is more practical and challenging than other settings, as it includes cases in which no information about the source data distribution and no prior knowledge on the label sets is provided," Shi said. "We reformulated the goal as estimating the conditional distribution rather than the distribution of the source data base on the Bayesian theory, which theoretically guarantees the reliability and efficiency of data generation."
Overall, initial findings suggest that the team's UniDA framework is both effective and practical. Moreover, compared to other existing methods to measure uncertainty, the transferable weight approach proposed by Shi and his colleagues can discriminate uncertainty better, particularly in instances where categorical distributions are relatively uniform.
"Our study can serve as a starting point in a challenging UniDA setting for remote sensing images," Shi said. "Based on this work, we can address two practical problems in the field of remote sensing. First, in a general scenario, we cannot select the proper domain adaptation methods (closed-set DA, partial DA, or open-set DA) because no prior knowledge about the target domain label set is given. Secondly, we can tackle cases in which source datasets are not available."
Today, effectively training computational models on real-world remote sensing tasks can be challenging, as datasets relevant to specific scenarios are scarce. For instance, many satellite companies and users do not share their data due to data privacy and security issues. In other cases, source datasets (e.g., high-resolution remote sensing images) may be so large that transferring them on other platforms becomes inconvenient or unfeasible.
The UniDA framework proposed by this team of researchers could help to improve the performance of models on remote sensing tasks, even in the absence task-specific training datasets. In addition, it could inspire the development of similar DA approaches for other real-world applications for which training data is limited.
"In our experiments, it is difficult for the transferable weight in model adaptation to tune an optimal threshold to apply it to all UniDA tasks of remote sensing images," Shi added. "Thus, in our future studies, we will focus on adaptively learning the threshold through the use of an open-set classifier."
More information: Qingsong Xu et al, Universal Domain Adaptation for Remote Sensing Image Scene Classification, IEEE Transactions on Geoscience and Remote Sensing (2023). DOI: 10.1109/TGRS.2023.3235988
© 2023 Science X Network