This article has been reviewed according to Science X's editorial process and policies. Editors have highlighted the following attributes while ensuring the content's credibility:
fact-checked
peer-reviewed publication
trusted source
proofread
Using artificial intelligence to design innovative materials
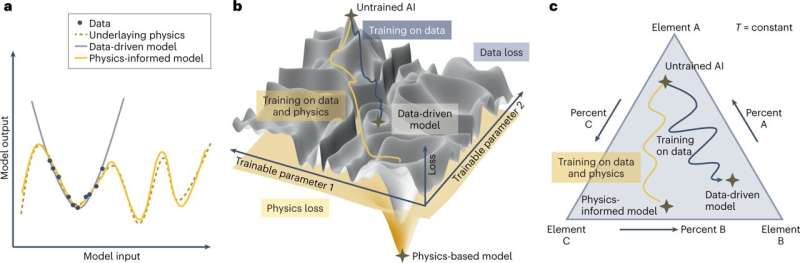
Advanced materials become increasingly complex due to the high requirements they have to fulfill regarding sustainability and applicability. Dierk Raabe and colleagues reviewed the use of artificial intelligence in materials science and the untapped spaces it opens if combined with physics-based simulations. Compared to traditional simulation methods AI has several advantages and will play a crucial role for material sciences in the future.
Advanced materials are urgently needed for everyday life, be it in high technology, mobility, infrastructure, green energy or medicine. However, traditional ways of discovering and exploring new materials encounter limits due to the complexity of chemical compositions, structures and targeted properties. Moreover, new materials should not only enable novel applications, but also include sustainable ways of producing, using and recycling them.
Researchers from the Max-Planck-Institut für Eisenforschung (MPIE) have reviewed the status of physics-based modeling and discuss how combining these approaches with artificial intelligence can open as-yet untapped spaces for the design of complex materials. They have published their perspective in the journal Nature Computational Science.
Combining physics-based approaches with artificial intelligence
To meet the demands of technological and environmental challenges, ever more demanding and multifold material properties have to be considered, which creates alloys more complex in terms of composition, synthesis, processing and recycling. Changes in these parameters entail changes in their microstructure, which directly impacts the material's properties. Computational materials design approaches play a crucial role here.
"Our means of designing new materials rely today exclusively on physics-based simulations and experiments. This approach can experience certain limits when it comes to the quantitative prediction of high-dimensional phase equilibria and particularly to the resulting non-equilibrium microstructures and properties. Moreover, many microstructure- and property-related models use simplified approximations and rely on a large number of variables. However, the question remains if and how these degrees of freedom are still capable of covering the material's complexity," explains Professor Dierk Raabe, director at MPIE and first author of the publication.
The paper compares physics-based simulations, like molecular dynamics and ab initio simulations, with descriptor-based modeling and advanced artificial intelligence approaches. While physics-based simulations are often too costly to predict materials with complex compositions, the use of artificial intelligence (AI) has several advantages.
"AI is capable of automatically extracting thermodynamic and microstructural features from large data sets obtained from electronic, atomistic and continuum simulations with high predictive power," says Professor Jörg Neugebauer, director at MPIE and co-author of the publication.
Enhancing machine learning with large data sets
As the predictive power of artificial intelligence depends on the availability of large data sets, ways of overcoming this obstacle are needed. One possibility is to use active learning cycles, where machine learning models are trained with initially small subsets of labeled data. The model's predictions are then screened by a labeling unit that feeds high quality data back into the pool of labeled records and the machine learning model is run again. This step-by-step approach leads to a final high-quality data set usable for accurate predictions.
There are still many open questions for the use of artificial intelligence in materials science: How to handle sparse and noisy data. How to consider interesting outliers or "misfits." How to implement unwanted elemental intrusion from synthesis or recycling. However, when it comes to designing compositionally complex alloys, artificial intelligence will play a more important role in the near future, especially with the development of algorithms, and the availability of high-quality material datasets and high-performance computing resources.
More information: Dierk Raabe et al, Accelerating the design of compositionally complex materials via physics-informed artificial intelligence, Nature Computational Science (2023). DOI: 10.1038/s43588-023-00412-7