This article has been reviewed according to Science X's editorial process and policies. Editors have highlighted the following attributes while ensuring the content's credibility:
fact-checked
proofread
A new perspective on Petri net learning
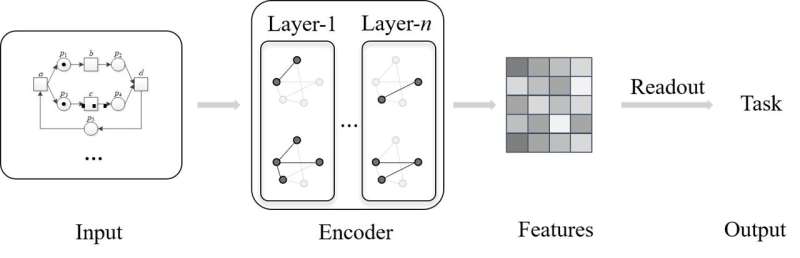
The state space explosion problem means that the state space of Petri nets (PNs) grows exponentially with PNs' size. Even the fundamental reachability problem is still an NP-Hard problem in general. It has been proved that the equivalence problem for the reachability set of arbitrary PNs is undecidable except for some subclass of PNs. That is, the reachability problem of arbitrary PNs cannot be solved exactly. Nowadays, there is no efficient and accurate algorithm to solve the problem.
A research team led by Changjun Jiang published their new research from a data-driven perspective on Nov. 6 in Frontiers of Computer Science.
Inspired by machine learning, the team proposed a method from a data-driven perspective, namely Net Learning. PNs (inputs) and corresponding tasks (actual outputs) are involved as training data. During the training phase, the method encodes the data to obtain hidden information by deep neural networks and further establishes the mapping function (i.e., the model) for the corresponding tasks.
The method will update the parameters of the deep neural networks through backpropagation and adjust the model so that the gap between the predicted and actual output is as tiny as possible. During the prediction phase, the trained model is used to predict unknown data.
Considering the static topology of PNs and their unique dynamic operation mechanism, suitable learning algorithms must be designed to support the quantitative analysis of PNs. Unlike traditional PN analysis methods, the method obtains a probabilistic approximate solution avoiding the state space explosion problem, which is a novel perspective that utilizes correlation relation in the field of machine learning as an approximate alternative to causality in the field of PNs.
Net Learning has injected new vitality into the field of PNs. In many real scenarios, some properties are difficult to reason and prove strictly. Driven by the data related to PNs, Net Learning provides a machine learning perspective to solve the problem, which promotes the development of the field of PNs.
More information: Hongda Qi et al, A perspective on Petri Net learning, Frontiers of Computer Science (2023). DOI: 10.1007/s11704-023-3381-5