This article has been reviewed according to Science X's editorial process and policies. Editors have highlighted the following attributes while ensuring the content's credibility:
fact-checked
trusted source
proofread
Audio explainable artificial intelligence: Demystifying 'black box' models
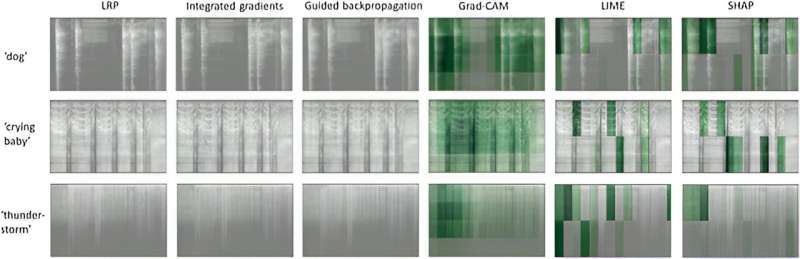
AI decision-making is now common in self-driving cars, patient diagnosis and legal consultation, and it needs to be safe and trustworthy. Researchers have been trying to demystify complex AI models by developing interpretable and transparent models, collectively known as explainable AI methods or explainable AI (XAI) methods. A research team offered their insight specifically into audio XAI models in a review article published in Intelligent Computing.
Although audio tasks are less researched than visual tasks, their expressive power is not less important. Audio signals are easy to understand and communicate, as they typically depend less on expert explanations than visual signals do. Moreover, scenarios like speech recognition and environmental sound classification are inherently audio-specific.
The review categorizes existing audio XAI methods into two groups: general methods applicable to audio models and audio-specific methods.
Using general methods means choosing an appropriate generic model originally built for non-audio tasks and adjusting it to suit a certain audio task. These methods explain audio models through various input representations like spectrograms and waveforms and different output formats like features, examples, and concepts.
Popular general methods include guided backpropagation, which enhances the standard backpropagation process by highlighting the most relevant parts of the input data; LIME, which approximates a complex model with a simpler model; and network dissection, which analyzes the internal representations learned by a neural network.
Audio-specific methods, on the other hand, are specially designed for audio tasks. They aim to decompose audio inputs into meaningful components, focusing on the auditory nature of audio data. Some examples are CoughLIME, which provides sonified explanations for cough sounds in COVID-19 detection, and audioLIME, which uses source separation to explain music tagging models by attributing importance to audio components.
XAI methods can also be categorized by their stage, scope, input data type, and output format. Stage refers to the period where the explanations are generated, whether before, during, or after the training process. Scope determines whether the explanation targets the entire model or a specific input.
XAI usually involves different strategies, such as explaining with predefined rules or specific input examples, highlighting the most important features, focus areas, or input changes, and using simpler models to explain complex ones locally.
The research team identifies several ways audio models could be made more interpretable, such as using raw waveforms or spectrograms to provide listenable explanations and defining higher-level concepts in audio data, which is similar to how superpixels are used in image data. They also believe the expressive power of audio explanations could be extended to non-audio models, and offering a complementary communication channel for vision-based user interactions could be one possibility.
More information: Alican Akman et al, Audio Explainable Artificial Intelligence: A Review, Intelligent Computing (2023). DOI: 10.34133/icomputing.0074