This article has been reviewed according to Science X's editorial process and policies. Editors have highlighted the following attributes while ensuring the content's credibility:
fact-checked
peer-reviewed publication
trusted source
proofread
Lightweight machine learning method enhances scalable structural inference and dynamic prediction accuracy
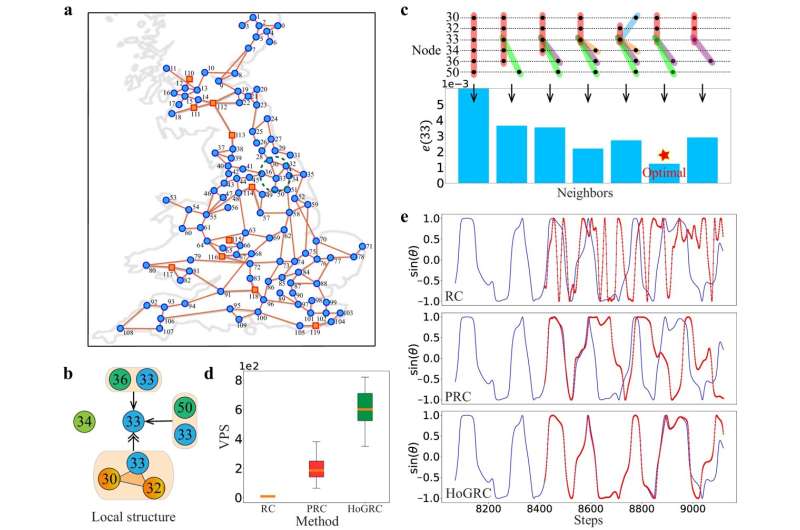
In recent strides within machine learning technology, particularly in reservoir computing (RC), notable advancements have been made in understanding complex systems across various domains. Researchers have been tirelessly innovating machine learning methods to analyze and forecast the dynamic behaviors of intricate systems using observed time series data. However, a pressing challenge persists: how to uphold a lightweight model while harnessing more structural information to achieve precise predictions of complex dynamics.
Addressing this challenge, a collaborative effort among applied mathematicians and AI scientists from institutions in China has yielded a solution. Published in Nature Communications, the study, involving Fudan University, Center for Applied Mathematics of Huanan, and Soochow University introduces the Higher-Order Granger Reservoir Computing (HoGRC).
HoGRC stands as a lightweight framework designed for higher-order structures inference and dynamics prediction grounded in Granger causality and reservoir computing principles. Notably, this framework adeptly discerns the system's underlying high-order interactions while integrating the inferred high-order structures into reservoir computing, thereby elevating dynamics prediction accuracy.
To validate the HoGRC framework's efficacy, extensive experiments spanning diverse systems were conducted, including the classic chaotic systems, the networked complex systems, and the UK power grid system.
The results unveiled significant advancements in both structure inference and dynamics prediction tasks, underscoring the potential of integrating structural information to bolster predictive capabilities and model robustness.
This pioneering work marks a pivotal step forward in the realm of lightweight machine learning models, promising enhanced accuracy in forecasting complex dynamics across various domains.
More information: Xin Li et al, Higher-order Granger reservoir computing: simultaneously achieving scalable complex structures inference and accurate dynamics prediction, Nature Communications (2024). DOI: 10.1038/s41467-024-46852-1