This article has been reviewed according to Science X's editorial process and policies. Editors have highlighted the following attributes while ensuring the content's credibility:
fact-checked
peer-reviewed publication
proofread
New framework may solve mode collapse in generative adversarial network
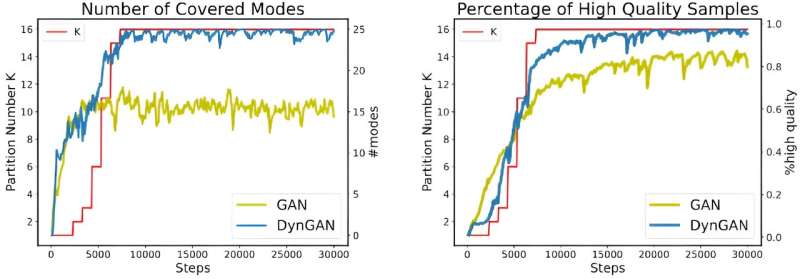
Generative adversarial networks (GANs) are widely used to synthesize intricate and realistic data by learning the distribution of authentic real samples. However, a significant challenge that GANs face is mode collapse, where the diversity of generated samples is notably lower than that of real samples. The complexity of GANs and their training process has made it difficult to reveal the underlying mechanism of mode collapse.
A research team led by Prof. Yang Zhouwang from the University of Science and Technology of China (USTC) of the Chinese Academy of Sciences (CAS) conducted a thorough investigation into the root cause of mode collapse and proposed a new framework, Dynamic GAN (DynGAN), to quantitatively detect and resolve mode collapse in GANs. Their work was published in IEEE Transactions on Pattern Analysis and Machine Intelligence.
Through theoretical analysis, the team found that the generator loss function is non-convex with respect to its parameters when multiple modes exist in the real data. Specifically, the parameters that result in the generated distribution covering only part of the real distribution's modes is the local minima of the generator loss function.
To tackle the issue of mode collapse, the team proposed a unified framework, DynGAN. This framework can establish thresholds on observable discriminator outputs to detect samples that the generator fails to generate, known as collapsed samples. The training sets are divided based on the collapsed samples, and then dynamic conditional generative models are trained on the partitions.
The theoretical results ensured the progressive mode coverage of DynGAN. Experimental results on both synthetic and real-world data sets showed that DynGAN surpasses existing GANs and their variants in resolving address mode collapse.
This research not only advanced the theoretical understanding of GAN, but also provides a crucial implementation strategy for improving the mode coverage of generative models.
More information: Yixin Luo et al, DynGAN: Solving Mode Collapse in GANs with Dynamic Clustering, IEEE Transactions on Pattern Analysis and Machine Intelligence (2024). DOI: 10.1109/TPAMI.2024.3367532