October 4, 2018 feature
A new developmental framework could allow robots to optimize hyper-parameters autonomously
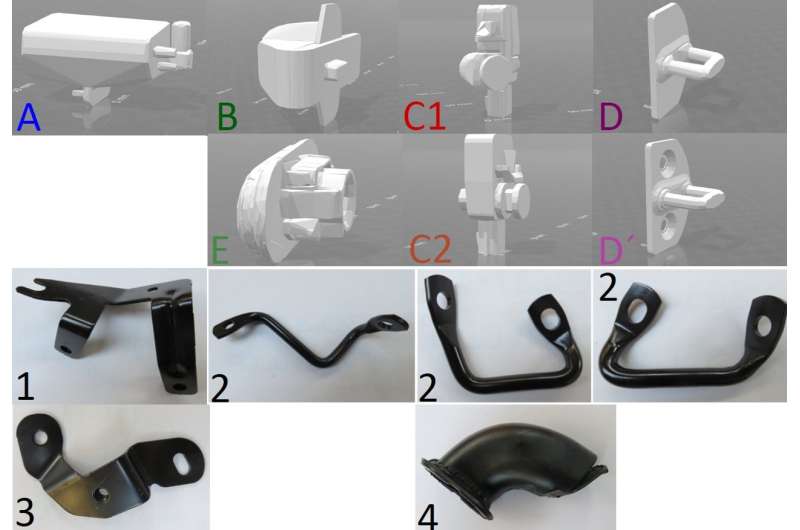
Researchers at Ecole Centrale de Lyon have recently devised a new developmental framework inspired by the long-term memory and reasoning mechanisms of humans. This framework, outlined in a paper presented at IEEE ICDL-Epirob in Tokyo and pre-published on arXiv, allows robots to autonomously optimize hyper-parameters tuned from any action and/or vision module, which are treated as a black box.
In recent years, researchers have built robots that can complete a variety of tasks. Nonetheless, the environment in which these robots operate is often somewhat constrained. This is because in robotics, most algorithms are crafted and optimized manually by human experts to anticipate the potential challenges that the robot might encounter within a given situation.
"However, when dealing with uncertainties, for instance, when the robot will have to deal with new objects frequently during its lifetime, always relying on additional programs and optimization from human engineers is not viable: the robot has to learn by itself," Dr. Maxime Petit, one of the researchers who carried out the study, told TechXplore. "We want to provide robots with learning mechanisms in order for them to be autonomous and adapt to various situations, even when these are not anticipated."
Developing robots that automatically adapt to different situations could have a number of useful applications, for instance, in the context of industrial robotics, where robotic arms need to pick up unknown objects from homogeneous bulks.
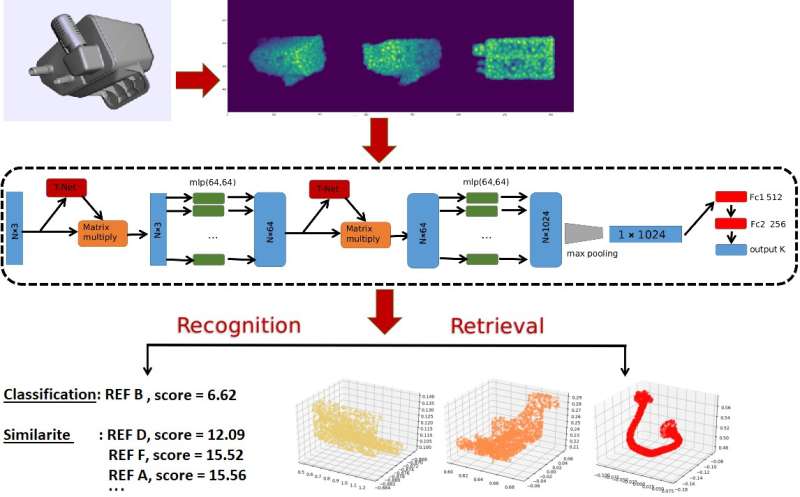
"We wanted the robot to autonomously optimize the parameters of a vision-based grasping software (Kamido by Siléane) for each object in less than a few hours," Petit explained. "To do so, we used a developmental framework that aims to replicate how children learn about the world. In particular, we want to take advantage of the similarity between objects to speed up the learning process by transferring knowledge of one task to another similar one."
The developmental framework presented by Petit and his colleagues is aimed at enabling lifelong and open-ended learning of new skills in robots through a process similar to that observed in human children. In order to achieve this, their framework includes a long-term memory in which robots store their experiences, as well as reasoning capabilities that allow them to identify useful elements in their stored data.
"The Bayesian optimization method works as a smart 'trial and error' strategy: The robot estimates what set of parameters it is best to evaluate based on the results of the previous evaluation," Petit explained. "In short, this method efficiently guides the robot in exploring parameters around values that were not tried, but also toward values that have high potential."
Despite the efficiency of this technique, the researchers wanted to increase the convergence of the robot's learning to achieve better optimization. Traditional Bayesian optimization methods were hence not desirable, as with these, the robot's learning starts from scratch for each new object it encounters. To address this limitation, the researchers employed a strategy based on vision similarity.
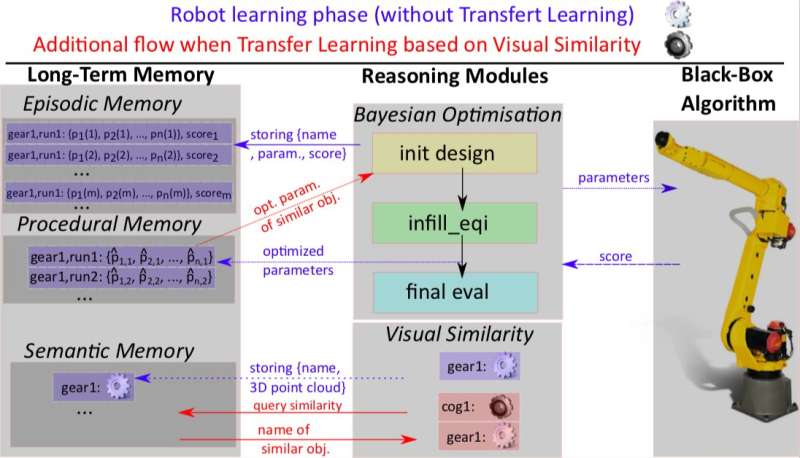
"We wanted to take advantage of the previous experience of the robot for different but similar tasks," Petit said. "Thus, when confronted with a new object, we force the robot to initially explore solutions that were optimized for similar objects. For example, if the robot knows how to grasp an orange and has now to grasp an apple, we force the robot to grasp the apple using the 'grasping orange' strategy. We are doing so by asking the visual similarity module what objects are similar to the new ones, and extracting the best solutions for these similar objects from the long-term memory."
The researchers evaluated their framework within a simulation environment. They found the transfer learning strategy based on visual similarity to be far more beneficial than amnesic learning, in which robots learn strategies from scratch every time they perform a new task.
So far, developmental frameworks have primarily been investigated within the context of social robotics, for instance, helping to personalize the assistance of social robots to the needs or preferences of their users.
"Using this framework in an industrial robotics setup led to convincing results, implying that the fields of social and industrial robotics have a lot to learn from each other," Petit said. "Another interesting aspect of our study is that the framework is optimizing a "black-box" algorithm, which means that we do not know anything about how it is working. We provide parameters to it, and in exchange, we receive a score for their quality. This means that it could also be applied to different apps and algorithms (even outside the robotics field) that need frequent fine tuning."
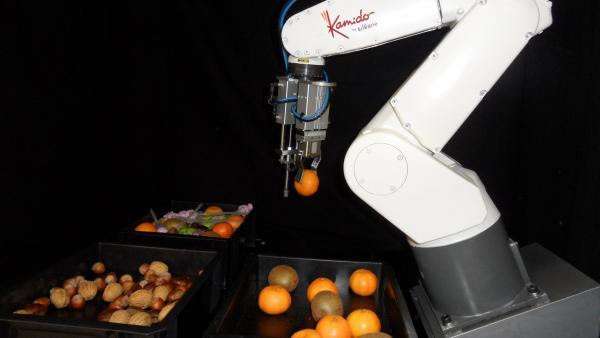
The researchers are now exploring ways in which robots could transfer knowledge about objects acquired during simulations to real tasks involving the same objects. This would further improve a robot's efficiency, allowing it to learn new things virtually and then apply them in real life.
"The optimization of parameters for objects sharing physical properties might also lead to the emergence of such concept for specific robots," Petit added. "For instance, a robot could learn that a 'flat,' 'heavy,' 'long,' etc. object has to be grasped with specific values for a subset of parameters. This can be used to enhance human-robot interactions and learning using natural language, for instance, with workers helping the robot by providing a small description of the new objects to be handled."
More information: Developmental Bayesian optimization of black-box with visual similarity-based transfer learning. Accepted and presented at IEEE ICDL-Epirob 2018, Tokyo, Japan. Pre-print version available at arxiv.org/abs/1809.10141
© 2018 Tech Xplore