New, more realistic simulator will improve self-driving vehicle safety before road testing
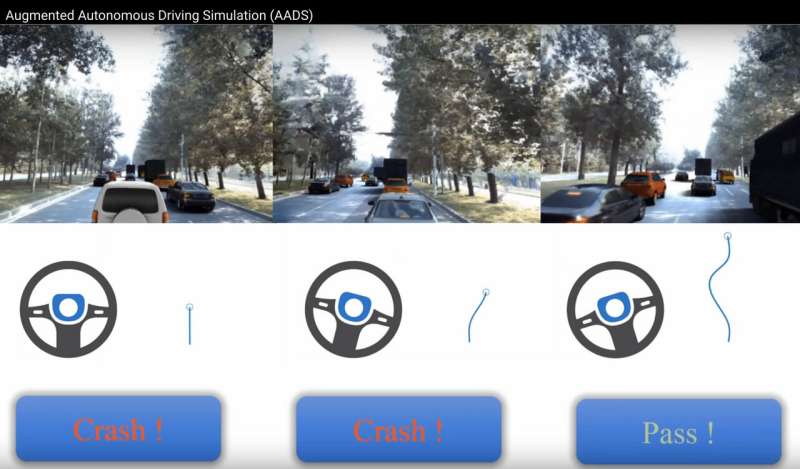
University of Maryland computer scientist Dinesh Manocha, in collaboration with a team of colleagues from Baidu Research and the University of Hong Kong, has developed a photo-realistic simulation system for training and validating self-driving vehicles. The new system provides a richer, more authentic simulation than current systems that use game engines or high-fidelity computer graphics and mathematically rendered traffic patterns.
Their system, called Augmented Autonomous Driving Simulation (AADS), could make self-driving technology easier to evaluate in the lab while also ensuring more reliable safety before expensive road testing begins.
The scientists described their methodology in a research paper published March 27, 2019 in the journal Science Robotics.
"This work represents a new simulation paradigm in which we can test the reliability and safety of automatic driving technology before we deploy it on real cars and test it on the highways or city roads," said Manocha, one of the paper's corresponding authors, and a professor with joint appointments in computer science, electrical and computer engineering, and the University of Maryland Institute for Advanced Computer Studies.
One potential benefit of self-driving cars is that they could be safer than human drivers who are prone to distraction, fatigue and emotional decisions that lead to mistakes. But to ensure safety, autonomous vehicles must evaluate and respond to the driving environment without fail. Given the innumerable situations that a car might encounter on the road, an autonomous driving system requires hundreds of millions of miles worth of test drives under challenging conditions to demonstrate reliability.
While that could take decades to accomplish on the road, preliminary evaluations could be conducted quickly, efficiently and more safely by computer simulations that accurately represent the real world and model the behavior of surrounding objects. Current state-of-the art simulation systems described in scientific literature fall short in portraying photo-realistic environments and presenting real-world traffic flow patterns or driver behaviors.
AADS is a data-driven system that more accurately represents the inputs a self-driving car would receive on the road. Self-driving cars rely on a perception module, which receives and interprets information about the real world, and a navigation module that makes decisions, such as where to steer or whether to break or accelerate, based on the perception module.
In the real world, the perception module of a self-driving car typically receives input from cameras and lidar sensors, which use pulses of light to measure distances of surrounding. In current simulator technology, the perception module receives input from computer-generated imagery and mathematically modeled movement patterns for pedestrians, bicycles, and other cars. It is a relatively crude representation of the real world. It is also expensive and time- consuming to create because computer-generated imagery models must be hand generated.
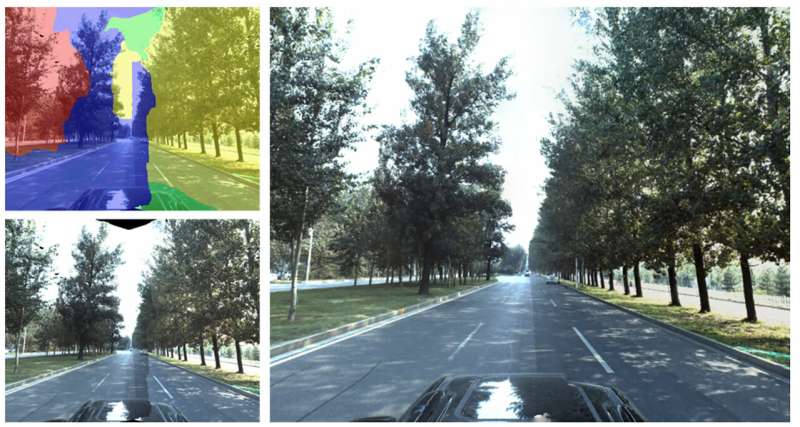
The AADS system combines photos, videos, and lidar point clouds—which are like 3-D shape renderings—with real-world trajectory data for pedestrians, bicycles, and other cars. These trajectories can be used to predict the driving behavior and future positions of other vehicles or pedestrians on the road for safer navigation.
"We are rendering and simulating the real world visually, using videos and photos," said Manocha, "but also we're capturing real behavior and patterns of movement. The way humans drive is not easy to capture by mathematical models and laws of physics. So, we extracted data about real trajectories from all the video we had available, and we modeled driving behaviors using social science methodologies. This data-driven approach has given us a much more realistic and beneficial traffic simulator."
The scientists had a long-standing challenge to overcome in using real video imagery and lidar data for their simulation: Every scene must respond to a self-driving car's movements, even though those movements may not have been captured by the original camera or lidar sensor. Whatever angle or viewpoint is not captured by a photo or video has to be rendered or simulated using prediction methods. This is why simulation technology has always relied so heavily on computer-generated graphics and physics-based prediction techniques.
-
The dataset produced by the AADS system, including RGB images (top), annotations of different objects (middle), and a point cloud (a set of data points in space). Credit: Li et al., Sci. Robot. 4, eaaw0863 (2019) -
The AADS method produced an image (bottom left corner) that is more visually similar to a real image from CityScapes (bottom right), a robust dataset of urban street scenes, than it is to CARLA (upper left), the most recent and popular VR simulator for autonomous driving, or the fully synthetic dataset VKITTI (upper right). Credit: Li et al., Sci. Robot. 4, eaaw0863 (2019)
To overcome this challenge, the researchers developed technology that isolates the various components of a real-world street scene and renders them as individual elements that can be resynthesized to create a multitude of photo-realistic driving scenarios.
With AADS, vehicles and pedestrians can be lifted from one environment and placed into another with the proper lighting and movement patterns. Roads can be recreated with different levels of traffic. Multiple viewing angles of every scene provide more realistic perspectives during lane changes and turns. In addition, advanced image processing technology enables smooth transitions and reduces distortion compared with other video simulation techniques. The image processing techniques are also used to extract trajectories, and thereby model driver behaviors.
"Because we're using real-world video and real-world movements, our perception module has more accurate information than previous methods," Manocha said. "And then, because of the realism of the simulator, we can better evaluate navigation strategies of an autonomous driving system."
Manocha said that by publishing this work, the scientists hope some of the corporations developing self-driving vehicles might incorporate the same data-driven approach to improve their own simulators for testing and evaluating autonomous driving systems.
More information: "AADS: Augmented Autonomous Driving Simulation using Data-driven Algorithms," Science Robotics (2019). robotics.sciencemag.org/lookup … /scirobotics.aaw0863