Meet Jaco and Baxter, machine learning robots who cook perfect hot dogs
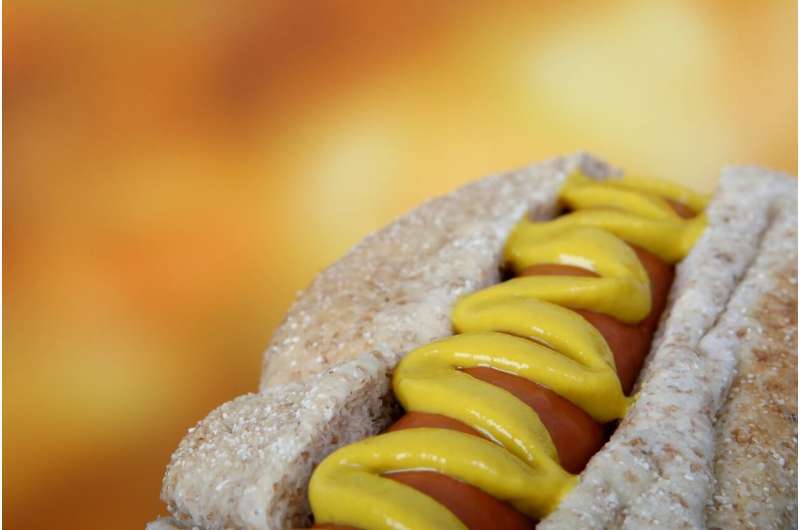
Craving a bite out of a freshly grilled ballpark frank? Two robots named Jaco and Baxter can serve one up. Boston University engineers have made a jump in using machine learning to teach robots to perform complex tasks, a framework that could be applied to a host of tasks, like identifying cancerous spots on mammograms or better understanding spoken commands to play music. But first, as a proof of concept—they've learned how to prepare the perfect hot dog.
Researchers still don't fully understand exactly how machine-learning algorithms—well, learn. That blind spot makes it difficult to apply the technique to complex, high-risk tasks such as autonomous driving, where safety is a concern. In a step forward published in Science Robotics, Calin Belta, a BU College of Engineering professor, and researchers in his lab taught two robots to cook, assemble, and serve hot dogs together. Their method combines techniques from machine learning and formal methods, an area of computer science that is typically used to guarantee safety, most notably used in avionics or cybersecurity software. These disparate techniques are difficult to combine mathematically and to put together into a language a robot will understand.
Belta, a professor of mechanical, systems, and electrical and computing engineering, and his team employed a branch of machine learning known as reinforcement learning. When a computer completes a task correctly, it receives a reward that guides its learning process. Although the steps of the task are outlined in a "prior knowledge" algorithm, how exactly to perform those steps isn't. When the robot gets better at performing a step, its reward increases, creating a feedback mechanism that pushes the robot to learning the best way to, for example, place a hot dog on a bun.
Integrating prior knowledge with reinforcement learning and formal methods is what makes this technique novel. By combining these three techniques, the team can cut down the amount of possibilities the robots have to run through to learn how to cook, assemble, and serve a hot dog safely. Belta sees this work as a proof-of-concept demonstration of their general framework, and he hopes that moving forward it can be applied to other complex tasks, such as autonomous driving.
More information: Xiao Li et al. A formal methods approach to interpretable reinforcement learning for robotic planning, Science Robotics (2019). DOI: 10.1126/scirobotics.aay6276