Q&A: How electric vehicles interact with the electric grid
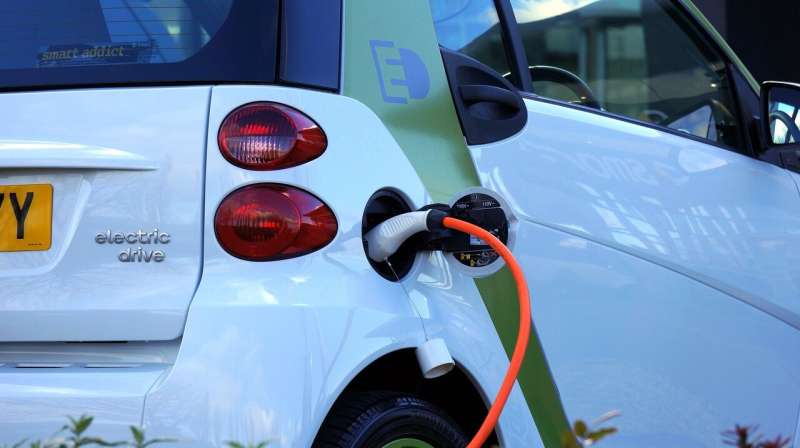
As the rate of electric vehicle (EV) adoption in the U.S. rises, the transportation sector will put additional pressure on the power grid. California expects more than 50% zero-emission vehicles (ZEVs), which includes battery EV, plug-in hybrid EV and fuel cell EV, in new vehicle sales by 2030 to achieve statewide emission and pollution reduction goals. This added pressure on the grid could be very disruptive, but transportation and power grid researchers at the Department of Energy's Lawrence Berkeley National Laboratory (Berkeley Lab) are looking for solutions to help city planners prepare.
Berkeley Lab Research Scientist Bin Wang knows that coming up with solutions is going to require an interdisciplinary approach, and in many ways Wang's background represents the perfect intersection of expertise required. He has experience working on electric grid, transportation and vehicle engineering research, all of which are key domains needed to solve for ZEV growth.
Researchers really only started looking at the integration of grid and transportation research in the last couple of years, due to the growth of EVs, grid modernization, ride-sharing and autonomous vehicles on the road. Wang is focused on developing scalable solutions to analyze EV impacts on the grid as well as maximize the benefits of EV adoption. Wang's vision is to develop these algorithms on a large metropolitan-scale model, e.g. the San Francisco Bay Area. The combination of doing this analysis on a large-scale—as well as optimizing for both the transportation and electric grid—makes this more complex than many other computational transportation projects.
In this Q&A, Wang shares his insights on the role EV adoption will play in the evolution of our electric grid, and he outlines the work Berkeley Lab is doing to help prepare our transportation and grid sectors for this major shift.
Q. How would you define grid/transportation integration and why is it important?
A. Our transportation system is rapidly modernizing, and the trend is towards transportation electrification, i.e. EVs. Grid/transportation integration refers to the innovative ways that EV electricity demand in the transportation sector interacts with electric grid planning and operations. Specifically, EVs will have the capability to be managed in terms of charging location, time, and power, as well as to support multiple grid services.
According to regulations in California, we expect 5 million electric vehicles in the state by 2030. I think it is a huge transition that current technology, including hardware, software, algorithms and even the consumers are not ready for. In the next few years, it is very likely that we will have more EVs than available public chargers so software can be useful to coordinate those charging behaviors among many drivers over a large utility service territory.
If we just drive our EV from home to work and work to home, and we charge our cars whenever we want to charge, this is not the best approach. With additional information, we can optimize or change our behavior a little bit. For example, I can do some programming to set the chargers to start charging after midnight so I can personally reduce my energy bill, and I can avoid congested roads so that mileage anxiety can be alleviated. We can make smarter decisions in terms of charging time, power location and route selection.
In the EV Integration domain, we are working to solve those barriers. Software solutions can be used as the bridge to connect the physical infrastructure with real-world EV drivers. It will enable more complex interactions between EV drivers and the charging infrastructures. For example, as an EV driver, I may need to know if the charging infrastructure will be occupied when I arrive. If I arrive at a certain time of the day, how much energy do I need to draw from the electric grid into my car battery, and how long can I be there? Maybe I will be subject to some surcharges from the utility depending on the time of day. Intelligent decision algorithms can help us solve for all of these variables.
The other side of this problem is with the electric utilities. What they may see in their system are unexpected load spikes caused by a huge number of EV charging activities. For example, in the San Francisco Bay Area, the EV charging load spikes around 7 or 8 pm and could be problematic.
Those unexpected behaviors need to be modeled for utilities to see how much damage there could be on their systems, what kind of equipment would be needed to upgrade the infrastructure and how much money they will need to invest in additional circuits, generators or protection devices for the system to prevent damage from the new EV charging load.
Much of our modeling work can also impact planning. For example, if I want to save energy for Alameda County and save travel time for travelers in the county, I can publish attractive pricing policies so people can shift their behavior a little bit so the overall impact on the system can be reduced. Or, if I have a $1 billion budget to spend in the next 10 years for upgrading the infrastructure, I can use a computational tool to let me know if I do it in this way, how much benefit I can have, how many customers or EV drivers I can serve, and how many dollars I can save in terms of the energy bill. Among all of these scenarios, I can tell you which one is the best. We can use the program to compute better solutions for urban planners and utilities. This is how we can do things intelligently.
Q. How have optimization techniques and modeling been adapted to benefit grid/transportation integration and what challenges still exist?
A. Previously, we already had a variety of existing modeling and optimization techniques for transportation, the electric grid and also for electric vehicles, but those approaches were separate and disconnected. Now these three systems are tightly coupled together and it turns out there is not a comprehensive optimization framework that captures all elements involved in this new problem.
Some of our biggest challenges to grid/transportation integration include modeling the complex interactions between the electric grid (planning and operation) and the electric vehicle behaviors (travel and charge) within the context of the entire transportation system. Solving the exact optimal solution is computationally hard, especially for the full scale problem. Super-fast solutions with novel algorithms are needed, leveraging high-performance computing technology. In order to improve the model fidelity and scalability for the metropolitan-scale transportation and electric grid systems, e.g. the San Francisco Bay Area, such computational techniques are essential to enable faster simulation, optimization and control with reduced solving time.
Q. What advances has your team at Berkeley Lab made toward increased grid/transportation integration?
A. We have completed preliminary work on large-scale transportation and electric grid modeling, computation and control leveraging high-performance computing techniques. Part of my work previously was to develop an optimal fleet charging optimization strategy that has been implemented in the real world, i.e. a demonstration site in the Alameda County with a number of fleet EVs. The software solution we developed was taking the information from the drivers, the vehicles as well as the demand response signals from the utilities to manage the charging time and load of the fleet vehicles, so that the aggregate load profile minimizes energy bills while ensuring that each individual EV's energy demand is satisfied.
We kick-started a new project with the California Energy Commission to develop a planning tool for electric medium- and heavy-duty vehicles (MHDV) projection, a.k.a HEVI-Pro. The goal of this project is to plan for the charging infrastructure needed to decarbonize MHDVs and reduce the impact of diesel air pollution in California. The new tool will enable policy-makers to perform optimal assessment of multiple charging infrastructure deployment scenarios for medium and heavy-duty electric vehicles across the entire state of California.
The research problem is statewide, but the granularity is down to each county. For example, the tool is able to determine how many/what types of fast chargers are needed in Alameda County or Los Angeles County by 2030. While cost is one consideration in our modeling, there are also other concerns like equity among EV drivers and also emissions goals by county. In the future, we hope to be able to provide an open-source solution to help city planners make the grid and transportation infrastructure more resilient. For example, city planners would be able to use this tool to determine the number of charging stations required and optimal locations for those charging stations throughout the SF Bay Area while ensuring the circuit capacity constraints are not violated by the additional charging load from electric MHDVs.
Q. Do you have any closing thoughts about the future of grid/transportation integration related to your current research?
A. Approximately 30% of energy consumption in the transportation sector will likely come from the electric grid in California by 2030, and therefore there will be more interdependencies between the transportation sector and electric grid sector. I think a brighter future of transportation/grid integration lies ahead with the integration of other cutting-edge technologies, such as Artificial Intelligence, autonomous driving and data analytics, etc. However, more work needs to be done to develop novel models with enough scalability, fidelity and computational breakthroughs to enable more effective decision-making over the coupled grid-transportation-EV systems.
Another area of research that may be interesting in the future is to take into account electric vehicles as moving energy storage. If the number of those electric vehicles is growing, then we may be able to aggregate this mobile energy storage as one virtual stationary battery or one virtual power plant to provide a variety of services for transportation and electric grid systems. It makes the system more flexible to operate multiple EV types, serving travel demand and providing real-time support to balance the electric grid. Ultimately, these intelligent decision-making applications will minimize the impact of increased EV usage on the power grid and improve overall societal benefits.