March 6, 2021 report
Facebook enhances AI computer vision with SEER
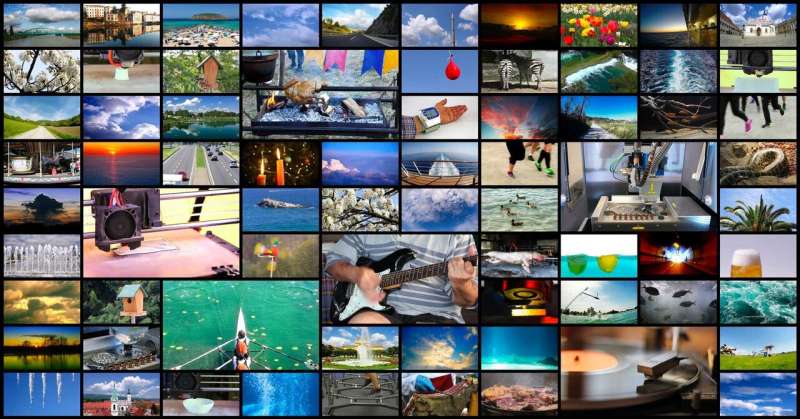
At a time when many versions of AI rely on pre-established data sets for image recognition, Facebook has developed SEER (Self-supERvised) – a deep learning solution able to register images on the Internet independent of curated and labeled data sets.
With major advances already underway in natural language processing (NLP) including machine translation, natural language interference and question answering, SEER uses an innovative billion-parameter, self-supervised computer vision model able to learn from any online image.
Thus far, the Facebook AI team has tested SEER on one billion uncurated and unlabeled public Instagram images. The new program performed better than the most advanced self-supervised systems as well as self-supervised models on downstream tasks such as low-shot, object detection, image detection and segmentation. In fact, exposure to only 10 percent of the ImageNet data set still resulted in a 77.9 percent recognition rate by SEER. Additionally, SEER obtained a 60.5 percent accuracy rate when trained on only 1 percent of the same data set.
Now that Facebook has witnessed SEER's ability to recognize Internet images in an applied setting, the AI team encourages developers and other interested parties in the machine learning field to share ideas for improvement and knowledge regarding SEER's capabilities. The company has opened this discussion via its open source library, VISSL, used to develop SEER.
Naturally, machine learning for language versus for visual recognition differs in that linguistics requires a program to recognize the semantic connection between a word and its corresponding definition. Computer vision, on the other hand, must identify how individual pixels group to form a completed image. Successful vision technology tackles such a challenge using two methods: 1) an algorithm that trains using a large number of random online images without annotations or metadata, and 2) a network large enough to capture and learn every visual component from the data set in question.
In order to mitigate challenges related to computing capacity for such large amounts of graphics, Facebook AI has developed the SwAV algorithm. This algorithm uses online clustering to quickly group images with similar visual concepts in order to identify similar visual data encountered later on. So far, SwAV has helped SEER perform with 6x less training time.
In addition to the use of SEER and VISSL to improve computer vision and machine learning, Facebook has implemented several existing algorithms that reduce the memory requirement per graphical programming unit, thus increasing the training speed of any model. These algorithms include mixed precision from NVIDIA Apex library, gradient checking from PyTorch, sharded optimizer from the FairScale library, and dedicated optimizations for online self-supervised training.
More information: Goyal, P., et al. "SEER: The Start of a More Powerful, Flexible, and Accessible Era for Computer Vision." Facebook AI, Facebook, 4 Mar. 2021, ai.facebook.com/blog/seer-the- … for-computer-vision/
© 2021 Science X Network