New method detects deepfake videos with up to 99% accuracy
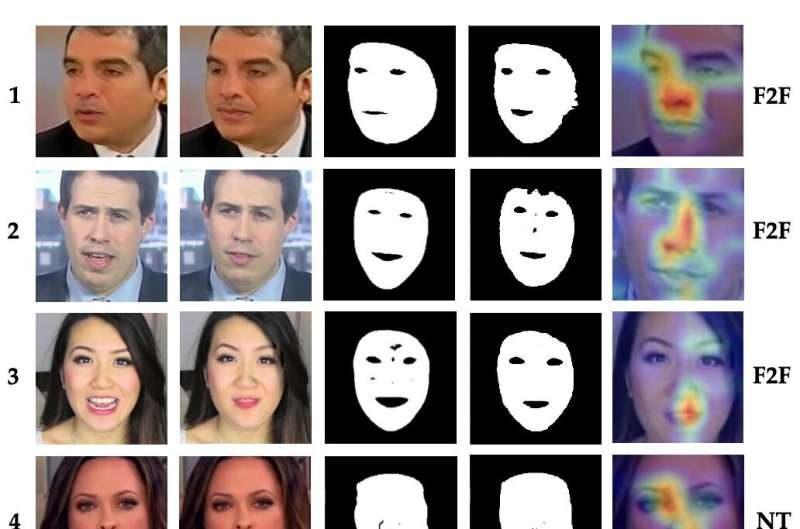
Computer scientists at UC Riverside can detect manipulated facial expressions in deepfake videos with higher accuracy than current state-of-the-art methods. The method also works as well as current methods in cases where the facial identity, but not the expression, has been swapped, leading to a generalized approach to detect any kind of facial manipulation. The achievement brings researchers a step closer to developing automated tools for detecting manipulated videos that contain propaganda or misinformation.
Developments in video editing software have made it easy to exchange the face of one person for another and alter the expressions on original faces. As unscrupulous leaders and individuals deploy manipulated videos to sway political or social opinions, the ability to identify these videos is considered by many essential to protecting free democracies. Methods exist that can detect with reasonable accuracy when faces have been swapped. But identifying faces where only the expressions have been changed is more difficult and to date, no reliable technique exists.
"What makes the deepfake research area more challenging is the competition between the creation and detection and prevention of deepfakes which will become increasingly fierce in the future. With more advances in generative models, deepfakes will be easier to synthesize and harder to distinguish from real," said paper co-author Amit Roy-Chowdhury, a Bourns College of Engineering professor of electrical and computer engineering.
The UC Riverside method divides the task into two components within a deep neural network. The first branch discerns facial expressions and feeds information about the regions that contain the expression, such as the mouth, eyes, or forehead, into a second branch, known as an encoder-decoder. The encoder-decoder architecture is responsible for manipulation detection and localization.
The framework, called Expression Manipulation Detection, or EMD, can both detect and localize the specific regions within an image that have been altered.
"Multi-task learning can leverage prominent features learned by facial expression recognition systems to benefit the training of conventional manipulation detection systems. Such an approach achieves impressive performance in facial expression manipulation detection," said doctoral student Ghazal Mazaheri, who led the research.
The benchmark datasets for facial manipulation are based on expression and identity swap. One transfers the expressions of a source video onto a target video without changing the identity of the person in the target video. The other swaps two identities in a single video.
Experiments on two challenging facial manipulation datasets show EMD has better performance in detection of not only facial expression manipulations but also identity swaps. EMD accurately detected 99% of the manipulated videos.
The paper is titled "Detection and Localization of Facial Expression Manipulations" and was presented at the 2022 Winter Conference on Applications of Computer Vision.
More information: Ghazal Mazaheri, Amit K. Roy-Chowdhury, Detection and Localization of Facial Expression Manipulations. arXiv:2103.08134v1 [cs.CV], arxiv.org/abs/2103.08134