Neural network to help ecologists, foresters and power line operators size up trees from above
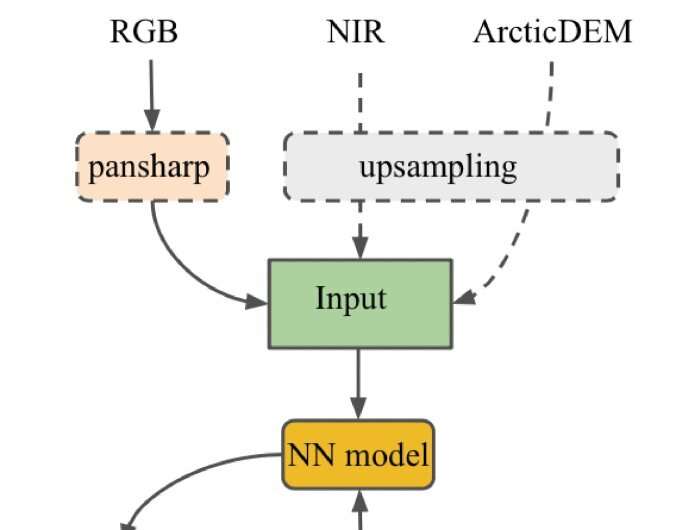
Skoltech researchers have trained a neural model to determine the height of trees on satellite imagery to monitor the natural environment, infrastructure, and timber supply. Unlike prior solutions, the model presented in IEEE Access does not require drone footage or imaging beyond the visible range, in multiple infrared bands—such data are much harder to come by.
Environmental scientists, civil engineers, and people from the wood industry need reliable estimates of forest canopy height based on optical satellite imagery. It tells them how well the ecosystem is doing, how much atmospheric carbon dioxide the trees are capturing, whether there's risk of trees damaging power lines, and how much timber is available for logging.
Observations on location by forest rangers or using drones are not feasible for large areas and regions that are hard to access. The alternative is satellite imaging simultaneously with multiple cameras that span several bands of infrared radiation in addition to visible light. These so-called multispectral data are more scarce and expensive, though.
In a recently published study, Skoltech researchers and their colleague from Sber present a neural network that delivers high-quality predictions of forest canopy height using ordinary, optical satellite imagery only.
"The one biggest factor that makes our neural network successful is its ability to analyze spatial data and texture characteristics. It exploits the connection that exists between tree crown shape and height," says the first author of the study, Skoltech Ph.D. student Svetlana Illarionova.
"Our neural network draws on additional input data," she continues. "Along with the optical imagery, we put in supplementary features in the form of ArcticDEM, a freely available high-resolution model. It is a 2-meter-resolved representation of the bare topographic surface of the Earth covering boreal regions."
The training data for the model come from the northern Russian region of Arkhangelsk. Canopy height predictions are scored based on how well they match lidar observations made on location in that region with drones. That said, the researchers say their solution is applicable to wherever more or less similar vegetation grows.
More information: Svetlana Illarionova et al, Estimation of the Canopy Height Model From Multispectral Satellite Imagery With Convolutional Neural Networks, IEEE Access (2022). DOI: 10.1109/ACCESS.2022.3161568