November 25, 2022 feature
A model that can recognize speech in different languages from a speaker's lip movements
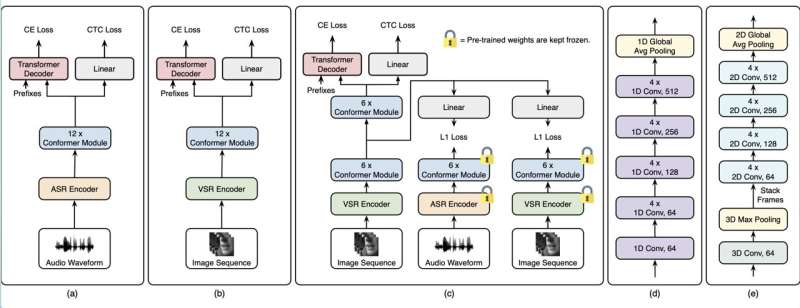
In recent years, deep learning techniques have achieved remarkable results in numerous language and image-processing tasks. This includes visual speech recognition (VSR), which entails identifying the content of speech solely by analyzing a speaker's lip movements.
While some deep learning algorithms have achieved highly promising results on VSR tasks, they were primarily trained to detect speech in English, as most existing training datasets only include English speech. This limits their potential user base to people who live or work in English-speaking contexts.
Researchers at Imperial College London have recently developed a new model that can tackle VSR tasks in multiple languages. This model, introduced in a paper published in Nature Machine Intelligence, was found to outperform some previously proposed models trained on far larger datasets.
"Visual speech recognition (VSR) was one of the main topics of my Ph.D. thesis," Pingchuan Ma, a Ph.D. graduate from Imperial College who carried out the study, told TechXplore. "During my studies, I worked on several topics, for instance exploring how to combine visual information with audio for audio-visual speech recognition and how to recognize visual speech independently of the head pose of participants. I realized that the vast majority of existing literature only dealt with English speech."
The key objective of the recent study by Ma and his colleagues was to train a deep learning model to recognize speech in languages other than English from the lip movements of speakers and then compare its performance to that of other models trained to recognize English speech. The model created by the researchers is similar to those introduced by other teams in the past, but but some of its hyper-parameters were optimized, the dataset was augmented (i.e., increased in size by adding synthetic, slightly modified versions of data) and additional loss functions were used.
"We showed that we can use the same models to train VSR models in other languages," Ma explained. "Our model takes raw images as input, without extracting any features, and then automatically learns what useful features to extract from these images to complete VSR tasks. The main novelty of this work is that we train a model to perform VSR and also add some additional data augmentation methods and loss functions."
In initial evaluations, the model created by Ma and his colleagues performed remarkably well, outperforming other VSR models trained on much larger datasets, even if it required less original training data. As expected, however, it did not perform as well as English-speech recognition models, mainly due to smaller datasets available for training.
"We achieved state-of-the-art results in multiple languages by carefully designing the model, rather than by simply using larger datasets or larger models, which is the current trend in the literature," Ma said. "In other words, we showed that how a model is designed is equally important to its performance than increasing its size or using more training data. This can potentially lead to a shift in the way researchers try to improve VSR models."
Ma and his colleagues showed that one can achieve state-of-the-art performances in VSR tasks by carefully designing deep learning models, instead of using larger versions of the same model or collecting additional training data, which is both expensive and time consuming. In the future, their work could inspire other research teams to develop alternative VSR models that can effectively recognize speech from lip movements in languages other than English.
"One of the main research areas I'm interested in is how we can combine VSR models with existing (audio-only) speech recognition," Ma added. "I'm particularly interested in how these models can be dynamically weighted, i.e., how the model can learn which model should rely on depending on the noise. In other words, in a noisy environment an audio-visual model should rely more on the visual stream, but when the mouth region is occluded it should rely more on the audio stream. Existing models are essentially frozen once trained and they cannot adapt to changes in the environment."
More information: Pingchuan Ma et al, Visual speech recognition for multiple languages in the wild, Nature Machine Intelligence (2022). DOI: 10.1038/s42256-022-00550-z
© 2022 Science X Network