This article has been reviewed according to Science X's editorial process and policies. Editors have highlighted the following attributes while ensuring the content's credibility:
fact-checked
peer-reviewed publication
trusted source
proofread
Artificial intelligence reframes nuclear material studies
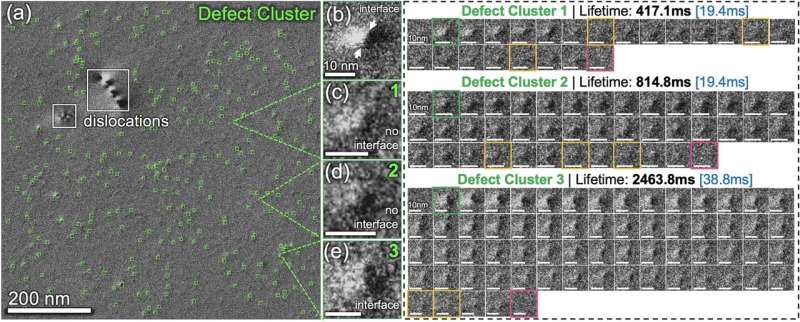
The future of nuclear energy, which can produce electricity without harmful emissions, depends on discovery of new materials. A scientist at Argonne is using computer vision to separate the best candidates from a crowded field.
If a picture can tell a thousand words, imagine the frame-by-frame story that can be gleaned from a single video. Five minutes of video containing 200 frames per second can result in 60,000 images—a visual "Moby Dick." Sound tedious to digest and catalog? It is, which explains why scientists don't usually analyze their experiments' videos in such detail.
Wei-Ying Chen, a principal materials scientist in the nuclear materials group at the Department of Energy's (DOE) Argonne National Laboratory, is experimenting with advances in artificial intelligence (AI) to change that. The deep learning-based multi-object tracking (MOT) algorithm he uses to extract data from videos, as detailed in a study recently published in Scientific Reports, aims to help the U.S. improve advanced nuclear reactor designs. In turn, modernized nuclear power would better produce safe, reliable electricity without releasing harmful greenhouse gases.
Currently, nuclear energy produces more electricity on less land than any other clean energy source. Many commercial nuclear reactors, which supply nearly 20% of total U.S. electricity, use older materials and technology. Scientists and engineers believe newer materials and advanced designs could substantially increase the percentage of clean electricity generated by nuclear power plants.
"We want to build advanced reactors that can run at higher temperatures, so we need to discover materials that are resistant to higher temperature and higher irradiation dose," said Chen. "With computer vision tools, we are on track to get all the data we need from all of the video frames."
Chen assists users and conducts experiments at Argonne's Intermediate Voltage Electron Microscope (IVEM) facility, a national user facility and a partner facility of DOE's Nuclear Science User Facilities (NSUF). The IVEM—part transmission electron microscope, part ion beam accelerator—is one of about a dozen instruments in the world that let researchers look at material changes caused by ion irradiation as the changes happen (in situ). This means scientists like Chen can study the effects of different energies on materials proposed for use in future nuclear reactors.
Understanding why, where and when materials break down and show defects under extreme conditions over the course of their lifetimes is critical in order to judge a material's suitability for use in a nuclear reactor. Extremely tiny defects are the first signs that a material will corrode, become brittle or fail. During experiments, defects happen within a picosecond, or one-trillionth of a second. At high temperatures, these defects appear and disappear in tens of milliseconds. Chen is an expert in IVEM experiments and said even he struggles to plot and interpret such fast-moving data.
The fleeting nature of defects during experiments explains why scientists traditionally captured only a smattering of data points along important lines of measure.
Chen has spent the past two years developing computer vision to track material changes from recorded experiments at IVEM. In one project, he examined 100 frames per second from videos one to two minutes long. In another, he extracted one frame per second in videos one to two hours long.
Similar to facial recognition software that can recognize and track people in surveillance footage, the computer vision at IVEM singles out material defects and structural voids. Instead of establishing a library of faces, Chen builds a vast, reliable collection of information about temperature resistance, irradiation resilience, microstructural defects and material lifetimes. This information can be plotted to inform better models and plan better experiments.
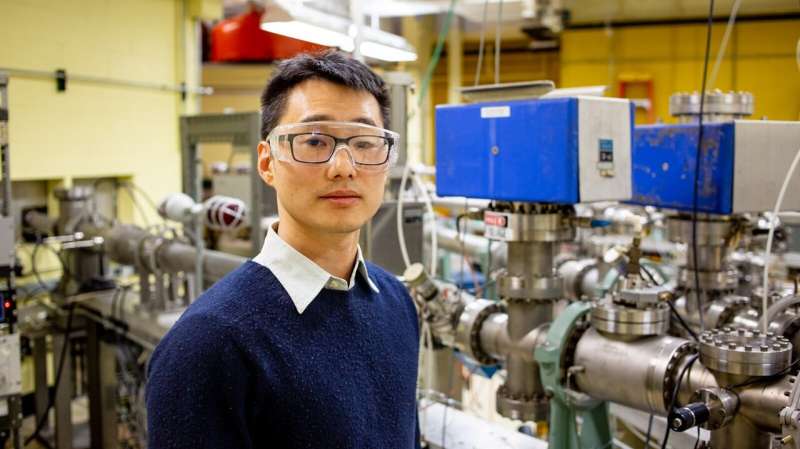
Chen stresses that saving time—a frequently cited benefit of computer-enabled work—isn't the exclusive benefit of using AI and computer vision at IVEM. With a greater ability to understand and steer experiments that are underway, IVEM users can make on-the-spot adjustments to use their time at IVEM more efficiently and capture important information.
"Videos look very nice, and we can learn a lot from them, but too often they get shown one time at a conference and then are not used again," said Chen. "With computer vision, we can actually learn a lot more about observed phenomena and we can convert video of phenomena into more useful data."
DefectTrack proves itself accurate and reliable
In research published in Scientific Reports, Chen and co-authors from the University of Connecticut (UConn) presented DefectTrack, a MOT capable of extracting complicated defect data in real time as materials were irradiated.
In the study, DefectTrack tracked up to 4,378 different defect clusters in just one minute, with lifetimes ranging from 19.4 to 64 milliseconds. The findings were starkly superior to the same work by human counterparts.
"Our statistical evaluations showed that the DefectTrack is more accurate and faster than human experts in analyzing the defect lifetime distribution," said UConn co-author and Ph.D. candidate Rajat Sainju.
Computer vision has multiple advantages; improved speed and accuracy are among them.
"We urgently need to speed up our understanding of nuclear materials degradation," said Yuanyuan Zhu, the UConn assistant professor of materials science and engineer who led the university's team of co-authors. "Dedicated computer vision models have the potential to revolutionize analysis and help us better understand the nature of nuclear radiation effects."
Chen is optimistic that computer vision such as DefectTrack will improve nuclear reactor designs.
"Computer vision can provide information that, from a practical standpoint, was unavailable before," said Chen. "It's exciting that we now have access to so much more raw data of unprecedented statistical significance and consistency."
More information: Rajat Sainju et al, DefectTrack: a deep learning-based multi-object tracking algorithm for quantitative defect analysis of in-situ TEM videos in real-time, Scientific Reports (2022). DOI: 10.1038/s41598-022-19697-1