This article has been reviewed according to Science X's editorial process and policies. Editors have highlighted the following attributes while ensuring the content's credibility:
fact-checked
proofread
Audio classification with skyrmion reservoirs
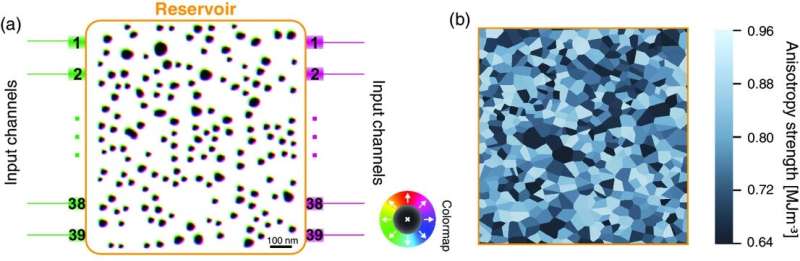
"Seven, one, nine, ...": A human voice pronounces digits, a physical material recognizes them with about 97 percent accuracy. This pattern recognition system was developed by physicists at the University of Duisburg-Essen (UDE) in collaboration with Ghent University (Belgium). The development enables multidimensional problems to be solved quickly and without energy-consuming training. The journal Advanced Intelligent Systems published the results.
Is it possible for an inanimate material to recognize patterns quickly and efficiently? That was the question asked by a team of theoretical physicists, led by Professor Dr. Karin Everschor-Sitte. To prove their point, the researchers used speech recognition.
The team, including Robin Msiska as first author, used audio recordings of the spoken digits ranging from 0 to 9 from a standard database. The physicists analyzed the type and intensity of the frequencies involved for each moment of the spoken word. Subsequently, they converted this information into voltage signals, that they applied to a thin magnetic film via 39 contacts.
This thin film material contains small magnetic whirls (skyrmions) that react to the voltage by deforming. "To put it simply, you can imagine it as a black-and-white grid pattern that changes shape in response to input signals," Msiska explains. The material thus forms unique patterns for each spoken number—like a QR code—which can be read out linearly with simple methods.
The team's research utilized complex simulations, primarily carried out at the Flemish Supercomputer Center (Vlaams Supercomputer Centrum) in collaboration with Ghent University. Their material system correctly recognized 97.4 percent of the numbers. Examining only female voices, the figure rose to 98.5 percent. "This shows the best performance ever reported for in-material reservoir computers," Everschor-Sitte reports. And it does so in the smallest of spaces; the sample the physicists worked with is just one micrometer in edge length.
"If one uses a neural network, training is expensive and requires enormous data sets. Our material system can solve machine learning problems without building a system of millions of interconnected neurons—the speech recognition shown here is just one example. It's faster and uses less energy," the physicist explains.
In her opinion, potential applications can be found where various signals need to be detected and interpreted: in autonomous driving, in weather forecasting, or in medical settings. In collaboration with other researchers at the UDE, their focus is currently on a standard medical examination: the electroencephalogram (EEG), which measures the electrical activity of the brain. The physicists are investigating whether the magnetic system can interpret these results independently.
More information: Robin Msiska et al, Audio Classification with Skyrmion Reservoirs, Advanced Intelligent Systems (2023). DOI: 10.1002/aisy.202200388