This article has been reviewed according to Science X's editorial process and policies. Editors have highlighted the following attributes while ensuring the content's credibility:
fact-checked
proofread
Simplified optical neural network component saves space and energy
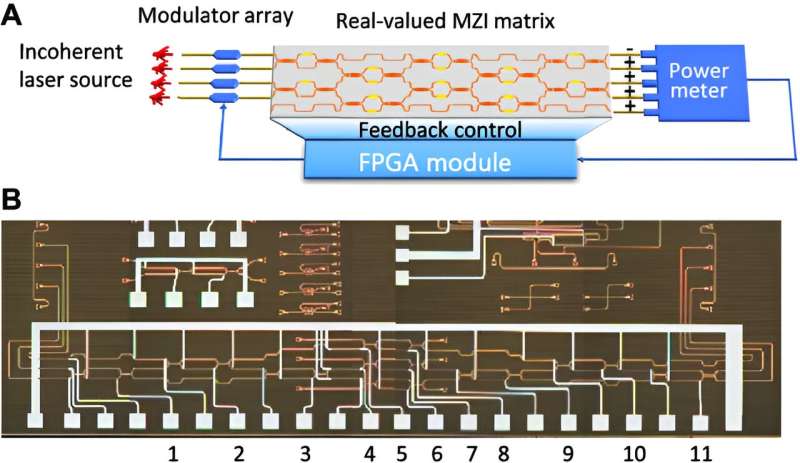
As research into photonic computing progresses, scientists seek to optimize the performance of optical computing devices by making purpose-specific changes to their design. A team led by Bo Wu and Shaojie Liu at Huazhong University of Science and Technology in China designed and tested a kind of Mach-Zehnder interferometer mesh that is more efficient at performing real-valued matrix-vector multiplication.
Their research was published Sep. 19 in Intelligent Computing.
Conventional Mach-Zehnder interferometer mesh has many advantages, including large bandwidth and high stability. However, it was designed for complex-valued matrix-vector multiplication, a type of computation that has hardware requirements different from those of real-valued matrix-vector multiplication. The new mesh is customized to take advantage of differences between the two types of computation.
The new mesh is more efficient because real-valued matrices have half as many degrees of freedom as complex-valued matrices, and thus require half as many phase shifters. Fewer phase shifters means a smaller piece of hardware that requires less electricity to operate. Moreover, the simplified mesh is easier to use and easier to fabricate because of the way it detects light.
The conventional mesh requires "coherent" light, such as light from a laser, whose waves are all the same size, shape, and direction. The simplified mesh detects "incoherent" light, a kind of light whose waves are much less regular and can be produced by a wider variety of light sources.
According to the researchers, "The results show that the proposed MZI mesh exhibits excellent performance in the benchmark task. Additionally, the error analysis indicates that the proposed scheme is robust to fabrication errors." Moreover, the simplified mesh is scalable, which means it holds great potential for use in large-scale optical neural networks, where the savings would be similarly large.
"We first designed the architecture with our intuition and common sense because there is a clear redundancy of phase shifters," said corresponding author Hailong Zhou of Huazhong University of Science and Technology.
The mesh was developed in several steps, beginning with a "type 0" conventional mesh for a complex-valued optical matrix, progressing to a "type 1" mesh with fewer phase shifters, then to a "type 2" mesh with an additional output port, then to a "type 3" mesh with a smaller footprint. The effectiveness of the type 3 mesh is supported by mathematical proofs and numerical simulation. "We just wanted to take a chance by doing the numerical simulation but surprisingly got an excellent result."
The simulation was the popular iris classification benchmark task, in which a computer must "learn" the properties of three different types of iris flower by processing a dataset of physical measurements corresponding to examples of each type. The training algorithm used in this research was particle swarm optimization. The next step, the authors said, is to perform a large-scale image classification task.
Optical neural networks are hardware devices that serve the same function as artificial neural networks that are built using conventional computers. That is, they can be programmed to perform machine learning tasks such as classification. According to the researchers, optical neural networks are similar to biological neural networks in that they both "handle input information with various linear and nonlinear physical operations."
The search for alternative computing paradigms such as photonic and quantum computing is motivated by the rapid increase in the computing power needed for artificial intelligence projects. The authors want to help "unleash the advantages of optical computing to the maximum."
They hope the technology can "truly get rid of the electronic components," so that there is no need for hybrid systems that must convert optical signals to electric signals and vice versa. They expect that powerful chips with "thousands of optical components" and "negligible power consumption" will someday "play an important role in our intelligent life" by underpinning computationally complex tasks such as autonomous driving.
More information: Bo Wu et al, Real-Valued Optical Matrix Computing with Simplified MZI Mesh, Intelligent Computing (2023). DOI: 10.34133/icomputing.0047