This article has been reviewed according to Science X's editorial process and policies. Editors have highlighted the following attributes while ensuring the content's credibility:
fact-checked
trusted source
proofread
New algorithms for intelligent and efficient robot navigation among the crowd
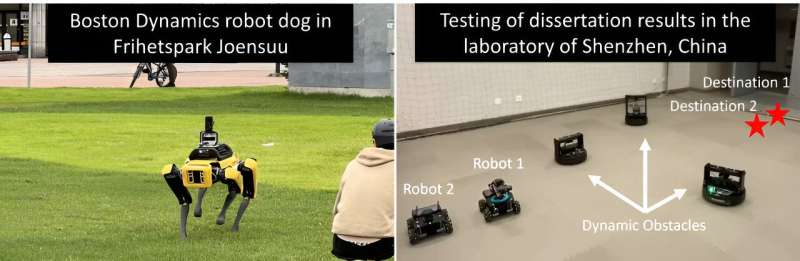
Service robots have started to appear in various daily tasks such as parcel delivery, as guide dogs for the visually impaired, as public servants at airports, or as seen in Joensuu: in the inspection of construction works. Robots are able to move in different ways: on legs, on wheels or by flying. They know the shortest or easiest route to the destination. A guide dog can search for bus schedules or even order a taxi when needed.
However, robots have difficulty coping with one basic thing: moving in the middle of a crowd of people. A robot observes the environment with a camera and other sensors, but its movement is jerky with continuous changes of direction, including several stops. Thus, robots are usually not even allowed to travel alone.
The problem with the latest robots is not in finding the destination or observing the surrounding world, but in the real-time reactions in the crowd. Current methods require too many computing resources and are therefore not suitable for real-time application where reactions should be quick.
In their dissertation, Chengmin Zhou, MSc, used reinforcement learning algorithms (RL) for the navigation of service robots. Algorithms solve navigation tasks in the case of several moving obstacles—that is, for example, in a situation where the robot moves in a crowd of people and has limited time to react.
The best solution turned out to be a model-free RL algorithm, which enables robots to learn from their historical experiences. After training or learning, robots are able to survive even in challenging situations. However, the model-free RL algorithm has many challenges, such as slow learning efficiency (convergence). In this dissertation, learning efficiency has been improved in two different ways:
- Utilization of data collected during operation for robot training. When operating robots, new real-time data is obtained. This data can be combined with previous training data, thus enhancing the robot's training.
- Translating environmental information. The sensor information collected from the robot's operating environment cannot be learned efficiently and accurately. It should be interpreted or translated so that the robot can learn it easily and the learned knowledge (trained model) can be used for navigation in other similar situations.
Robotic navigation is improved from three technical aspects: discrete actions (giving robots limited action choice to choose the next action), mixing real-time data and historical data, and exploiting relational data (utilizing the relationship of the robot and obstacles to train the robots). The developed algorithms have been tested both with computer simulations and in a laboratory environment at the Shenzhen Technology University, China.
The doctoral dissertation of Chengmin Zhou, MSc, titled "Deep Reinforcement Learning for Crowd-Aware Robotic Navigation," will be examined at the Faculty of Science, Forestry and Technology, Joensuu Science Park, 19 October 2023. The opponent will be Professor Juha Röning, University of Oulu, and the custos will be Professor Pasi Fränti, University of Eastern Finland. The language of the public defense is English.