This article has been reviewed according to Science X's editorial process and policies. Editors have highlighted the following attributes while ensuring the content's credibility:
fact-checked
peer-reviewed publication
trusted source
proofread
Researchers speed up design of next-gen biomedical implants, aerospace materials
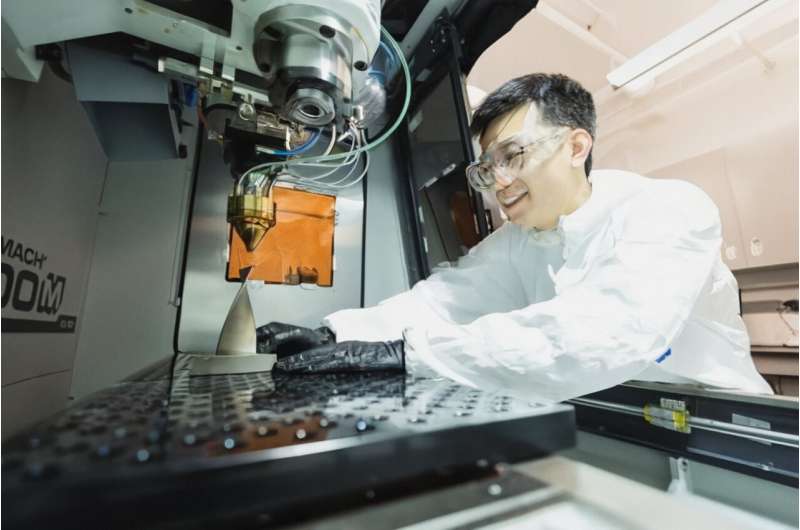
From bone-like medical implants to stronger, more fuel-efficient aircraft parts, advanced structural materials can take a long time to move from laboratories to industrial applications—but researchers at the University of Toronto are harnessing the power of machine learning to fast-track that process.
"Designing microstructures is a key step in materials development," says Yu Zou, an assistant professor in the department of mechanical and industrial engineering in the Faculty of Applied Science & Engineering. "But traditional materials design, which is based on experiments or simulation methods, could take years—even decades—to identify the right microstructure."
Zou and his lab group aim to accelerate the discovery of new structural materials. In a new paper, published in Materials Today, Zou's team describes a novel end-to-end framework used to tailor the microstructure of Ti-6Al-4V, the most widely used titanium alloy in the aerospace and biomedical industries.
"This work could enable materials scientists and engineers to discover microstructures at speeds unseen before, by simply inputting their desired mechanical properties into the framework," says Ph.D. candidate Xiao Shang, the lead author of the paper.
The researchers began by training two deep-learning models to accurately predict material properties from their microstructures. They then integrated a genetic algorithm with the deep-learning models to close the materials-by-design loop, which allows the framework to design optimal material microstructures with target mechanical properties.
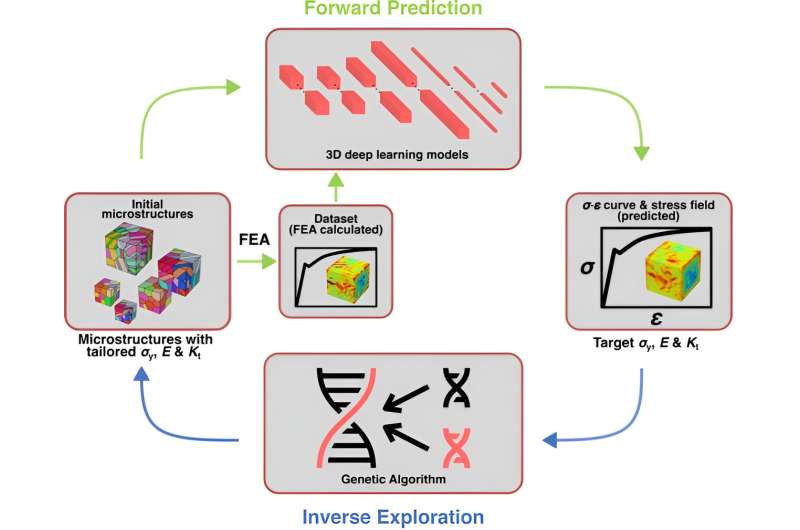
"In less than eight hours, we identified titanium alloy microstructures that showed both the high strength and high stiffness needed to strengthen the structural components of airplanes," says Shang. "We also designed titanium alloys with the same chemical compositions as the former but with different microstructures that are about 15 percent more compliant for biomedical implants compatible with human bones."
The researchers had to overcome several hurdles to develop their deep learning models. That includes generating their own dataset of close to 6,000 different microstructures through simulation—a task made possible by tapping the massive computing powers of super-computers at the Digital Research Alliance of Canada.
"We constantly ran into situations where our selected deep-learning models and/or optimization algorithms just wouldn't work as well as we expected," says Shang. "But we were patient and held on to our research plan while actively searching for new approaches to make the models work."
"Looking forward, we want to further optimize and improve additive manufacturing technology so that they can continue to advance this new framework," says Ph.D. candidate Tianyi Lyu, who co-authored the paper along with Ph.D. candidate Jiahui Zhang—both working on metal additive manufacturing.
"We are advancing the quality and reliability of metal additive manufacturing, unleashing its potentials to locally tailor the material microstructure during printing," adds Zou, who is a member of the Acceleration Consortium.
"For example, with traditional technology, it is close to impossible to tailor biomedical materials for different patients. But we want to enable the future of personalized biomedical implants by making it possible to print the shape and mechanical properties that match a patient's needs in only a few days."
More information: Xiao Shang et al, Tailoring the mechanical properties of 3D microstructures: A deep learning and genetic algorithm inverse optimization framework, Materials Today (2023). DOI: 10.1016/j.mattod.2023.09.007