This article has been reviewed according to Science X's editorial process and policies. Editors have highlighted the following attributes while ensuring the content's credibility:
fact-checked
trusted source
proofread
Researchers implement multi-focus image fusion using diffusion models
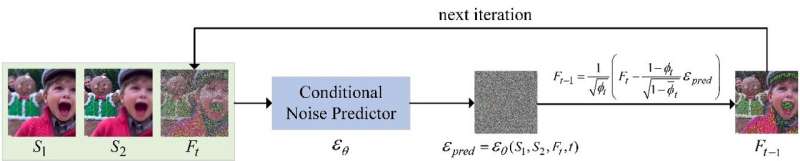
Multi-focus image fusion (MFIF) is an image enhancement technology that helps to solve the depth-of-field problem and capture all-in-focus images. It has broad application prospects that can effectively extend the depth of field of optical lenses.
Deep learning MFIF methods have shown advantages over traditional algorithms in recent years. However, more attention has been paid to increasingly large and complex network structures, gain modules, and loss functions to improve the fusion performance of the algorithms.
A team of researchers led by Fu Weiwei at the Suzhou Institute of Biomedical Engineering and Technology (SIBET) of the Chinese Academy of Sciences (CAS) has rethought the image fusion task and modeled it as a conditional generation model.
The researchers proposed an MFIF algorithm based on the diffusion model, called FusionDiff, by combining the current diffusion model with the best effect in the field of image generation. Their results were published in Expert Systems with Applications.
This is the first application of this diffusion model in the field of MFIF, which, according to the researchers, provides a new way of thinking for the research in this field.
Experiments show that FusionDiff outperforms traditional MFIF algorithms in terms of image fusion effect and few-shot learning performance.
"In addition, FusionDiff is a few-shot learning model, which means that it does not require much effort to generate large datasets," said Fu.
The fusion results achieved by FusionDiff are independent of a large amount of training data, according to Fu. "It realizes the transformation from data-driven to model-driven," he said.
Their study shows that FusionDiff achieves the same quality of fusion results as the other algorithms with only 2% of the training data they use. This significantly reduces the dependence of the fusion model on the dataset, said Fu.
More information: Mining Li et al, FusionDiff: Multi-focus image fusion using denoising diffusion probabilistic models, Expert Systems with Applications (2023). DOI: 10.1016/j.eswa.2023.121664